guide:090050c501: Difference between revisions
No edit summary |
mNo edit summary |
||
Line 1: | Line 1: | ||
<div class="d-none"> | <div class="d-none"> | ||
<math> | <math> | ||
\require{textmacros} | |||
\newcommand{\DS}{\displaystyle} | \newcommand{\DS}{\displaystyle} | ||
\newcommand{\intbeta}{\lfloor \beta \rfloor} | \newcommand{\intbeta}{\lfloor \beta \rfloor} | ||
Line 184: | Line 186: | ||
\newcommand{\thetalsK}{\hat \theta^{ls}_K} | \newcommand{\thetalsK}{\hat \theta^{ls}_K} | ||
\newcommand{\muls}{\hat \mu^{ls}} | \newcommand{\muls}{\hat \mu^{ls}} | ||
\newcommand{\thetahard}{\hat \theta^{\textsc{hrd}}} | \newcommand{\thetahard}{\hat \theta^{\textsc{hrd}}} | ||
\newcommand{\thetasoft}{\hat \theta^{\textsc{sft}}} | \newcommand{\thetasoft}{\hat \theta^{\textsc{sft}}} | ||
Line 198: | Line 196: | ||
\newcommand{\thetalsK}{\hat \theta^{\textsc{ls}}_K} | \newcommand{\thetalsK}{\hat \theta^{\textsc{ls}}_K} | ||
\newcommand{\muls}{\hat \mu^{\textsc{ls}}} | \newcommand{\muls}{\hat \mu^{\textsc{ls}}} | ||
</math> | |||
</div> | |||
In this chapter, we consider the following regression model: | In this chapter, we consider the following regression model: | ||
Line 211: | Line 213: | ||
Depending on the nature of the ''design'' points <math>X_1, \ldots, X_n</math>, we will favor a different measure of risk. In particular, we will focus either on ''fixed'' or ''random'' design. | Depending on the nature of the ''design'' points <math>X_1, \ldots, X_n</math>, we will favor a different measure of risk. In particular, we will focus either on ''fixed'' or ''random'' design. | ||
===Random design=== | ===Random design=== | ||
The case of random design corresponds to the statistical learning setup. Let <math>(X_1,Y_1), \ldots, (X_{n+1}, Y_{n+1})</math> be <math>n+1</math> | The case of random design corresponds to the statistical learning setup. Let <math>(X_1,Y_1), \ldots, (X_{n+1}, Y_{n+1})</math> be <math>n+1</math> i.i.d random couples. Given the pairs <math>(X_1,Y_1), \ldots, (X_{n}, Y_{n})</math>, the goal is construct a function <math>\hat f_n</math> such that <math>\hat f_n(X_{n+1})</math> is a good predictor of <math>Y_{n+1}</math>. Note that when <math>\hat f_n</math> is constructed, <math>X_{n+1}</math> is still unknown and we have to account for what value it is likely to take. | ||
Consider the following example from | Consider the following example from<ref name="HasTibFri01">{{cite journal|last1=Wright|first1=John|last2=Yang|first2=Allen Y.|last3=Ganesh|first3=Arvind|last4=Sastry|first4=S. Shankar|last5=Ma|first5=Yi|journal=IEEE Trans. Pattern Anal. Mach. Intell.|year=2009|title=Robust Face Recognition via Sparse Representation|volume=31|number=2|publisher=IEEE Computer Society}}</ref>{{rp|at=Section3.2}}. The response variable <math>Y</math> is the log-volume of a cancerous tumor, and the goal is to predict it based on <math>X \in \R^6</math>, a collection of variables that are easier to measure (age of patient, log-weight of prostate, ...). Here the goal is clearly to construct <math>f</math> for ''prediction'' purposes. Indeed, we want to find an automatic mechanism that outputs a good prediction of the log-weight of the tumor given certain inputs for a new (unseen) patient. | ||
A natural measure of performance here is the <math>L_2</math>-risk employed in the introduction: | A natural measure of performance here is the <math>L_2</math>-risk employed in the introduction: | ||
Line 256: | Line 258: | ||
A natural example of fixed design regression is image denoising. Assume that <math>\mu^*_i, i \in 1, \ldots, n</math> is the grayscale value of pixel <math>i</math> of an image. We do not get to observe the image <math>\mu^*</math> but rather a noisy version of it <math>Y=\mu^*+\eps</math>. Given a library of <math>d</math> images <math>\{x_1, \ldots, x_d\}, x_j \in \R^{n}</math>, our goal is to recover the original image <math>\mu^*</math> using linear combinations of the images <math>x_1, \ldots, x_d</math>. This can be done fairly accurately (see | A natural example of fixed design regression is image denoising. Assume that <math>\mu^*_i, i \in 1, \ldots, n</math> is the grayscale value of pixel <math>i</math> of an image. We do not get to observe the image <math>\mu^*</math> but rather a noisy version of it <math>Y=\mu^*+\eps</math>. Given a library of <math>d</math> images <math>\{x_1, \ldots, x_d\}, x_j \in \R^{n}</math>, our goal is to recover the original image <math>\mu^*</math> using linear combinations of the images <math>x_1, \ldots, x_d</math>. This can be done fairly accurately (see [[#FIG:number6 |Figure]]). | ||
<div id="FIG:number6" | <div id="FIG:number6" class="d-flex justify-content-center"> | ||
[[File:Guide_e2965_6.png | 400px | thumb | Reconstruction of the digit 6: Original (left), Noisy (middle) and Reconstruction (right). Here <math>n=16\times 16=256</math> pixels. Source<ref name="RigTsy11">{{cite journal|last1=Rigollet|first1=Philippe|last2=Tsybakov|first2=Alex|last3=re|journal=Ann. Statist.|year=2011|title=Exponential screening and optimal rates of sparse estimation|volume=39|number=2}}</ref>.]] | |||
[[File: | |||
</ | |||
</div> | </div> | ||
As we will see in Remark[[#rem:lam_min |Linear Regression Model]], properly choosing the design also ensures that if <math>\MSE(\hat f)</math> is small for some linear estimator <math>\hat f(x)=x^\top \hat \theta</math>, then <math>|\hat \theta -\theta^*|_2^2</math> is also small. | |||
As we will see in Remark | |||
==Least squares estimators== | ==Least squares estimators== | ||
Throughout this section, we consider the regression model | |||
Throughout this section, we consider the regression model\eqref{EQ:regmod_matrix} with fixed design. | |||
===Unconstrained least squares estimator=== | ===Unconstrained least squares estimator=== | ||
Define the (unconstrained) ’'least squares estimator'' <math>\thetals</math> to be any vector such that | Define the (unconstrained) ’'least squares estimator'' <math>\thetals</math> to be any vector such that | ||
Line 313: | Line 308: | ||
It concludes the proof of the first statement. The second statement follows from the definition of the Moore-Penrose pseudoinverse.}} | It concludes the proof of the first statement. The second statement follows from the definition of the Moore-Penrose pseudoinverse.}} | ||
We are now going to prove our first result on the finite sample performance of the least squares estimator for fixed design. | We are now going to prove our first result on the finite sample performance of the least squares estimator for fixed design. | ||
{{proofcard|Theorem|TH:lsOI|Assume that the linear model | {{proofcard|Theorem|TH:lsOI|Assume that the linear model\eqref{EQ:regmod_matrix} holds where <math>\eps\sim \sg_n(\sigma^2)</math>. Then the least squares estimator <math>\thetals</math> satisfies | ||
<math display="block"> | <math display="block"> | ||
Line 367: | Line 362: | ||
</math> | </math> | ||
Therefore, <math>\tilde \eps\sim \sg_r(\sigma^2)</math>. | Therefore, <math>\tilde \eps\sim \sg_r(\sigma^2)</math>. | ||
To conclude the bound in expectation, observe that | To conclude the bound in expectation, observe that [[guide:0251dfcdad#LEM:subgauss_moments |Lemma]] yields | ||
<math display="block"> | <math display="block"> | ||
4\E\big[\sup_{u \in \cB_2}(\tilde \eps^\top u )^2\big]=4\sum_{i=1}^r \E[\tilde \eps_i^2]\le 16\sigma^2r\,. | 4\E\big[\sup_{u \in \cB_2}(\tilde \eps^\top u )^2\big]=4\sum_{i=1}^r \E[\tilde \eps_i^2]\le 16\sigma^2r\,. | ||
</math> | </math> | ||
Moreover, with probability <math>1-\delta</math>, it follows from the last step in the proof<ref group="Notes" >we could use | Moreover, with probability <math>1-\delta</math>, it follows from the last step in the proof<ref group="Notes" >we could use [[guide:0251dfcdad#TH:supell2 |Theorem]] directly here but at the cost of a factor 2 in the constant.</ref> of [[guide:0251dfcdad#TH:supell2 |Theorem]] that | ||
<math display="block"> | <math display="block"> | ||
\sup_{u \in \cB_2}(\tilde \eps^\top u)^2 \le 8\log(6)\sigma^2 r + 8\sigma^2\log(1/\delta)\,. | \sup_{u \in \cB_2}(\tilde \eps^\top u)^2 \le 8\log(6)\sigma^2 r + 8\sigma^2\log(1/\delta)\,. | ||
</math>}} | </math>}} | ||
{{alert-info | | |||
<span id = "rem:lam_min"/> | |||
If <math>d \le n</math> and <math>B:=\frac{\X^\top \X}{n}</math> has rank <math>d</math>, then we have | {{alert-info | If <math>d \le n</math> and <math>B:=\frac{\X^\top \X}{n}</math> has rank <math>d</math>, then we have | ||
<math display="block"> | <math display="block"> | ||
|\thetals -\theta^*|_2^2 \le \frac{\MSE(\X\thetals)}{\lambda_{\mathrm{min}} (B)}\,, | |\thetals -\theta^*|_2^2 \le \frac{\MSE(\X\thetals)}{\lambda_{\mathrm{min}} (B)}\,, | ||
</math> | </math> | ||
and we can use | and we can use [[#TH:lsOI |Theorem]] to bound <math>|\thetals -\theta^*|_2^2</math> directly. | ||
By contrast, in the high-dimensional case, we will need more structural assumptions to come to a similar conclusion. | By contrast, in the high-dimensional case, we will need more structural assumptions to come to a similar conclusion. | ||
}} | }} | ||
Line 394: | Line 389: | ||
\thetalsK \in \argmin_{\theta \in K} |Y-\X\theta|_2^2\,. | \thetalsK \in \argmin_{\theta \in K} |Y-\X\theta|_2^2\,. | ||
</math> | </math> | ||
The fundamental inequality | The fundamental inequality\eqref{EQ:fund_ineq_ls} would still hold and the bounds on the MSE may be smaller. Indeed, \eqref{EQ:bound_ls_1} can be replaced by | ||
<math display="block"> | <math display="block"> | ||
Line 419: | Line 414: | ||
It implies that the set <math>\X K=\{\X\theta, \theta \in K\} \subset \R^n</math> is also a polytope with at most <math>2d</math> vertices that are in the set <math>\X\cV=\{\X_1, -\X_1, \ldots, \X_d, -\X_d\}</math> where <math>\X_j</math> is the <math>j</math>-th column of <math>\X</math>. Indeed, <math>\X K</math> is obtained by rescaling and embedding (resp. projecting) the polytope <math>K</math> when <math>d \le n</math> (resp., <math>d \ge n</math>). Note that some columns of <math>\X</math> might not be vertices of <math>\X K</math> so that <math>\X \cV</math> might be a strict superset of the set of vertices of <math>\X K</math>. | It implies that the set <math>\X K=\{\X\theta, \theta \in K\} \subset \R^n</math> is also a polytope with at most <math>2d</math> vertices that are in the set <math>\X\cV=\{\X_1, -\X_1, \ldots, \X_d, -\X_d\}</math> where <math>\X_j</math> is the <math>j</math>-th column of <math>\X</math>. Indeed, <math>\X K</math> is obtained by rescaling and embedding (resp. projecting) the polytope <math>K</math> when <math>d \le n</math> (resp., <math>d \ge n</math>). Note that some columns of <math>\X</math> might not be vertices of <math>\X K</math> so that <math>\X \cV</math> might be a strict superset of the set of vertices of <math>\X K</math>. | ||
{{proofcard|Theorem|TH:ell1const|Let <math>K=\cB_1</math> be the unit <math>\ell_1</math> ball of <math>\R^d, d\ge 2</math> and assume that <math>\theta^* \in \cB_1</math>. Moreover, assume the conditions of | {{proofcard|Theorem|TH:ell1const|Let <math>K=\cB_1</math> be the unit <math>\ell_1</math> ball of <math>\R^d, d\ge 2</math> and assume that <math>\theta^* \in \cB_1</math>. Moreover, assume the conditions of [[#TH:lsOI |Theorem]] and that the columns of <math>\X</math> are normalized in such a way that <math>\max_j|\X_j|_2\le \sqrt{n}</math>. Then the constrained least squares estimator <math>\thetals_{\cB_1}</math> satisfies | ||
<math display="block"> | <math display="block"> | ||
Line 434: | Line 429: | ||
|\X\thetals_{\cB_1}-\X\theta^*|_2^2\le4\sup_{v \in \X K}(\eps^\top v). | |\X\thetals_{\cB_1}-\X\theta^*|_2^2\le4\sup_{v \in \X K}(\eps^\top v). | ||
</math> | </math> | ||
Observe now that since <math>\eps\sim \sg_n(\sigma^2)</math>, for any column <math>\X_j</math> such that <math>|\X_j|_2\le \sqrt{n}</math>, the random variable <math>\eps^\top \X_j\sim \sg(n\sigma^2)</math>. Therefore, applying | Observe now that since <math>\eps\sim \sg_n(\sigma^2)</math>, for any column <math>\X_j</math> such that <math>|\X_j|_2\le \sqrt{n}</math>, the random variable <math>\eps^\top \X_j\sim \sg(n\sigma^2)</math>. Therefore, applying [[guide:0251dfcdad#TH:polytope |Theorem]], we get the bound on <math>\E\big[\MSE(\X\thetalsK)\big]</math> and for any <math>t\ge 0</math>, | ||
<math display="block"> | <math display="block"> | ||
Line 444: | Line 439: | ||
2de^{-\frac{nt^2}{32\sigma^2}}\le \delta \ \Leftrightarrow \ t^2 \ge 32\sigma^2 \frac{ \log(2d)}{n} + 32 \sigma^2 \frac{ \log(1/\delta)}{n}\,. | 2de^{-\frac{nt^2}{32\sigma^2}}\le \delta \ \Leftrightarrow \ t^2 \ge 32\sigma^2 \frac{ \log(2d)}{n} + 32 \sigma^2 \frac{ \log(1/\delta)}{n}\,. | ||
</math>}} | </math>}} | ||
Note that the proof of | Note that the proof of [[#TH:lsOI |Theorem]] also applies to <math>\thetals_{\cB_1}</math> (exercise!) so that <math>\X \thetals_{\cB_1}</math> benefits from the best of both rates, | ||
<math display="block"> | <math display="block"> | ||
Line 456: | Line 451: | ||
|\theta|_0=\sum_{j=1}^d \1(\theta_j \neq 0)\,. | |\theta|_0=\sum_{j=1}^d \1(\theta_j \neq 0)\,. | ||
</math> | </math> | ||
We call a vector <math>\theta</math> with | We call a vector <math>\theta</math> with ''small" <math>\ell_0</math> norm a ’'sparse'' vector. More precisely, if <math>|\theta|_0\le k</math>, we say that <math>\theta</math> is a <math>k</math>-sparse vector. We also call ''support'' of <math>\theta</math> the set | ||
<math display="block"> | <math display="block"> | ||
\supp(\theta)=\big\{j \in \{1, \ldots, d\}\,:\, \theta_j \neq 0\big\} | \supp(\theta)=\big\{j \in \{1, \ldots, d\}\,:\, \theta_j \neq 0\big\} | ||
</math> | </math> | ||
so that <math>|\theta|_0=\card(\supp(\theta))=:|\supp(\theta)|</math> | so that <math>|\theta|_0=\card(\supp(\theta))=:|\supp(\theta)|</math>. | ||
{{alert-info | | {{alert-info | | ||
The <math>\ell_0</math> terminology and notation comes from the fact that | The <math>\ell_0</math> terminology and notation comes from the fact that | ||
Line 476: | Line 471: | ||
</math> | </math> | ||
In this section, our goal is to control the <math>\MSE</math> of <math>\thetalsK</math> when <math>K=\cB_0(k)</math>. Note that computing <math>\thetals_{\cB_0(k)}</math> essentially requires computing <math>\binom{d}{k}</math> least squares estimators, which is an exponential number in <math>k</math>. In practice this will be hard (or even impossible) but it is interesting to understand the statistical properties of this estimator and to use them as a benchmark. | In this section, our goal is to control the <math>\MSE</math> of <math>\thetalsK</math> when <math>K=\cB_0(k)</math>. Note that computing <math>\thetals_{\cB_0(k)}</math> essentially requires computing <math>\binom{d}{k}</math> least squares estimators, which is an exponential number in <math>k</math>. In practice this will be hard (or even impossible) but it is interesting to understand the statistical properties of this estimator and to use them as a benchmark. | ||
{{proofcard|Theorem|TH:bss1|Fix a positive integer <math>k \le d/2</math>. Let <math>K=\cB_0(k)</math> be set of <math>k</math>-sparse vectors of <math>\R^d</math> and assume that <math>\theta^*\in \cB_0(k)</math>. Moreover, assume the conditions of | {{proofcard|Theorem|TH:bss1|Fix a positive integer <math>k \le d/2</math>. Let <math>K=\cB_0(k)</math> be set of <math>k</math>-sparse vectors of <math>\R^d</math> and assume that <math>\theta^*\in \cB_0(k)</math>. Moreover, assume the conditions of [[#TH:lsOI |Theorem]]. Then, for any <math>\delta > 0</math>, with probability <math>1-\delta</math>, it holds | ||
<math display="block"> | <math display="block"> | ||
\MSE(\X\thetals_{\cB_0(k)})\lesssim \frac{\sigma^2}{n}\log\binom{d}{2k} + \frac{\sigma^2k}{n} +\frac{\sigma^2}{n}\log(1/\delta)\,. | \MSE(\X\thetals_{\cB_0(k)})\lesssim \frac{\sigma^2}{n}\log\binom{d}{2k} + \frac{\sigma^2k}{n} +\frac{\sigma^2}{n}\log(1/\delta)\,. | ||
</math> | </math> | ||
|We begin as in the proof of | |We begin as in the proof of [[#TH:lsOI |Theorem]] to get\eqref{EQ:bound_ls_1}: | ||
<math display="block"> | <math display="block"> | ||
Line 508: | Line 503: | ||
\p\big(\max_{|S|= 2k}\sup_{u \in \cB_2^{r_S}}(\tilde \eps^\top u)^2 > t\big) \le \sum_{|S|= 2k} \p\big( \sup_{u \in \cB_2^{r_S}}(\tilde \eps^\top u)^2 > t\big) | \p\big(\max_{|S|= 2k}\sup_{u \in \cB_2^{r_S}}(\tilde \eps^\top u)^2 > t\big) \le \sum_{|S|= 2k} \p\big( \sup_{u \in \cB_2^{r_S}}(\tilde \eps^\top u)^2 > t\big) | ||
</math> | </math> | ||
It follows from the proof of | It follows from the proof of [[guide:0251dfcdad#TH:supell2 |Theorem]] that for any <math>|S| \le 2k</math>, | ||
<math display="block"> | <math display="block"> | ||
Line 551: | Line 546: | ||
<math display="block"> | <math display="block"> | ||
\begin{equation*} | \begin{equation*} | ||
\left(1+\frac1k\right)^k\le e. | \left(1+\frac1k\right)^k\le e. | ||
\end{equation*} | \end{equation*} | ||
</math>}} | </math>}} | ||
It immediately leads to the following corollary: | It immediately leads to the following corollary: | ||
{{proofcard|Corollary|COR:bss1|Under the assumptions of | {{proofcard|Corollary|COR:bss1|Under the assumptions of [[#TH:bss1 |Theorem]], for any <math>\delta > 0</math>, with probability at least <math>1-\delta</math>, it holds | ||
<math display="block"> | <math display="block"> | ||
Line 565: | Line 560: | ||
\MSE(\X\thetals_{\cB_0(k)})\le C_\delta\frac{\sigma^2k}{n}\log \Big(\frac{ed}{2k}\Big)\,. | \MSE(\X\thetals_{\cB_0(k)})\le C_\delta\frac{\sigma^2k}{n}\log \Big(\frac{ed}{2k}\Big)\,. | ||
</math> | </math> | ||
Comparing this result with | Comparing this result with [[#TH:lsOI |Theorem]] with <math>r=k</math>, we see that the price to pay for not knowing the support of <math>\theta^*</math> but only its size, is a logarithmic factor in the dimension <math>d</math>. | ||
This result immediately leads to the following bound in expectation. | This result immediately leads to the following bound in expectation. | ||
{{proofcard|Corollary|COR:bss2|Under the assumptions of | {{proofcard|Corollary|COR:bss2|Under the assumptions of [[#TH:bss1 |Theorem]], | ||
<math display="block"> | <math display="block"> | ||
\E\big[\MSE(\X\thetals_{\cB_0(k)})\big]\lesssim\frac{\sigma^2k}{n}\log \Big(\frac{ed}{k}\Big)\,. | \E\big[\MSE(\X\thetals_{\cB_0(k)})\big]\lesssim\frac{\sigma^2k}{n}\log \Big(\frac{ed}{k}\Big)\,. | ||
</math> | </math> | ||
|It follows from | |It follows from\eqref{EQ:prMSEl0HP} that for any <math>H\ge 0</math>, | ||
<math display="block"> | <math display="block"> | ||
Line 595: | Line 590: | ||
==The Gaussian Sequence Model== | ==The Gaussian Sequence Model== | ||
The Gaussian Sequence Model is a toy model that has received a lot of attention, mostly in the eighties. The main reason for its popularity is that it carries already most of the insight of nonparametric estimation. While the model looks very simple it allows to carry deep ideas that extend beyond its framework and in particular to the linear regression model that we are interested in. Unfortunately, we will only cover a small part of these ideas and the interested reader should definitely look at the excellent books by A. Tsybakov | The Gaussian Sequence Model is a toy model that has received a lot of attention, mostly in the eighties. The main reason for its popularity is that it carries already most of the insight of nonparametric estimation. While the model looks very simple it allows to carry deep ideas that extend beyond its framework and in particular to the linear regression model that we are interested in. Unfortunately, we will only cover a small part of these ideas and the interested reader should definitely look at the excellent books by A. Tsybakov<ref name="Tsy09">{{cite journal|last=LeCam|first=L.|journal=Ann. Statist.|year=1973|title=Convergence of estimates under dimensionality restrictions|volume=1}}</ref>{{rp|at=Chapter3}} and I. Johnstone<ref name="Joh11">{{cite journal|last1=Ga{ö{\i}}ffas|first1=St{{\'e}}phane|last2=Lecu{{\'e}}|first2=Guillaume|journal=J. Mach. Learn. Res.|year=2011|title=Hyper-sparse optimal aggregation|volume=12}}</ref>. | ||
The model is as follows: | The model is as follows: | ||
Line 604: | Line 599: | ||
\end{equation} | \end{equation} | ||
</math> | </math> | ||
where <math>\eps_1, \ldots, \eps_d</math> are | where <math>\eps_1, \ldots, \eps_d</math> are i.i.d <math>\cN(0,\sigma^2)</math> random variables. Note that often, <math>d</math> is taken equal to <math>\infty</math> in this sequence model and we will also discuss this case. Its links to nonparametric estimation will become clearer in Chapter [[guide:8dff7bda6c#chap:misspecified |Misspecified Linear Models]]. The goal here is to estimate the unknown vector <math>\theta^*</math>. | ||
===The sub-Gaussian Sequence Model=== | ===The sub-Gaussian Sequence Model=== | ||
Note first that the model | Note first that the model\eqref{EQ:gsm} is a special case of the linear model with fixed design\eqref{EQ:regmod} with <math>n=d</math> and <math>f(x_i)=x_i^\top \theta^*</math>, where <math>x_1, \ldots, x_n</math> form the canonical basis <math> e_1, \dots, e_n </math> of <math>\R^n</math> and <math>\eps</math> has a Gaussian distribution. Therefore, <math>n=d</math> is both the dimension of the parameter <math>\theta</math> and the number of observations and it looks like we have chosen to index this problem by <math>d</math> rather than <math>n</math> somewhat arbitrarily. We can bring <math>n</math> back into the picture, by observing that this model encompasses slightly more general choices for the design matrix <math>\X</math> as long as it satisfies the following assumption. | ||
'''Assumption''' <math>\textsf{ORT}</math> | |||
The design matrix satisfies | The design matrix satisfies | ||
Line 615: | Line 612: | ||
</math> | </math> | ||
where <math>I_d</math> denotes the identity matrix of <math>\R^d</math>. | where <math>I_d</math> denotes the identity matrix of <math>\R^d</math>. | ||
Assumption | Assumption <math>\textsf{ORT}</math> allows for cases where <math>d\le n</math> but not <math>d > n</math> (high dimensional case) because of obvious rank constraints. In particular, it means that the <math>d</math> columns of <math>\X</math> are orthogonal in <math>\R^n</math> and all have norm <math>\sqrt{n}</math>. | ||
Under this assumption, it follows from the linear regression model | Under this assumption, it follows from the linear regression model\eqref{EQ:regmod_matrix} that | ||
<math display="block"> | <math display="block"> | ||
Line 625: | Line 622: | ||
\end{align*} | \end{align*} | ||
</math> | </math> | ||
where <math>\xi=(\xi_1, \ldots, \xi_d)\sim \sg_d(\sigma^2/n)</math> (If <math>\eps</math> is Gaussian, we even have <math>\xi \sim \cN_d(0, \sigma^2/n)</math>). As a result, under the | where <math>\xi=(\xi_1, \ldots, \xi_d)\sim \sg_d(\sigma^2/n)</math> (If <math>\eps</math> is Gaussian, we even have <math>\xi \sim \cN_d(0, \sigma^2/n)</math>). As a result, under the Assumption <math>\textsf{ORT}</math>, when <math>\xi</math> is Gaussian, the linear regression model\eqref{EQ:regmod_matrix} is equivalent to the Gaussian Sequence Model\eqref{EQ:gsm} up to a transformation of the data <math>Y</math> and a rescaling of the variance. Moreover, for any estimator <math>\hat \theta \in \R^d</math>, under <math>\textsf{ORT}</math>, it follows from\eqref{EQ:mse_matrix} that | ||
<math display="block"> | <math display="block"> | ||
\MSE(\X\hat \theta)=(\hat \theta -\theta^*)^\top \frac{\X^\top \X}{n}(\hat \theta -\theta^*)=|\hat \theta - \theta^*|_2^2\,. | \MSE(\X\hat \theta)=(\hat \theta -\theta^*)^\top \frac{\X^\top \X}{n}(\hat \theta -\theta^*)=|\hat \theta - \theta^*|_2^2\,. | ||
</math> | </math> | ||
Furthermore, for any <math>\theta \in \R^d</math>, the | Furthermore, for any <math>\theta \in \R^d</math>, the Assumption <math>\textsf{ORT}</math> yields, | ||
<math display="block"> | <math display="block"> | ||
Line 657: | Line 654: | ||
</math> | </math> | ||
where <math>\xi\sim \sg_d(\sigma^2/n)</math>. | where <math>\xi\sim \sg_d(\sigma^2/n)</math>. | ||
In this section, we can actually completely ‘`forget" about our original model | In this section, we can actually completely ‘`forget" about our original model\eqref{EQ:regmod_matrix}. In particular we can define this model independently of Assumption <math>\textsf{ORT}</math> and thus for any values of <math>n</math> and <math>d</math>. | ||
The sub-Gaussian sequence model and the Gaussian sequence model are called ’'direct'' (observation) problems as opposed to ''inverse problems'' where the goal is to estimate the parameter <math>\theta^*</math> only from noisy observations of its image through an operator. The linear regression model is one such inverse problem where the matrix <math>\X</math> plays the role of a linear operator. However, in these notes, we never try to invert the operator. See <ref name="Cav11">{{citation|last1=Alex|last2=re|first2=B.|year=2004|title=Statistique appliqu\'ee}}</ref> for an interesting survey on the statistical theory of inverse problems. | The sub-Gaussian sequence model and the Gaussian sequence model are called ’'direct'' (observation) problems as opposed to ''inverse problems'' where the goal is to estimate the parameter <math>\theta^*</math> only from noisy observations of its image through an operator. The linear regression model is one such inverse problem where the matrix <math>\X</math> plays the role of a linear operator. However, in these notes, we never try to invert the operator. See <ref name="Cav11">{{citation|last1=Alex|last2=re|first2=B.|year=2004|title=Statistique appliqu\'ee}}</ref> for an interesting survey on the statistical theory of inverse problems. | ||
===Sparsity adaptive thresholding estimators=== | ===Sparsity adaptive thresholding estimators=== | ||
If we knew a priori that <math>\theta</math> was <math>k</math>-sparse, we could directly employ | If we knew a priori that <math>\theta</math> was <math>k</math>-sparse, we could directly employ [[#COR:bss1 |Corollary]] to obtain that with probability at least <math>1-\delta</math>, we have | ||
<math display="block"> | <math display="block"> | ||
\MSE(\X\thetals_{\cB_0(k)})\le C_\delta\frac{\sigma^2k}{n}\log\Big(\frac{ed}{2k}\Big)\,. | \MSE(\X\thetals_{\cB_0(k)})\le C_\delta\frac{\sigma^2k}{n}\log\Big(\frac{ed}{2k}\Big)\,. | ||
</math> | </math> | ||
As we will see, the | As we will see, the Assumption <math>\textsf{ORT}</math> gives us the luxury to not know <math>k</math> and yet ''adapt'' to its value. Adaptation means that we can construct an estimator that does not require the knowledge of <math>k</math> (the smallest such that <math>|\theta^*|_0\le k</math>) and yet perform as well as <math>\thetals_{\cB_0(k)}</math>, up to a multiplicative constant. | ||
Let us begin with some heuristic considerations to gain some intuition. Assume the sub-Gaussian sequence model | Let us begin with some heuristic considerations to gain some intuition. Assume the sub-Gaussian sequence model\eqref{EQ:sGSM}. If nothing is known about <math>\theta^*</math> it is natural to estimate it using the least squares estimator <math>\thetals=y</math>. In this case, | ||
<math display="block"> | <math display="block"> | ||
Line 673: | Line 670: | ||
</math> | </math> | ||
where the last inequality holds with probability at least <math>1-\delta</math>. This is actually what we are looking for if <math>k=Cd</math> for some positive constant <math>C\le 1</math>. The problem with this approach is that it does not use the fact that <math>k</math> may be much smaller than <math>d</math>, which happens when <math>\theta^*</math> has many zero coordinates. | where the last inequality holds with probability at least <math>1-\delta</math>. This is actually what we are looking for if <math>k=Cd</math> for some positive constant <math>C\le 1</math>. The problem with this approach is that it does not use the fact that <math>k</math> may be much smaller than <math>d</math>, which happens when <math>\theta^*</math> has many zero coordinates. | ||
If <math>\theta^*_j=0</math>, then, <math>y_j=\xi_j</math>, which is a sub-Gaussian random variable with variance proxy <math>\sigma^2/n</math>. In particular, we know from | If <math>\theta^*_j=0</math>, then, <math>y_j=\xi_j</math>, which is a sub-Gaussian random variable with variance proxy <math>\sigma^2/n</math>. In particular, we know from [[guide:0251dfcdad#lem:subgauss |Lemma]] that with probability at least <math>1-\delta</math>, | ||
<math display="block"> | <math display="block"> | ||
Line 694: | Line 691: | ||
</math> | </math> | ||
for <math>j=1, \ldots, d</math>. In short, we can write <math>\thetahard_j=y_j\1(|y_j| > 2\tau)</math>.}} | for <math>j=1, \ldots, d</math>. In short, we can write <math>\thetahard_j=y_j\1(|y_j| > 2\tau)</math>.}} | ||
From our above consideration, we are tempted to choose <math>\tau</math> as in | From our above consideration, we are tempted to choose <math>\tau</math> as in\eqref{EQ:tau1}. Yet, this threshold is not large enough. Indeed, we need to choose <math>\tau</math> such that <math>|\xi_j|\le \tau</math> ''simultaneously'' for all <math>j</math>. This can be done using a maximal inequality. Namely, [[guide:0251dfcdad#TH:finitemax |Theorem]] ensures that with probability at least <math>1-\delta</math>, | ||
<math display="block"> | <math display="block"> | ||
Line 700: | Line 697: | ||
</math> | </math> | ||
It yields the following theorem. | It yields the following theorem. | ||
{{proofcard|Theorem|TH:hard|Consider the linear regression model | {{proofcard|Theorem|TH:hard|Consider the linear regression model\eqref{EQ:regmod_matrix} under the Assumption <math>\textsf{ORT}</math> or, equivalenty, the sub-Gaussian sequence model\eqref{EQ:sGSM}. Then the hard thresholding estimator <math>\thetahard</math> with threshold | ||
<math display="block"> | <math display="block"> | ||
Line 725: | Line 722: | ||
\cA=\Big\{\max_j|\xi_j|\le \tau\big\}\,, | \cA=\Big\{\max_j|\xi_j|\le \tau\big\}\,, | ||
</math> | </math> | ||
and recall that | and recall that [[guide:0251dfcdad#TH:finitemax |Theorem]] yields <math>\p(\cA)\ge 1-\delta</math>. On the event <math>\cA</math>, the following holds for any <math>j=1, \ldots, d</math>. | ||
First, observe that | First, observe that | ||
Line 748: | Line 745: | ||
|\thetahard_j-\theta^*_j|&=|y_j-\theta^*_j|\1(|y_j| > 2\tau)+ |\theta^*_j|\1(|y_j|\le2\tau)&\\ | |\thetahard_j-\theta^*_j|&=|y_j-\theta^*_j|\1(|y_j| > 2\tau)+ |\theta^*_j|\1(|y_j|\le2\tau)&\\ | ||
&\le \tau\1(|y_j| > 2\tau)+|\theta^*_j|\1(|y_j|\le2\tau)& \\\ | &\le \tau\1(|y_j| > 2\tau)+|\theta^*_j|\1(|y_j|\le2\tau)& \\\ | ||
&\le \tau\1(|\theta^*_j| > \tau)+|\theta^*_j|\1(|\theta^*_j|\le 3\tau)& \text{by} | &\le \tau\1(|\theta^*_j| > \tau)+|\theta^*_j|\1(|\theta^*_j|\le 3\tau)& \text{by}\eqref{EQ:prthhard1} \text{ and}\eqref{EQ:prthhard2}\\ | ||
&\le 4\min(|\theta^*_j|,\tau) | &\le 4\min(|\theta^*_j|,\tau) | ||
\end{align*} | \end{align*} | ||
Line 788: | Line 785: | ||
\thetasoft_j=\Big(1-\frac{2\tau}{|y_j|}\Big)_+y_j | \thetasoft_j=\Big(1-\frac{2\tau}{|y_j|}\Big)_+y_j | ||
</math> | </math> | ||
<div id="FIG:threshold" class="d-flex justify-content-center"> | <div id="FIG:threshold" class="d-flex justify-content-center"> | ||
[[File: | | [[File:Guide_e2965_hs.png | 600px | thumb | Transformation applied to <math>y_j</math> with <math>2\tau=1</math> to obtain the hard (left) and soft (right) thresholding estimators]] | ||
</div> | </div> | ||
==High-dimensional linear regression== | ==High-dimensional linear regression== | ||
===The BIC and Lasso estimators=== | ===The BIC and Lasso estimators=== | ||
It can be shown (see Problem | It can be shown (see Problem[[#EXO:sparse:variation |Linear Regression Model]]) that the hard and soft thresholding estimators are solutions of the following penalized empirical risk minimization problems: | ||
<math display="block"> | <math display="block"> | ||
Line 804: | Line 801: | ||
\end{align*} | \end{align*} | ||
</math> | </math> | ||
In view of | In view of\eqref{EQ:ERM_sGSM}, under the Assumption <math>\textsf{ORT}</math>, the above variational definitions can be written as | ||
<math display="block"> | <math display="block"> | ||
Line 812: | Line 809: | ||
\end{align*} | \end{align*} | ||
</math> | </math> | ||
When the | When the Assumption <math>\textsf{ORT}</math> is not satisfied, they no longer correspond to thresholding estimators but can still be defined as above. We change the constant in the threshold parameters for future convenience.{{defncard|label=|id=|Fix <math>\tau > 0</math> and assume the linear regression model\eqref{EQ:regmod_matrix}. The BIC<ref group="Notes" >Note that it minimizes the Bayes Information Criterion (BIC) employed in the traditional literature of asymptotic statistics if <math>\tau=\sqrt{\log(d)/n}</math>. We will use the same value below, up to multiplicative constants (it's the price to pay to get non-asymptotic results).</ref> estimator of <math>\theta^*</math> is defined by any <math>\thetabic</math> such that | ||
<math display="block"> | <math display="block"> | ||
Line 835: | Line 832: | ||
By contrast, computing the Lasso estimator is a convex problem and there exist many efficient algorithms to solve it. We will not describe this optimization problem in detail but only highlight a few of the best known algorithms: | By contrast, computing the Lasso estimator is a convex problem and there exist many efficient algorithms to solve it. We will not describe this optimization problem in detail but only highlight a few of the best known algorithms: | ||
<ul><li> Probably the most popular method among statisticians relies on coordinate gradient descent. It is implemented in the \textsf{glmnet} package in \textsf{R} <ref name="FriHasTib10">{{cite journal|last1=Friedman|first1=Jerome|last2=Hastie|first2=Trevor|last3=Tibshirani|first3=Robert|journal=Journal of Statistical Software|title=Regularization paths for generalized linear models via coordinate descent|volume=33|number=1|publisher=NIH Public Access}}</ref>, | <ul><li> Probably the most popular method among statisticians relies on coordinate gradient descent. It is implemented in the <math>\textsf{glmnet}</math> package in <math>\textsf{R}</math> <ref name="FriHasTib10">{{cite journal|last1=Friedman|first1=Jerome|last2=Hastie|first2=Trevor|last3=Tibshirani|first3=Robert|journal=Journal of Statistical Software|title=Regularization paths for generalized linear models via coordinate descent|volume=33|number=1|publisher=NIH Public Access}}</ref>, | ||
</li> | </li> | ||
<li> An interesting method called LARS <ref name="EfrHasJoh04">{{cite journal|last1=Efron|first1=Bradley|last2=Hastie|first2=Trevor|last3=Johnstone|first3=Iain|last4=Tibshirani|first4=Robert|journal=Ann. Statist.|year=2004|title=Least angle regression|volume=32|number=2}}</ref> computes the entire ''regularization path'', i.e., the solution of the convex problem for all values of <math>\tau</math>. It relies on the fact that, as a function of <math>\tau</math>, the solution <math>\thetalasso</math> is a piecewise linear function (with values in <math>\R^d</math>). Yet this method proved to be too slow for very large problems and has been replaced by <TT>glmnet</TT> which computes solutions for values of <math>\tau</math> on a grid much faster. | <li> An interesting method called LARS <ref name="EfrHasJoh04">{{cite journal|last1=Efron|first1=Bradley|last2=Hastie|first2=Trevor|last3=Johnstone|first3=Iain|last4=Tibshirani|first4=Robert|journal=Ann. Statist.|year=2004|title=Least angle regression|volume=32|number=2}}</ref> computes the entire ''regularization path'', i.e., the solution of the convex problem for all values of <math>\tau</math>. It relies on the fact that, as a function of <math>\tau</math>, the solution <math>\thetalasso</math> is a piecewise linear function (with values in <math>\R^d</math>). Yet this method proved to be too slow for very large problems and has been replaced by <TT>glmnet</TT> which computes solutions for values of <math>\tau</math> on a grid much faster. | ||
Line 848: | Line 845: | ||
===Analysis of the BIC estimator=== | ===Analysis of the BIC estimator=== | ||
While computationally hard to implement, the BIC estimator gives us a good benchmark for sparse estimation. Its performance is similar to that of <math>\thetahard</math> but without requiring the | While computationally hard to implement, the BIC estimator gives us a good benchmark for sparse estimation. Its performance is similar to that of <math>\thetahard</math> but without requiring the Assumption <math>\textsf{ORT}</math>. | ||
{{proofcard|Theorem|TH:BIC|Assume that the linear model | {{proofcard|Theorem|TH:BIC|Assume that the linear model\eqref{EQ:regmod_matrix} holds, where <math>\eps \sim \sg_n(\sigma^2)</math> and that <math>|\theta^*|_0\ge 1</math>. Then, the BIC estimator <math>\thetabic</math> with regularization parameter | ||
<math display="block"> | <math display="block"> | ||
Line 919: | Line 916: | ||
\end{align*} | \end{align*} | ||
</math> | </math> | ||
Moreover, using the <math>\eps</math>-net argument from | Moreover, using the <math>\eps</math>-net argument from [[guide:0251dfcdad#TH:supell2 |Theorem]], we get for <math>|S|=k</math>, | ||
<math display="block"> | <math display="block"> | ||
Line 929: | Line 926: | ||
\end{align*} | \end{align*} | ||
</math> | </math> | ||
where, in the last inequality, we used the definition | where, in the last inequality, we used the definition\eqref{EQ:taubic} of <math>\tau</math>. | ||
Putting everything together, we get | Putting everything together, we get | ||
Line 937: | Line 934: | ||
& \sum_{k=1}^d\sum_{|S|=k} \exp\Big(-\frac{t}{32\sigma^2}- 2k\log(ed)+|\theta^*|_0\log(12)\Big)\\ | & \sum_{k=1}^d\sum_{|S|=k} \exp\Big(-\frac{t}{32\sigma^2}- 2k\log(ed)+|\theta^*|_0\log(12)\Big)\\ | ||
&= \sum_{k=1}^d \binom{d}{k} \exp\Big(-\frac{t}{32\sigma^2}- 2k\log(ed)+|\theta^*|_0\log(12)\Big)\\ | &= \sum_{k=1}^d \binom{d}{k} \exp\Big(-\frac{t}{32\sigma^2}- 2k\log(ed)+|\theta^*|_0\log(12)\Big)\\ | ||
&\le \sum_{k=1}^d \exp\Big(-\frac{t}{32\sigma^2}-k\log(ed)+|\theta^*|_0\log(12)\Big)& \text{by | &\le \sum_{k=1}^d \exp\Big(-\frac{t}{32\sigma^2}-k\log(ed)+|\theta^*|_0\log(12)\Big)& \text{by [[#lem:nchoosek |Lemma]]}\\ | ||
&= \sum_{k=1}^d(ed)^{-k} \exp\Big(-\frac{t}{32\sigma^2} + |\theta^*|_0\log(12)\Big)\\ | &= \sum_{k=1}^d(ed)^{-k} \exp\Big(-\frac{t}{32\sigma^2} + |\theta^*|_0\log(12)\Big)\\ | ||
&\le \exp\Big(-\frac{t}{32\sigma^2} + |\theta^*|_0\log(12)\Big)\,. | &\le \exp\Big(-\frac{t}{32\sigma^2} + |\theta^*|_0\log(12)\Big)\,. | ||
\end{align*} | \end{align*} | ||
</math> | </math> | ||
To conclude the proof, choose <math>t=32\sigma^2 |\theta^*|_0\log(12) + 32\sigma^2\log(1/\delta)</math> and observe that combined with | To conclude the proof, choose <math>t=32\sigma^2 |\theta^*|_0\log(12) + 32\sigma^2\log(1/\delta)</math> and observe that combined with\eqref{EQ:pr_bic_1}, it yields with probability <math>1-\delta</math>, | ||
<math display="block"> | <math display="block"> | ||
Line 951: | Line 948: | ||
\end{align*} | \end{align*} | ||
</math>}} | </math>}} | ||
It follows from | It follows from [[#TH:BIC |Theorem]] that <math>\thetabic</math> ’'adapts'' to the unknown sparsity of <math>\theta^*</math>, just like <math>\thetahard</math>. Moreover, this holds under no assumption on the design matrix <math>\X</math>. | ||
===Analysis of the Lasso estimator=== | ===Analysis of the Lasso estimator=== | ||
====Slow rate for the Lasso estimator==== | ====Slow rate for the Lasso estimator==== | ||
The properties of the BIC estimator are quite impressive. It shows that under no assumption on <math>\X</math>, one can mimic two oracles: (i) the oracle that knows the support of <math>\theta^*</math> (and computes least squares on this support), up to a <math>\log(ed)</math> term and (ii) the oracle that knows the sparsity <math>|\theta^*|_0</math> of <math>\theta^*</math>, up to a smaller logarithmic term <math>\log(ed/|\theta^*|_0)</math>, which is subsumed by <math>\log(ed)</math>. Actually, the <math> \log(ed) </math> can even be improved to <math> \log(ed/|\theta^\ast|_0) </math> by using a modified BIC estimator (see Problem | The properties of the BIC estimator are quite impressive. It shows that under no assumption on <math>\X</math>, one can mimic two oracles: (i) the oracle that knows the support of <math>\theta^*</math> (and computes least squares on this support), up to a <math>\log(ed)</math> term and (ii) the oracle that knows the sparsity <math>|\theta^*|_0</math> of <math>\theta^*</math>, up to a smaller logarithmic term <math>\log(ed/|\theta^*|_0)</math>, which is subsumed by <math>\log(ed)</math>. Actually, the <math> \log(ed) </math> can even be improved to <math> \log(ed/|\theta^\ast|_0) </math> by using a modified BIC estimator (see Problem[[#EXO:sparse:betterbic |Linear Regression Model]]). | ||
The Lasso estimator is a bit more difficult to analyze because, by construction, it should more naturally adapt to the unknown <math>\ell_1</math>-norm of <math>\theta^*</math>. This can be easily shown as in the next theorem, analogous to | The Lasso estimator is a bit more difficult to analyze because, by construction, it should more naturally adapt to the unknown <math>\ell_1</math>-norm of <math>\theta^*</math>. This can be easily shown as in the next theorem, analogous to [[#TH:ell1const |Theorem]]. | ||
{{proofcard|Theorem|TH:lasso_slow|Assume that the linear model | {{proofcard|Theorem|TH:lasso_slow|Assume that the linear model\eqref{EQ:regmod_matrix} holds where <math>\eps\sim\sg_n(\sigma^2)</math>. Moreover, assume that the columns of <math>\X</math> are normalized in such a way that <math>\max_j|\X_j|_2\le \sqrt{n}</math>. Then, the Lasso estimator <math>\thetalasso</math> with regularization parameter | ||
<math display="block"> | <math display="block"> | ||
Line 976: | Line 973: | ||
\frac1n|Y-\X\thetalasso|_2^2 + 2\tau|\thetalasso|_1 \le \frac1n|Y-\X\theta^*|_2^2 + 2\tau|\theta^*|_1\,. | \frac1n|Y-\X\thetalasso|_2^2 + 2\tau|\thetalasso|_1 \le \frac1n|Y-\X\theta^*|_2^2 + 2\tau|\theta^*|_1\,. | ||
</math> | </math> | ||
Using | Using Höolder's inequality, it implies | ||
<math display="block"> | <math display="block"> | ||
Line 994: | Line 991: | ||
<math display="block"> | <math display="block"> | ||
\begin{equation*} | \begin{equation*} | ||
|\X\thetalasso-\X\theta^*|_2^2\le 4n\tau|\theta^*|_1\,. | |\X\thetalasso-\X\theta^*|_2^2\le 4n\tau|\theta^*|_1\,. | ||
\end{equation*} | \end{equation*} | ||
</math>}} | </math>}} | ||
Notice that the regularization parameter | Notice that the regularization parameter\eqref{EQ:taulasso} depends on the confidence level <math>\delta</math>. This is not the case for the BIC estimator (see\eqref{EQ:taubic}). | ||
The rate in | The rate in [[#TH:lasso_slow |Theorem]] is of order <math>\sqrt{(\log d)/n}</math> (''slow rate''), which is much slower than the rate of order <math>(\log d)/n</math> (''fast rate'') for the BIC estimator. Hereafter, we show that fast rates can also be achieved by the computationally efficient Lasso estimator, but at the cost of a much stronger condition on the design matrix <math>\X</math>. | ||
====Incoherence==== | ====Incoherence==== | ||
Line 1,024: | Line 1,021: | ||
</ul> | </ul> | ||
\end{assumption} | \end{assumption} | ||
Note that Assumption | Note that Assumption <math>\textsf{ORT}</math> arises as the limiting case of <math>\mathsf{INC}(k)</math> as <math>k \to \infty</math>. However, while Assumption <math>\textsf{ORT}</math> requires <math>d \le n</math>, here we may have <math>d \gg n</math> as illustrated in [[#prop:INCRad |Proposition]] below. To that end, we simply have to show that there exists a matrix that satisfies <math>\mathsf{INC}(k)</math> even for <math>d > n</math>. We resort to the ''probabilistic method'' <ref name="AloSpe08">{{citation|last1=Alex|last2=re|first2=B.|year=2004|title=Statistique appliqu\'ee}}</ref>. The idea of this method is that if we can find a probability measure that assigns positive probability to objects that satisfy a certain property, then there must exist objects that satisfy said property. | ||
In our case, we consider the following probability distribution on random matrices with entries in <math>\{\pm 1\}</math>. Let the design matrix <math>\X</math> have entries that are | In our case, we consider the following probability distribution on random matrices with entries in <math>\{\pm 1\}</math>. Let the design matrix <math>\X</math> have entries that are i.i.d Rademacher <math>(\pm 1)</math> random variables. We are going to show that most realizations of this random matrix satisfy Assumption<math>\mathsf{INC}(k)</math> for large enough <math>n</math>. | ||
{{proofcard|Proposition|prop:INCRad|Let <math>\X \in \R^{n\times d}</math> be a random matrix with entries <math>X_{ij}, i=1,\ldots, n, j=1, \ldots, d</math>, that are | {{proofcard|Proposition|prop:INCRad|Let <math>\X \in \R^{n\times d}</math> be a random matrix with entries <math>X_{ij}, i=1,\ldots, n, j=1, \ldots, d</math>, that are i.i.d Rademacher <math>(\pm 1)</math> random variables. Then, <math>\X</math> has incoherence <math>k</math> with probability <math>1-\delta</math> as soon as | ||
<math display="block"> | <math display="block"> | ||
n\ge 2^{11}k^2\log(1/\delta)+2^{13}k^2\log(d)\,. | n\ge 2^{11}k^2\log(1/\delta)+2^{13}k^2\log(d)\,. | ||
</math> | </math> | ||
It implies that there exist matrices that satisfy Assumption | It implies that there exist matrices that satisfy Assumption<math>\mathsf{INC}(k)</math> for | ||
<math display="block"> | <math display="block"> | ||
n \geq C k^2\log(d)\,, | n \geq C k^2\log(d)\,, | ||
Line 1,048: | Line 1,045: | ||
\frac{1}{n}\sum_{i=1}^n \eps_{i,j}\eps_{i,k}=\frac{1}{n}\sum_{i=1}^n \xi_{i}^{(j,k)}\,, | \frac{1}{n}\sum_{i=1}^n \eps_{i,j}\eps_{i,k}=\frac{1}{n}\sum_{i=1}^n \xi_{i}^{(j,k)}\,, | ||
</math> | </math> | ||
where for each pair <math>(j,k)</math>, <math>\xi_i^{(j,k)}=\eps_{i,j}\eps_{i,k}</math>, so that <math>\xi_1^{(j,k)}, \ldots, \xi_n^{(j,k)}</math> are | where for each pair <math>(j,k)</math>, <math>\xi_i^{(j,k)}=\eps_{i,j}\eps_{i,k}</math>, so that <math>\xi_1^{(j,k)}, \ldots, \xi_n^{(j,k)}</math> are i.i.d Rademacher random variables. | ||
Therefore, we get that for any <math>t > 0</math>, | Therefore, we get that for any <math>t > 0</math>, | ||
Line 1,055: | Line 1,052: | ||
\p\left(\left|\frac{\X^\top \X}{n}-I_d\right|_\infty > t\right) &=\p\Big(\max_{j\neq k}\Big|\frac{1}{n}\sum_{i=1}^n \xi_{i}^{(j,k)}\Big| > t\Big)&\\ | \p\left(\left|\frac{\X^\top \X}{n}-I_d\right|_\infty > t\right) &=\p\Big(\max_{j\neq k}\Big|\frac{1}{n}\sum_{i=1}^n \xi_{i}^{(j,k)}\Big| > t\Big)&\\ | ||
&\le \sum_{j\neq k}\p\Big(\Big|\frac{1}{n}\sum_{i=1}^n \xi_{i}^{(j,k)}\Big| > t\Big)&\text{(Union bound)}\\ | &\le \sum_{j\neq k}\p\Big(\Big|\frac{1}{n}\sum_{i=1}^n \xi_{i}^{(j,k)}\Big| > t\Big)&\text{(Union bound)}\\ | ||
&\le \sum_{j\neq k}2e^{-\frac{nt^2}{2}}&\text{(Hoeffding: | &\le \sum_{j\neq k}2e^{-\frac{nt^2}{2}}&\text{(Hoeffding: [[guide:0251dfcdad#TH:hoeffding |Theorem]])}\\ | ||
&\le 2 d^2e^{-\frac{nt^2}{2}}. | &\le 2 d^2e^{-\frac{nt^2}{2}}. | ||
\end{align*} | \end{align*} | ||
Line 1,068: | Line 1,065: | ||
<math display="block"> | <math display="block"> | ||
\begin{equation*} | \begin{equation*} | ||
n\ge 2^{11}k^2\log(1/\delta)+2^{13}k^2\log(d)\,. | n\ge 2^{11}k^2\log(1/\delta)+2^{13}k^2\log(d)\,. | ||
\end{equation*} | \end{equation*} | ||
</math>}} | </math>}} | ||
For any <math>\theta \in \R^d</math>, <math>S\subset\{1, \ldots, d\}</math>, define <math>\theta_S</math> to be the vector with coordinates | For any <math>\theta \in \R^d</math>, <math>S\subset\{1, \ldots, d\}</math>, define <math>\theta_S</math> to be the vector with coordinates | ||
Line 1,112: | Line 1,109: | ||
\frac{|\X\theta_{S^c}|_2^2}{n} \ge |\theta_{S^c}|_2^2-\frac{|\theta_{S^c}|_1^2}{32k}\ge |\theta_{S^c}|_2^2-\frac{9|\theta_{S}|_1^2}{32k}\,, | \frac{|\X\theta_{S^c}|_2^2}{n} \ge |\theta_{S^c}|_2^2-\frac{|\theta_{S^c}|_1^2}{32k}\ge |\theta_{S^c}|_2^2-\frac{9|\theta_{S}|_1^2}{32k}\,, | ||
</math> | </math> | ||
where, in the last inequality, we used the cone condition | where, in the last inequality, we used the cone condition\eqref{EQ:conecond}.</li> | ||
<li>Finally, | <li>Finally, | ||
Line 1,118: | Line 1,115: | ||
2\Big|\theta_S^\top \frac{ \X^\top\X}{n}\theta_{S^c}\Big|\le \frac{2}{32k}|\theta_S|_1|\theta_{S^c}|_1\le \frac{6}{32k}|\theta_S|_1^2. | 2\Big|\theta_S^\top \frac{ \X^\top\X}{n}\theta_{S^c}\Big|\le \frac{2}{32k}|\theta_S|_1|\theta_{S^c}|_1\le \frac{6}{32k}|\theta_S|_1^2. | ||
</math> | </math> | ||
where, in the last inequality, we used the cone condition | where, in the last inequality, we used the cone condition\eqref{EQ:conecond}.</li> | ||
</ul> | </ul> | ||
Observe now that it follows from the Cauchy-Schwarz inequality that | Observe now that it follows from the Cauchy-Schwarz inequality that | ||
Line 1,129: | Line 1,126: | ||
<math display="block"> | <math display="block"> | ||
\begin{equation*} | \begin{equation*} | ||
\frac{|\X\theta|_2^2}{n} \ge |\theta_S|_2^2 +|\theta_{S^c}|_2^2- \frac{16|S|}{32k}|\theta_S|_2^2 \ge \frac12|\theta|_2^2. | \frac{|\X\theta|_2^2}{n} \ge |\theta_S|_2^2 +|\theta_{S^c}|_2^2- \frac{16|S|}{32k}|\theta_S|_2^2 \ge \frac12|\theta|_2^2. | ||
\end{equation*} | \end{equation*} | ||
</math>}} | </math>}} | ||
====Fast rate for the Lasso==== | ====Fast rate for the Lasso==== | ||
{{proofcard|Theorem|TH:lasso_fast|Fix <math>n \ge 2</math>. Assume that the linear model | |||
{{proofcard|Theorem|TH:lasso_fast|Fix <math>n \ge 2</math>. Assume that the linear model\eqref{EQ:regmod_matrix} holds where <math>\eps\sim \sg_n(\sigma^2)</math>. Moreover, assume that <math>|\theta^*|_0\le k</math> and that <math>\X</math> satisfies assumption <math>\textsf{INC$(k)$}</math>. Then the Lasso estimator <math>\thetalasso</math> with regularization parameter defined by | |||
<math display="block"> | <math display="block"> | ||
Line 1,165: | Line 1,163: | ||
|\X\thetalasso-\X\theta^*|_2^2+n\tau|\thetalasso-\theta^*|_1 \le2\eps^\top\X(\thetalasso-\theta^*) +n\tau|\thetalasso-\theta^*|_1+ 2n\tau|\theta^*|_1- 2n\tau|\thetalasso|_1 \,. | |\X\thetalasso-\X\theta^*|_2^2+n\tau|\thetalasso-\theta^*|_1 \le2\eps^\top\X(\thetalasso-\theta^*) +n\tau|\thetalasso-\theta^*|_1+ 2n\tau|\theta^*|_1- 2n\tau|\thetalasso|_1 \,. | ||
</math> | </math> | ||
Applying | Applying Höolder's inequality and using the same steps as in the proof of [[#TH:lasso_slow |Theorem]], we get that with probability <math>1-\delta</math>, we get | ||
<math display="block"> | <math display="block"> | ||
Line 1,193: | Line 1,191: | ||
\end{equation} | \end{equation} | ||
</math> | </math> | ||
so that <math>\theta=\thetalasso-\theta^*</math> satisfies the cone condition | so that <math>\theta=\thetalasso-\theta^*</math> satisfies the cone condition\eqref{EQ:conecond}. | ||
Using now the Cauchy-Schwarz inequality and | Using now the Cauchy-Schwarz inequality and [[#lem:inc |Lemma]] respectively, we get, since <math>|S|\le k</math>, | ||
<math display="block"> | <math display="block"> | ||
|\thetalasso_S-\theta^*|_1 \le \sqrt{|S|}|\thetalasso_S-\theta^*|_2 \le \sqrt{|S|}|\thetalasso-\theta^*|_2 \le\sqrt{\frac{2k}{n}}|\X\thetalasso-\X\theta^*|_2\,. | |\thetalasso_S-\theta^*|_1 \le \sqrt{|S|}|\thetalasso_S-\theta^*|_2 \le \sqrt{|S|}|\thetalasso-\theta^*|_2 \le\sqrt{\frac{2k}{n}}|\X\thetalasso-\X\theta^*|_2\,. | ||
</math> | </math> | ||
Combining this result with | Combining this result with\eqref{EQ:pr:lassofast1}, we find | ||
<math display="block"> | <math display="block"> | ||
Line 1,205: | Line 1,203: | ||
</math> | </math> | ||
This concludes the proof of the bound on the MSE. | This concludes the proof of the bound on the MSE. | ||
To prove | To prove\eqref{EQ:bornel2lasso}, we use [[#lem:inc |Lemma]] once again to get | ||
<math display="block"> | <math display="block"> | ||
\begin{equation*} | \begin{equation*} | ||
|\thetalasso -\theta^*|_2^2\le 2\MSE(\X\thetalasso)\le 64k\tau^2. | |\thetalasso -\theta^*|_2^2\le 2\MSE(\X\thetalasso)\le 64k\tau^2. | ||
\end{equation*} | \end{equation*} | ||
</math>}} | </math>}} | ||
Note that all we required for the proof was not really incoherence but the conclusion of | Note that all we required for the proof was not really incoherence but the conclusion of [[#lem:inc |Lemma]]: | ||
<math display="block"> | <math display="block"> | ||
Line 1,225: | Line 1,223: | ||
\cC_S=\big\{ |\theta_{S^c}|_1 \le 3|\theta_S|_1 \big\}\,. | \cC_S=\big\{ |\theta_{S^c}|_1 \le 3|\theta_S|_1 \big\}\,. | ||
</math> | </math> | ||
Condition | Condition\eqref{EQ:REcond} is sometimes called ''restricted eigenvalue (RE) condition''. Its name comes from the following observation. Note that all <math>k</math>-sparse vectors <math>\theta</math> are in a cone <math>\cC_{S}</math> with <math>|S|\le k</math> so that the RE condition implies that the smallest eigenvalue of <math>\X_S</math> satisfies | ||
<math>\lambda_\textrm{min}(\X_S)\ge n\kappa</math> for all <math>S</math> such that <math>|S|\le k</math>. Clearly, the RE condition is weaker than incoherence and it can actually be shown that a design matrix <math>\X</math> of | <math>\lambda_\textrm{min}(\X_S)\ge n\kappa</math> for all <math>S</math> such that <math>|S|\le k</math>. Clearly, the RE condition is weaker than incoherence and it can actually be shown that a design matrix <math>\X</math> of i.i.d Rademacher random variables satisfies the RE conditions as soon as <math>n\ge Ck\log(d)</math> with positive probability. | ||
===The Slope estimator=== | ===The Slope estimator=== | ||
We noticed that the BIC estimator can actually obtain a rate of <math> k\log(ed/k) </math>, where <math> k = |\theta^\ast|_0 </math>, while the LASSO only achieves <math> k \log(ed) </math>. | We noticed that the BIC estimator can actually obtain a rate of <math> k\log(ed/k) </math>, where <math> k = |\theta^\ast|_0 </math>, while the LASSO only achieves <math> k \log(ed) </math>. | ||
This begs the question whether the same rate can be achieved by an efficiently computable estimator. | This begs the question whether the same rate can be achieved by an efficiently computable estimator. | ||
The answer is yes, and the estimator achieving this is a slight modification of the LASSO, where the entries in the <math> \ell_1 </math>-norm are weighted differently according to their size in order to get rid of a slight inefficiency in our bounds. | The answer is yes, and the estimator achieving this is a slight modification of the LASSO, where the entries in the <math> \ell_1 </math>-norm are weighted differently according to their size in order to get rid of a slight inefficiency in our bounds. | ||
{{defncard|label=Slope | {{defncard|label=Slope estimator|id=| Let <math> \lambda = (\lambda_1, \dots, \lambda_d) </math> be a non-increasing sequence of positive real numbers, <math> \lambda_1 \geq \lambda_2 \geq \dots \geq \lambda_d > 0 </math>. | ||
For <math> \theta = (\theta_1, \dots, \theta_d) \in \R^d </math>, let <math> (\theta^\ast_1, \dots, \theta^\ast_d) </math> be a non-increasing rearrangement of the modulus of the entries, <math> |\theta_1|, \dots, |\theta_d| </math>. | For <math> \theta = (\theta_1, \dots, \theta_d) \in \R^d </math>, let <math> (\theta^\ast_1, \dots, \theta^\ast_d) </math> be a non-increasing rearrangement of the modulus of the entries, <math> |\theta_1|, \dots, |\theta_d| </math>. | ||
We define the ''sorted <math> \ell_1 </math> norm of <math> \theta </math>'' as | We define the ''sorted <math> \ell_1 </math> norm of <math> \theta </math>'' as | ||
Line 1,278: | Line 1,276: | ||
\end{equation} | \end{equation} | ||
</math> | </math> | ||
|We consider the moment generating function to apply a Chernoff bound. | |We consider the moment generating function to apply a Chernoff bound. Use Jensen's inequality on the sum to get | ||
<math display="block"> | <math display="block"> | ||
\begin{align*} | \begin{align*} | ||
\E \exp \left( \frac{3}{8 k \sigma^2} \sum_{j=1}^{k} (g^\ast_j)^2 \right) | \E \exp \left( \frac{3}{8 k \sigma^2} \sum_{j=1}^{k} (g^\ast_j)^2 \right) | ||
\leq {} & \frac{1}{k} \sum_{j=1}^{k} \E \exp \left( \frac{3 (g_j^\ast)^2}{8 \sigma^2} \right)\\ | \leq {} & \frac{1}{k} \sum_{j=1}^{k} \E \exp \left( \frac{3 (g_j^\ast)^2}{8 \sigma^2} \right)\\ | ||
Line 1,292: | Line 1,289: | ||
where we used the bound on the moment generating function of the <math> \chi^2 </math> distribution from Problem [[guide:0251dfcdad#EXO:chi2 |Sub-Gaussian Random Variables]] to get that <math> \E[\exp(3g^2/8)] = 2 </math> for <math> g \sim \cN(0,1) </math>. | where we used the bound on the moment generating function of the <math> \chi^2 </math> distribution from Problem [[guide:0251dfcdad#EXO:chi2 |Sub-Gaussian Random Variables]] to get that <math> \E[\exp(3g^2/8)] = 2 </math> for <math> g \sim \cN(0,1) </math>. | ||
The rest follows from a Chernoff bound.}} | The rest follows from a Chernoff bound.}} | ||
{{proofcard|Lemma|lem:b|Define <math>[d]:=\{1, \ldots, d\}</math>. Under the same assumptions as in | {{proofcard|Lemma|lem:b|Define <math>[d]:=\{1, \ldots, d\}</math>. Under the same assumptions as in [[#lem:a |Lemma]], | ||
<math display="block"> | <math display="block"> | ||
Line 1,308: | Line 1,305: | ||
\end{equation} | \end{equation} | ||
</math> | </math> | ||
Applying | Applying [[#lem:a |Lemma]] yields | ||
<math display="block"> | <math display="block"> | ||
Line 1,340: | Line 1,337: | ||
</math> | </math> | ||
for <math> \delta \leq 1/2 </math>.}} | for <math> \delta \leq 1/2 </math>.}} | ||
{{proofcard|Theorem|thm-1|Fix <math>n \ge 2</math>. Assume that the linear model | {{proofcard|Theorem|thm-1|Fix <math>n \ge 2</math>. Assume that the linear model\eqref{EQ:regmod_matrix} holds where <math>\eps\sim \cN_n(0,\sigma^2 I_n)</math>. Moreover, assume that <math>|\theta^*|_0\le k</math> and that <math>\X</math> satisfies assumption <math>\textsf{INC$(k')$}</math> with <math> k' \geq 4k \log(2de/k) </math>. Then the Slope estimator <math>\thetaslope</math> with regularization parameter defined by | ||
<math display="block"> | <math display="block"> | ||
Line 1,387: | Line 1,384: | ||
By | By [[#lem:b |Lemma]], we can estimate | ||
<math display="block"> | <math display="block"> | ||
Line 1,436: | Line 1,433: | ||
We can now repeat the incoherence arguments from | We can now repeat the incoherence arguments from [[#lem:inc |Lemma]], with <math> S </math> being the <math> k </math> largest entries of <math> |u| </math>, to get the same conclusion under the restriction <math> \textsf{INC}(k') </math>. | ||
First, by exactly the same argument as in | First, by exactly the same argument as in [[#lem:inc |Lemma]], we have | ||
<math display="block"> | <math display="block"> | ||
Line 1,513: | Line 1,510: | ||
\begin{equation} | \begin{equation} | ||
\label{eq:z} | \label{eq:z} | ||
|u|_2^2 \leq 2^{13} \frac{k}{n} \sigma^2 \log(2de/k) \log(1/\delta). | |u|_2^2 \leq 2^{13} \frac{k}{n} \sigma^2 \log(2de/k) \log(1/\delta). | ||
\end{equation} | \end{equation} | ||
</math>}} | </math>}} | ||
==General references== | ==General references== | ||
{{cite arXiv|last1=Rigollet|first1=Philippe|last2=Hütter|first2=Jan-Christian|year=2023|title=High-Dimensional Statistics|eprint=2310.19244|class=math.ST}} | {{cite arXiv|last1=Rigollet|first1=Philippe|last2=Hütter|first2=Jan-Christian|year=2023|title=High-Dimensional Statistics|eprint=2310.19244|class=math.ST}} | ||
==Notes== | ==Notes== |
Revision as of 18:34, 21 May 2024
[math] \require{textmacros} \newcommand{\DS}{\displaystyle} \newcommand{\intbeta}{\lfloor \beta \rfloor} \newcommand{\cA}{\mathcal{A}} \newcommand{\cB}{\mathcal{B}} \newcommand{\cC}{\mathcal{C}} \newcommand{\cD}{\mathcal{D}} \newcommand{\cE}{\mathcal{E}} \newcommand{\cF}{\mathcal{F}} \newcommand{\cG}{\mathcal{G}} \newcommand{\cH}{\mathcal{H}} \newcommand{\cI}{\mathcal{I}} \newcommand{\cJ}{\mathcal{J}} \newcommand{\cK}{\mathcal{K}} \newcommand{\cL}{\mathcal{L}} \newcommand{\cM}{\mathcal{M}} \newcommand{\cN}{\mathcal{N}} \newcommand{\cO}{\mathcal{O}} \newcommand{\cP}{\mathcal{P}} \newcommand{\cQ}{\mathcal{Q}} \newcommand{\cS}{\mathcal{S}} \newcommand{\cT}{\mathcal{T}} \newcommand{\cU}{\mathcal{U}} \newcommand{\cV}{\mathcal{V}} \newcommand{\cX}{\mathcal{X}} \newcommand{\cY}{\mathcal{Y}} \newcommand{\cZ}{\mathcal{Z}} \newcommand{\sg}{\mathsf{subG}} \newcommand{\subE}{\mathsf{subE}} \newcommand{\bA}{\mathbf{A}} \newcommand{\bB}{\mathbf{B}} \newcommand{\bC}{\mathbf{C}} \newcommand{\bD}{\mathbf{D}} \newcommand{\bE}{\mathbf{E}} \newcommand{\bF}{\mathbf{F}} \newcommand{\bG}{\mathbf{G}} \newcommand{\bH}{\mathbf{H}} \newcommand{\bI}{\mathbf{I}} \newcommand{\bJ}{\mathbf{J}} \newcommand{\bK}{\mathbf{K}} \newcommand{\bM}{\mathbf{M}} \newcommand{\bN}{\mathbf{N}} \newcommand{\bO}{\mathbf{O}} \newcommand{\bP}{\mathbf{P}} \newcommand{\bp}{\mathbf{p}} \newcommand{\bQ}{\mathbf{Q}} \newcommand{\bS}{\mathbf{S}} \newcommand{\bT}{\mathbf{T}} \newcommand{\bU}{\mathbf{U}} \newcommand{\bV}{\mathbf{V}} \newcommand{\bX}{\mathbf{X}} \newcommand{\bY}{\mathbf{Y}} \newcommand{\bZ}{\mathbf{Z}} \newcommand{\bflambda}{\boldsymbol{\lambda}} \newcommand{\bftheta}{\boldsymbol{\theta}} \newcommand{\bfg}{\boldsymbol{g}} \newcommand{\bfy}{\boldsymbol{y}} \def\thetaphat{\hat{\bftheta}_\bp} \def\bflam{\boldsymbol{\lambda}} \def\Lam{\Lambda} \def\lam{\lambda} \def\bfpi{\boldsymbol{\pi}} \def\bfz{\boldsymbol{z}} \def\bfw{\boldsymbol{w}} \def\bfeta{\boldsymbol{\eta}} \newcommand{\R}{\mathrm{ I}\kern-0.18em\mathrm{ R}} \newcommand{\h}{\mathrm{ I}\kern-0.18em\mathrm{ H}} \newcommand{\K}{\mathrm{ I}\kern-0.18em\mathrm{ K}} \newcommand{\p}{\mathrm{ I}\kern-0.18em\mathrm{ P}} \newcommand{\E}{\mathrm{ I}\kern-0.18em\mathrm{ E}} %\newcommand{\Z}{\mathrm{ Z}\kern-0.26em\mathrm{ Z}} \newcommand{\1}{\mathrm{ 1}\kern-0.24em\mathrm{ I}} \newcommand{\N}{\mathrm{ I}\kern-0.18em\mathrm{ N}} \newcommand{\field}[1]{\mathbb{#1}} \newcommand{\q}{\field{Q}} \newcommand{\Z}{\field{Z}} \newcommand{\X}{\field{X}} \newcommand{\Y}{\field{Y}} \newcommand{\bbS}{\field{S}} \newcommand{\n}{\mathcal{N}} \newcommand{\x}{\mathcal{X}} \newcommand{\pp}{{\sf p}} \newcommand{\hh}{{\sf h}} \newcommand{\ff}{{\sf f}} \newcommand{\Bern}{\mathsf{Ber}} \newcommand{\Bin}{\mathsf{Bin}} \newcommand{\Lap}{\mathsf{Lap}} \newcommand{\tr}{\mathsf{Tr}} \newcommand{\phin}{\varphi_n} \newcommand{\phinb}{\overline \varphi_n(t)} \newcommand{\pn}{\p_{\kern-0.25em n}} \newcommand{\pnm}{\p_{\kern-0.25em n,m}} \newcommand{\psubm}{\p_{\kern-0.25em m}} \newcommand{\psubp}{\p_{\kern-0.25em p}} \newcommand{\cfi}{\cF_{\kern-0.25em \infty}} \newcommand{\e}{\mathrm{e}} \newcommand{\ic}{\mathrm{i}} \newcommand{\Leb}{\mathrm{Leb}_d} \newcommand{\Var}{\mathrm{Var}} \newcommand{\ddelta}{d_{\symdiffsmall}} \newcommand{\dsubh}{d_H} \newcommand{\indep}{\perp\kern-0.95em{\perp}} \newcommand{\supp}{\mathop{\mathrm{supp}}} \newcommand{\rank}{\mathop{\mathrm{rank}}} \newcommand{\vol}{\mathop{\mathrm{vol}}} \newcommand{\conv}{\mathop{\mathrm{conv}}} \newcommand{\card}{\mathop{\mathrm{card}}} \newcommand{\argmin}{\mathop{\mathrm{argmin}}} \newcommand{\argmax}{\mathop{\mathrm{argmax}}} \newcommand{\ud}{\mathrm{d}} \newcommand{\var}{\mathrm{var}} \newcommand{\re}{\mathrm{Re}} \newcommand{\MSE}{\mathsf{MSE}} \newcommand{\im}{\mathrm{Im}} \newcommand{\epr}{\hfill\hbox{\hskip 4pt\vrule width 5pt height 6pt depth 1.5pt}\vspace{0.5cm}\par} \newcommand{\bi}[1]{^{(#1)}} \newcommand{\eps}{\varepsilon} \newcommand{\Deq}{\stackrel{\mathcal{D}}{=}} \newcommand{\ubar}{\underbar} \newcommand{\Kbeta}{K_{\hspace{-0.3mm} \beta}} \newcommand{\crzero}[1]{\overset{r_0}{\underset{#1}{\longleftrightarrow}}} \newcommand{\hint}[1]{\texttt{[Hint:#1]}} \newcommand{\vp}{\vspace{.25cm}} \newcommand{\vm}{\vspace{.5cm}} \newcommand{\vg}{\vspace{1cm}} \newcommand{\vgneg}{\vspace{-1cm}} \newcommand{\vneg}{\vspace{-.5cm}} \newcommand{\vpneg}{\vspace{-.25cm}} \newcommand{\tp}{\ptsize{10}} \newcommand{\douzp}{\ptsize{12}} \newcommand{\np}{\ptsize{9}} \newcommand{\hp}{\ptsize{8}} \newcommand{\red}{\color{red}} \newcommand{\ndpr}[1]{{\textsf{\red[#1]}}} \newcommand\iid{i.i.d\@ifnextchar.{}{.\@\xspace} } \newcommand\MoveEqLeft[1][2]{\kern #1em & \kern -#1em} \newcommand{\leadeq}[2][4]{\MoveEqLeft[#1] #2 \nonumber} \newcommand{\leadeqnum}[2][4]{\MoveEqLeft[#1] #2} \newcommand\independent{\protect\mathpalette{\protect\independenT}{\perp}} \def\independenT#1#2{\mathrel{\rlap{$#1#2$}\mkern2mu{#1#2}}} \newcommand{\MIT}[1]{{\color{MITred} #1}} \newcommand{\dHyp}{\{-1,1\}^d} \newcommand{\thetahard}{\hat \theta^{hrd}} \newcommand{\thetasoft}{\hat \theta^{sft}} \newcommand{\thetabic}{\hat \theta^{bic}} \newcommand{\thetalasso}{\hat \theta^{\cL}} \newcommand{\thetaslope}{\hat \theta^{\cS}} \newcommand{\thetahard}{\hat \theta^{hrd}} \newcommand{\thetasoft}{\hat \theta^{sft}} \newcommand{\thetabic}{\hat \theta^{bic}} \newcommand{\thetalasso}{\hat \theta^{\cL}} \newcommand{\thetaslope}{\hat \theta^{\cS}} \newcommand{\thetals}{\hat \theta^{ls}} \newcommand{\thetalsm}{\tilde \theta^{ls_X}} \newcommand{\thetaridge}{\hat\theta^{\mathrm{ridge}}_\tau} \newcommand{\thetalsK}{\hat \theta^{ls}_K} \newcommand{\muls}{\hat \mu^{ls}} \newcommand{\thetahard}{\hat \theta^{\textsc{hrd}}} \newcommand{\thetasoft}{\hat \theta^{\textsc{sft}}} \newcommand{\thetabic}{\hat \theta^{\textsc{bic}}} \newcommand{\thetalasso}{\hat \theta^{\cL}} \newcommand{\thetaslope}{\hat \theta^{\cS}} \newcommand{\thetals}{\hat \theta^{\textsc{ls}}} \newcommand{\thetalsm}{\tilde \theta^{\textsc{ls}}_X} \newcommand{\thetaridge}{\hat\theta^{\mathrm{ridge}}_\tau} \newcommand{\thetalsK}{\hat \theta^{\textsc{ls}}_K} \newcommand{\muls}{\hat \mu^{\textsc{ls}}} [/math]
In this chapter, we consider the following regression model:
where [math]\eps=(\eps_1, \ldots, \eps_n)^\top [/math] is sub-Gaussian with variance proxy [math]\sigma^2[/math] and such that [math]\E[\eps]=0[/math]. Our goal is to estimate the function [math]f[/math] under a linear assumption. Namely, we assume that [math]x \in \R^d[/math] and [math]f(x)=x^\top\theta^*[/math] for some unknown [math]\theta^* \in \R^d[/math].
Fixed design linear regression
Depending on the nature of the design points [math]X_1, \ldots, X_n[/math], we will favor a different measure of risk. In particular, we will focus either on fixed or random design.
Random design
The case of random design corresponds to the statistical learning setup. Let [math](X_1,Y_1), \ldots, (X_{n+1}, Y_{n+1})[/math] be [math]n+1[/math] i.i.d random couples. Given the pairs [math](X_1,Y_1), \ldots, (X_{n}, Y_{n})[/math], the goal is construct a function [math]\hat f_n[/math] such that [math]\hat f_n(X_{n+1})[/math] is a good predictor of [math]Y_{n+1}[/math]. Note that when [math]\hat f_n[/math] is constructed, [math]X_{n+1}[/math] is still unknown and we have to account for what value it is likely to take. Consider the following example from[1](Section3.2). The response variable [math]Y[/math] is the log-volume of a cancerous tumor, and the goal is to predict it based on [math]X \in \R^6[/math], a collection of variables that are easier to measure (age of patient, log-weight of prostate, ...). Here the goal is clearly to construct [math]f[/math] for prediction purposes. Indeed, we want to find an automatic mechanism that outputs a good prediction of the log-weight of the tumor given certain inputs for a new (unseen) patient.
A natural measure of performance here is the [math]L_2[/math]-risk employed in the introduction:
where [math]P_X[/math] denotes the marginal distribution of [math]X_{n+1}[/math]. It measures how good the prediction of [math]Y_{n+1}[/math] is in average over realizations of [math]X_{n+1}[/math]. In particular, it does not put much emphasis on values of [math]X_{n+1}[/math] that are not very likely to occur. Note that if the [math]\eps_i[/math] are random variables with variance [math]\sigma^2[/math], then one simply has [math]R(\hat f_n)=\sigma^2+\|\hat f_n -f\|^2_{L^2(P_X)}[/math]. Therefore, for random design, we will focus on the squared [math]L_2[/math] norm [math]\|\hat f_n -f\|^2_{L^2(P_X)}[/math] as a measure of accuracy. It measures how close [math]\hat f_n[/math] is to the unknown [math]f[/math] in average over realizations of [math]X_{n+1}[/math].
Fixed design
In fixed design, the points (or vectors) [math]X_1, \ldots, X_n[/math] are deterministic. To emphasize this fact, we use lowercase letters [math]x_1, \ldots, x_n[/math] to denote fixed design. Of course, we can always think of them as realizations of a random variable but the distinction between fixed and random design is deeper and significantly affects our measure of performance. Indeed, recall that for random design, we look at the performance in average over realizations of [math]X_{n+1}[/math]. Here, there is no such thing as a marginal distribution of [math]X_{n+1}[/math]. Rather, since the design points [math]x_1, \ldots, x_n[/math] are considered deterministic, our goal is to estimate [math]f[/math] only at these points. This problem is sometimes called denoising since our goal is to recover [math]f(x_1), \ldots, f(x_n)[/math] given noisy observations of these values. In many instances, fixed designs exhibit particular structures. A typical example is the regular design on [math][0,1][/math], given by [math]x_i=i/n[/math], [math]i=1, \ldots, n[/math]. Interpolation between these points is possible under smoothness assumptions. Note that in fixed design, we observe [math]\mu^*+\eps[/math], where [math]\mu^*=\big(f(x_1), \ldots, f(x_n)\big)^\top \in \R^n[/math] and [math]\eps=(\eps_1, \ldots, \eps_n)^\top \in \R^n[/math] is sub-Gaussian with variance proxy [math]\sigma^2[/math]. Instead of a functional estimation problem, it is often simpler to view this problem as a vector problem in [math]\R^n[/math]. This point of view will allow us to leverage the Euclidean geometry of [math]\R^n[/math]. In the case of fixed design, we will focus on the Mean Squared Error (MSE) as a measure of performance. It is defined by
Equivalently, if we view our problem as a vector problem, it is defined by
Often, the design vectors [math]x_1, \ldots, x_n \in \R^d[/math] are stored in an [math]n\times d[/math] design matrix [math]\X[/math], whose [math]j[/math]th row is given by [math]x_j^\top[/math]. With this notation, the linear regression model can be written as
where [math]Y=(Y_1, \ldots, Y_n)^\top[/math] and [math]\eps=(\eps_1, \ldots, \eps_n)^\top[/math]. Moreover,
A natural example of fixed design regression is image denoising. Assume that [math]\mu^*_i, i \in 1, \ldots, n[/math] is the grayscale value of pixel [math]i[/math] of an image. We do not get to observe the image [math]\mu^*[/math] but rather a noisy version of it [math]Y=\mu^*+\eps[/math]. Given a library of [math]d[/math] images [math]\{x_1, \ldots, x_d\}, x_j \in \R^{n}[/math], our goal is to recover the original image [math]\mu^*[/math] using linear combinations of the images [math]x_1, \ldots, x_d[/math]. This can be done fairly accurately (see Figure).
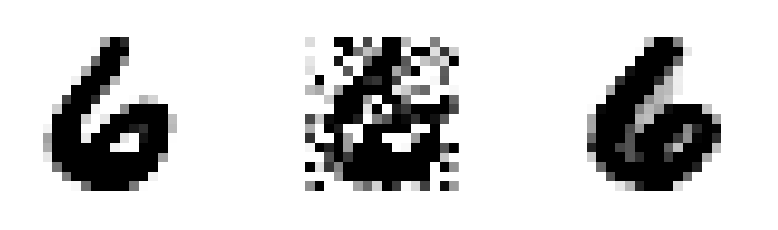
As we will see in RemarkLinear Regression Model, properly choosing the design also ensures that if [math]\MSE(\hat f)[/math] is small for some linear estimator [math]\hat f(x)=x^\top \hat \theta[/math], then [math]|\hat \theta -\theta^*|_2^2[/math] is also small.
Least squares estimators
Throughout this section, we consider the regression model\eqref{EQ:regmod_matrix} with fixed design.
Unconstrained least squares estimator
Define the (unconstrained) ’'least squares estimator [math]\thetals[/math] to be any vector such that
Note that we are interested in estimating [math]\X\theta^*[/math] and not [math]\theta^*[/math] itself, so by extension, we also call [math]\muls=\X\thetals[/math] least squares estimator. Observe that [math]\muls[/math] is the projection of [math]Y[/math] onto the column span of [math]\X[/math].
It is not hard to see that least squares estimators of [math]\theta^*[/math] and [math]\mu^*=\X\theta^*[/math] are maximum likelihood estimators when [math]\eps \sim \cN(0,\sigma^2I_n)[/math].
The least squares estimator [math]\muls=\X\thetals \in \R^n[/math] satisfies
The function [math]\theta \mapsto |Y-\X\theta|_2^2[/math] is convex so any of its minima satisfies
We are now going to prove our first result on the finite sample performance of the least squares estimator for fixed design.
Assume that the linear model\eqref{EQ:regmod_matrix} holds where [math]\eps\sim \sg_n(\sigma^2)[/math]. Then the least squares estimator [math]\thetals[/math] satisfies
Note that by definition
Constrained least squares estimator
Let [math]K\subset \R^d[/math] be a symmetric convex set. If we know ’'a priori that [math]\theta^* \in K[/math], we may prefer a constrained least squares estimator [math]\thetalsK[/math] defined by
The fundamental inequality\eqref{EQ:fund_ineq_ls} would still hold and the bounds on the MSE may be smaller. Indeed, \eqref{EQ:bound_ls_1} can be replaced by
where [math]K-K=\{x-y\,:\, x, y \in K\}[/math]. It is easy to see that if [math]K[/math] is symmetric ([math]x \in K \Leftrightarrow -x \in K[/math]) and convex, then [math]K-K=2K[/math] so that
where [math]\X K=\{\X\theta\,:\, \theta \in K\} \subset \R^n[/math]. This is a measure of the size (width) of [math]\X K[/math]. If [math]\eps\sim \cN(0,I_d)[/math], the expected value of the above supremum is actually called Gaussian width of [math]\X K[/math]. While [math]\eps[/math] is not Gaussian but sub-Gaussian here, similar properties will still hold.
[math]\ell_1[/math] constrained least squares
Assume here that [math]K=\cB_1[/math] is the unit [math]\ell_1[/math] ball of [math]\R^d[/math]. Recall that it is defined by
and it has exactly [math]2d[/math] vertices [math]\cV=\{e_1, -e_1, \ldots, e_d, -e_d\}[/math], where [math]e_j[/math] is the [math]j[/math]-th vector of the canonical basis of [math]\R^d[/math] and is defined by
It implies that the set [math]\X K=\{\X\theta, \theta \in K\} \subset \R^n[/math] is also a polytope with at most [math]2d[/math] vertices that are in the set [math]\X\cV=\{\X_1, -\X_1, \ldots, \X_d, -\X_d\}[/math] where [math]\X_j[/math] is the [math]j[/math]-th column of [math]\X[/math]. Indeed, [math]\X K[/math] is obtained by rescaling and embedding (resp. projecting) the polytope [math]K[/math] when [math]d \le n[/math] (resp., [math]d \ge n[/math]). Note that some columns of [math]\X[/math] might not be vertices of [math]\X K[/math] so that [math]\X \cV[/math] might be a strict superset of the set of vertices of [math]\X K[/math].
Let [math]K=\cB_1[/math] be the unit [math]\ell_1[/math] ball of [math]\R^d, d\ge 2[/math] and assume that [math]\theta^* \in \cB_1[/math]. Moreover, assume the conditions of Theorem and that the columns of [math]\X[/math] are normalized in such a way that [math]\max_j|\X_j|_2\le \sqrt{n}[/math]. Then the constrained least squares estimator [math]\thetals_{\cB_1}[/math] satisfies
From the considerations preceding the theorem, we get that
Note that the proof of Theorem also applies to [math]\thetals_{\cB_1}[/math] (exercise!) so that [math]\X \thetals_{\cB_1}[/math] benefits from the best of both rates,
This is called an elbow effect. The elbow takes place around [math]r\simeq\sqrt{n}[/math] (up to logarithmic terms).
[math]\ell_0[/math] constrained least squares
By an abuse of notation, we call the number of non-zero coefficients of a vector [math] \theta \in \R^d [/math] its [math]\ell_0[/math] norm (it is not actually a norm). It is denoted by
We call a vector [math]\theta[/math] with small" [math]\ell_0[/math] norm a ’'sparse vector. More precisely, if [math]|\theta|_0\le k[/math], we say that [math]\theta[/math] is a [math]k[/math]-sparse vector. We also call support of [math]\theta[/math] the set
so that [math]|\theta|_0=\card(\supp(\theta))=:|\supp(\theta)|[/math].
The [math]\ell_0[/math] terminology and notation comes from the fact that
By extension, denote by [math]\cB_0(k)[/math] the [math]\ell_0[/math] ball of [math]\R^d[/math], i.e., the set of [math]k[/math]-sparse vectors, defined by
In this section, our goal is to control the [math]\MSE[/math] of [math]\thetalsK[/math] when [math]K=\cB_0(k)[/math]. Note that computing [math]\thetals_{\cB_0(k)}[/math] essentially requires computing [math]\binom{d}{k}[/math] least squares estimators, which is an exponential number in [math]k[/math]. In practice this will be hard (or even impossible) but it is interesting to understand the statistical properties of this estimator and to use them as a benchmark.
Fix a positive integer [math]k \le d/2[/math]. Let [math]K=\cB_0(k)[/math] be set of [math]k[/math]-sparse vectors of [math]\R^d[/math] and assume that [math]\theta^*\in \cB_0(k)[/math]. Moreover, assume the conditions of Theorem. Then, for any [math]\delta \gt 0[/math], with probability [math]1-\delta[/math], it holds
We begin as in the proof of Theorem to get\eqref{EQ:bound_ls_1}:
How large is [math]\log\binom{d}{2k}[/math]? It turns out that it is not much larger than [math]k[/math].
For any integers [math]1\le k \le n[/math], it holds
Observe first that if [math]k=1[/math], since [math]n \ge 1[/math], it holds,
It immediately leads to the following corollary:
Under the assumptions of Theorem, for any [math]\delta \gt 0[/math], with probability at least [math]1-\delta[/math], it holds
Note that for any fixed [math]\delta[/math], there exits a constant [math]C_\delta \gt 0[/math] such that for any [math]n \ge 2k[/math], with high probability,
Comparing this result with Theorem with [math]r=k[/math], we see that the price to pay for not knowing the support of [math]\theta^*[/math] but only its size, is a logarithmic factor in the dimension [math]d[/math]. This result immediately leads to the following bound in expectation.
Under the assumptions of Theorem,
It follows from\eqref{EQ:prMSEl0HP} that for any [math]H\ge 0[/math],
The Gaussian Sequence Model
The Gaussian Sequence Model is a toy model that has received a lot of attention, mostly in the eighties. The main reason for its popularity is that it carries already most of the insight of nonparametric estimation. While the model looks very simple it allows to carry deep ideas that extend beyond its framework and in particular to the linear regression model that we are interested in. Unfortunately, we will only cover a small part of these ideas and the interested reader should definitely look at the excellent books by A. Tsybakov[3](Chapter3) and I. Johnstone[4]. The model is as follows:
where [math]\eps_1, \ldots, \eps_d[/math] are i.i.d [math]\cN(0,\sigma^2)[/math] random variables. Note that often, [math]d[/math] is taken equal to [math]\infty[/math] in this sequence model and we will also discuss this case. Its links to nonparametric estimation will become clearer in Chapter Misspecified Linear Models. The goal here is to estimate the unknown vector [math]\theta^*[/math].
The sub-Gaussian Sequence Model
Note first that the model\eqref{EQ:gsm} is a special case of the linear model with fixed design\eqref{EQ:regmod} with [math]n=d[/math] and [math]f(x_i)=x_i^\top \theta^*[/math], where [math]x_1, \ldots, x_n[/math] form the canonical basis [math] e_1, \dots, e_n [/math] of [math]\R^n[/math] and [math]\eps[/math] has a Gaussian distribution. Therefore, [math]n=d[/math] is both the dimension of the parameter [math]\theta[/math] and the number of observations and it looks like we have chosen to index this problem by [math]d[/math] rather than [math]n[/math] somewhat arbitrarily. We can bring [math]n[/math] back into the picture, by observing that this model encompasses slightly more general choices for the design matrix [math]\X[/math] as long as it satisfies the following assumption.
Assumption [math]\textsf{ORT}[/math]
The design matrix satisfies
where [math]I_d[/math] denotes the identity matrix of [math]\R^d[/math].
Assumption [math]\textsf{ORT}[/math] allows for cases where [math]d\le n[/math] but not [math]d \gt n[/math] (high dimensional case) because of obvious rank constraints. In particular, it means that the [math]d[/math] columns of [math]\X[/math] are orthogonal in [math]\R^n[/math] and all have norm [math]\sqrt{n}[/math]. Under this assumption, it follows from the linear regression model\eqref{EQ:regmod_matrix} that
where [math]\xi=(\xi_1, \ldots, \xi_d)\sim \sg_d(\sigma^2/n)[/math] (If [math]\eps[/math] is Gaussian, we even have [math]\xi \sim \cN_d(0, \sigma^2/n)[/math]). As a result, under the Assumption [math]\textsf{ORT}[/math], when [math]\xi[/math] is Gaussian, the linear regression model\eqref{EQ:regmod_matrix} is equivalent to the Gaussian Sequence Model\eqref{EQ:gsm} up to a transformation of the data [math]Y[/math] and a rescaling of the variance. Moreover, for any estimator [math]\hat \theta \in \R^d[/math], under [math]\textsf{ORT}[/math], it follows from\eqref{EQ:mse_matrix} that
Furthermore, for any [math]\theta \in \R^d[/math], the Assumption [math]\textsf{ORT}[/math] yields,
where [math]Q[/math] is a constant that does not depend on [math]\theta[/math] and is defined by
This implies in particular that the least squares estimator [math]\thetals[/math] is equal to [math]y[/math]. \bigskip We introduce a sightly more general model called sub-Gaussian sequence model:
where [math]\xi\sim \sg_d(\sigma^2/n)[/math]. In this section, we can actually completely ‘`forget" about our original model\eqref{EQ:regmod_matrix}. In particular we can define this model independently of Assumption [math]\textsf{ORT}[/math] and thus for any values of [math]n[/math] and [math]d[/math]. The sub-Gaussian sequence model and the Gaussian sequence model are called ’'direct (observation) problems as opposed to inverse problems where the goal is to estimate the parameter [math]\theta^*[/math] only from noisy observations of its image through an operator. The linear regression model is one such inverse problem where the matrix [math]\X[/math] plays the role of a linear operator. However, in these notes, we never try to invert the operator. See [5] for an interesting survey on the statistical theory of inverse problems.
Sparsity adaptive thresholding estimators
If we knew a priori that [math]\theta[/math] was [math]k[/math]-sparse, we could directly employ Corollary to obtain that with probability at least [math]1-\delta[/math], we have
As we will see, the Assumption [math]\textsf{ORT}[/math] gives us the luxury to not know [math]k[/math] and yet adapt to its value. Adaptation means that we can construct an estimator that does not require the knowledge of [math]k[/math] (the smallest such that [math]|\theta^*|_0\le k[/math]) and yet perform as well as [math]\thetals_{\cB_0(k)}[/math], up to a multiplicative constant. Let us begin with some heuristic considerations to gain some intuition. Assume the sub-Gaussian sequence model\eqref{EQ:sGSM}. If nothing is known about [math]\theta^*[/math] it is natural to estimate it using the least squares estimator [math]\thetals=y[/math]. In this case,
where the last inequality holds with probability at least [math]1-\delta[/math]. This is actually what we are looking for if [math]k=Cd[/math] for some positive constant [math]C\le 1[/math]. The problem with this approach is that it does not use the fact that [math]k[/math] may be much smaller than [math]d[/math], which happens when [math]\theta^*[/math] has many zero coordinates. If [math]\theta^*_j=0[/math], then, [math]y_j=\xi_j[/math], which is a sub-Gaussian random variable with variance proxy [math]\sigma^2/n[/math]. In particular, we know from Lemma that with probability at least [math]1-\delta[/math],
The consequences of this inequality are interesting. One the one hand, if we observe [math]|y_j|\gg \tau[/math] , then it must correspond to [math]\theta_j^*\neq 0[/math]. On the other hand, if [math]|y_j| \le \tau[/math] is smaller, then, [math]\theta_j^*[/math] cannot be very large. In particular, by the triangle inequality, [math]|\theta^*_j| \le |y_j|+|\xi_j| \le 2\tau[/math]. Therefore, we loose at most [math]2\tau[/math] by choosing [math]\hat \theta_j=0[/math]. It leads us to consider the following estimator.
The hard thresholding estimator with threshold [math]2\tau \gt 0[/math] is denoted by [math]\thetahard[/math] and has coordinates
From our above consideration, we are tempted to choose [math]\tau[/math] as in\eqref{EQ:tau1}. Yet, this threshold is not large enough. Indeed, we need to choose [math]\tau[/math] such that [math]|\xi_j|\le \tau[/math] simultaneously for all [math]j[/math]. This can be done using a maximal inequality. Namely, Theorem ensures that with probability at least [math]1-\delta[/math],
It yields the following theorem.
Consider the linear regression model\eqref{EQ:regmod_matrix} under the Assumption [math]\textsf{ORT}[/math] or, equivalenty, the sub-Gaussian sequence model\eqref{EQ:sGSM}. Then the hard thresholding estimator [math]\thetahard[/math] with threshold
- If [math]|\theta^*|_0=k[/math],
[[math]] \MSE(\X\thetahard)=|\thetahard-\theta^*|_2^2\lesssim \sigma^2\frac{k\log (2d/\delta)}{n}\,. [[/math]]
- if [math]\min_{j \in \supp(\theta^*)}|\theta^*_j| \gt 3\tau[/math], then
[[math]] \supp(\thetahard)=\supp(\theta^*)\,. [[/math]]
Define the event
Similar results can be obtained for the soft thresholding estimator [math]\thetasoft[/math] defined by
In short, we can write
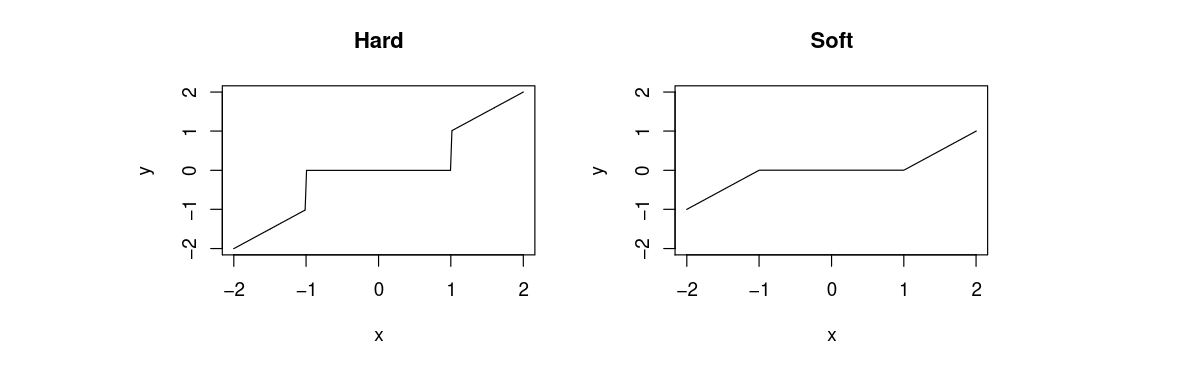
High-dimensional linear regression
The BIC and Lasso estimators
It can be shown (see ProblemLinear Regression Model) that the hard and soft thresholding estimators are solutions of the following penalized empirical risk minimization problems:
In view of\eqref{EQ:ERM_sGSM}, under the Assumption [math]\textsf{ORT}[/math], the above variational definitions can be written as
When the Assumption [math]\textsf{ORT}[/math] is not satisfied, they no longer correspond to thresholding estimators but can still be defined as above. We change the constant in the threshold parameters for future convenience.
Fix [math]\tau \gt 0[/math] and assume the linear regression model\eqref{EQ:regmod_matrix}. The BIC[Notes 2] estimator of [math]\theta^*[/math] is defined by any [math]\thetabic[/math] such that
By contrast, computing the Lasso estimator is a convex problem and there exist many efficient algorithms to solve it. We will not describe this optimization problem in detail but only highlight a few of the best known algorithms:
- Probably the most popular method among statisticians relies on coordinate gradient descent. It is implemented in the [math]\textsf{glmnet}[/math] package in [math]\textsf{R}[/math] [6],
- An interesting method called LARS [7] computes the entire regularization path, i.e., the solution of the convex problem for all values of [math]\tau[/math]. It relies on the fact that, as a function of [math]\tau[/math], the solution [math]\thetalasso[/math] is a piecewise linear function (with values in [math]\R^d[/math]). Yet this method proved to be too slow for very large problems and has been replaced by glmnet which computes solutions for values of [math]\tau[/math] on a grid much faster.
- The optimization community has made interesting contributions to this field by using proximal methods to solve this problem. These methods exploit the structure of the objective function which is of the form smooth (sum of squares) + simple ([math]\ell_1[/math] norm). A good entry point to this literature is perhaps the FISTA algorithm [8].
- Recently there has been a lot of interest around this objective for very large [math]d[/math] and very large [math]n[/math]. In this case, even computing [math]|Y-\X\theta|_2^2 [/math] may be computationally expensive and solutions based on stochastic gradient descent are flourishing.
Note that by Lagrange duality, computing [math]\thetalasso[/math] is equivalent to solving an [math]\ell_1[/math] constrained least squares. Nevertheless, the radius of the [math]\ell_1[/math] constraint is unknown. In general, it is hard to relate Lagrange multipliers to the size constraints. The name ‘`Lasso" was originally given to the constrained version this estimator in the original paper of Robert Tibshirani [9].
Analysis of the BIC estimator
While computationally hard to implement, the BIC estimator gives us a good benchmark for sparse estimation. Its performance is similar to that of [math]\thetahard[/math] but without requiring the Assumption [math]\textsf{ORT}[/math].
Assume that the linear model\eqref{EQ:regmod_matrix} holds, where [math]\eps \sim \sg_n(\sigma^2)[/math] and that [math]|\theta^*|_0\ge 1[/math]. Then, the BIC estimator [math]\thetabic[/math] with regularization parameter
We begin as usual by noting that
It follows from Theorem that [math]\thetabic[/math] ’'adapts to the unknown sparsity of [math]\theta^*[/math], just like [math]\thetahard[/math]. Moreover, this holds under no assumption on the design matrix [math]\X[/math].
Analysis of the Lasso estimator
Slow rate for the Lasso estimator
The properties of the BIC estimator are quite impressive. It shows that under no assumption on [math]\X[/math], one can mimic two oracles: (i) the oracle that knows the support of [math]\theta^*[/math] (and computes least squares on this support), up to a [math]\log(ed)[/math] term and (ii) the oracle that knows the sparsity [math]|\theta^*|_0[/math] of [math]\theta^*[/math], up to a smaller logarithmic term [math]\log(ed/|\theta^*|_0)[/math], which is subsumed by [math]\log(ed)[/math]. Actually, the [math] \log(ed) [/math] can even be improved to [math] \log(ed/|\theta^\ast|_0) [/math] by using a modified BIC estimator (see ProblemLinear Regression Model). The Lasso estimator is a bit more difficult to analyze because, by construction, it should more naturally adapt to the unknown [math]\ell_1[/math]-norm of [math]\theta^*[/math]. This can be easily shown as in the next theorem, analogous to Theorem.
Assume that the linear model\eqref{EQ:regmod_matrix} holds where [math]\eps\sim\sg_n(\sigma^2)[/math]. Moreover, assume that the columns of [math]\X[/math] are normalized in such a way that [math]\max_j|\X_j|_2\le \sqrt{n}[/math]. Then, the Lasso estimator [math]\thetalasso[/math] with regularization parameter
From the definition of [math]\thetalasso[/math], it holds
Notice that the regularization parameter\eqref{EQ:taulasso} depends on the confidence level [math]\delta[/math]. This is not the case for the BIC estimator (see\eqref{EQ:taubic}).
The rate in Theorem is of order [math]\sqrt{(\log d)/n}[/math] (slow rate), which is much slower than the rate of order [math](\log d)/n[/math] (fast rate) for the BIC estimator. Hereafter, we show that fast rates can also be achieved by the computationally efficient Lasso estimator, but at the cost of a much stronger condition on the design matrix [math]\X[/math].
Incoherence
\begin{assumption}{[math]\mathsf{INC}(k)[/math]} We say that the design matrix [math]\X[/math] has incoherence [math]k[/math] for some integer [math]k \gt 0[/math] if
where the [math]|A|_\infty[/math] denotes the largest element of [math]A[/math] in absolute value. Equivalently,
- For all [math]j=1, \ldots, d[/math],
[[math]] \left|\frac{|\X_j|_2^2}{n}-1\right|\le \frac{1}{32k}\,. [[/math]]
- For all [math]1\le i,j\le d[/math], [math]i\neq j[/math], we have
[[math]] \frac{|\X_i^\top \X_j|}{n} \le \frac{1}{32k}\,. [[/math]]
\end{assumption} Note that Assumption [math]\textsf{ORT}[/math] arises as the limiting case of [math]\mathsf{INC}(k)[/math] as [math]k \to \infty[/math]. However, while Assumption [math]\textsf{ORT}[/math] requires [math]d \le n[/math], here we may have [math]d \gg n[/math] as illustrated in Proposition below. To that end, we simply have to show that there exists a matrix that satisfies [math]\mathsf{INC}(k)[/math] even for [math]d \gt n[/math]. We resort to the probabilistic method [10]. The idea of this method is that if we can find a probability measure that assigns positive probability to objects that satisfy a certain property, then there must exist objects that satisfy said property. In our case, we consider the following probability distribution on random matrices with entries in [math]\{\pm 1\}[/math]. Let the design matrix [math]\X[/math] have entries that are i.i.d Rademacher [math](\pm 1)[/math] random variables. We are going to show that most realizations of this random matrix satisfy Assumption[math]\mathsf{INC}(k)[/math] for large enough [math]n[/math].
Let [math]\X \in \R^{n\times d}[/math] be a random matrix with entries [math]X_{ij}, i=1,\ldots, n, j=1, \ldots, d[/math], that are i.i.d Rademacher [math](\pm 1)[/math] random variables. Then, [math]\X[/math] has incoherence [math]k[/math] with probability [math]1-\delta[/math] as soon as
Let [math]\eps_{ij} \in \{-1,1\}[/math] denote the Rademacher random variable that is on the [math]i[/math]th row and [math]j[/math]th column of [math]\X[/math]. Note first that the [math]j[/math]th diagonal entries of [math]\X^\top\X/n[/math] are given by
For any [math]\theta \in \R^d[/math], [math]S\subset\{1, \ldots, d\}[/math], define [math]\theta_S[/math] to be the vector with coordinates
In particular [math]|\theta|_1=|\theta_S|_1+|\theta_{S^c}|_1[/math]. The following lemma holds
Fix a positive integer [math]k \le d[/math] and assume that [math]\X[/math] satisfies assumption [math]\mathsf{INC}(k)[/math]. Then, for any [math]S \in \{1, \ldots, d\}[/math] such that [math]|S|\le k[/math] and any [math]\theta \in \R^d[/math] that satisfies the cone condition
We have
- First, if follows from the incoherence condition that
[[math]] \frac{|\X\theta_S|_2^2}{n}=\theta_S^\top \frac{\X^\top\X}{n}\theta_S =|\theta_S|_2^2+\theta_S^\top \left(\frac{\X^\top\X}{n} - I_d\right)\theta_S \ge |\theta_S|_2^2-\frac{|\theta_S|_1^2}{32k}\,, [[/math]]
- Similarly,
[[math]] \frac{|\X\theta_{S^c}|_2^2}{n} \ge |\theta_{S^c}|_2^2-\frac{|\theta_{S^c}|_1^2}{32k}\ge |\theta_{S^c}|_2^2-\frac{9|\theta_{S}|_1^2}{32k}\,, [[/math]]where, in the last inequality, we used the cone condition\eqref{EQ:conecond}.
- Finally,
[[math]] 2\Big|\theta_S^\top \frac{ \X^\top\X}{n}\theta_{S^c}\Big|\le \frac{2}{32k}|\theta_S|_1|\theta_{S^c}|_1\le \frac{6}{32k}|\theta_S|_1^2. [[/math]]where, in the last inequality, we used the cone condition\eqref{EQ:conecond}.
Observe now that it follows from the Cauchy-Schwarz inequality that
Fast rate for the Lasso
Fix [math]n \ge 2[/math]. Assume that the linear model\eqref{EQ:regmod_matrix} holds where [math]\eps\sim \sg_n(\sigma^2)[/math]. Moreover, assume that [math]|\theta^*|_0\le k[/math] and that [math]\X[/math] satisfies assumption [math]\textsf{INC$(k)$}[/math]. Then the Lasso estimator [math]\thetalasso[/math] with regularization parameter defined by
From the definition of [math]\thetalasso[/math], it holds
In particular, it implies that
Note that all we required for the proof was not really incoherence but the conclusion of Lemma:
where [math]\kappa=1/2[/math] and [math]\cC_S[/math] is the cone defined by
Condition\eqref{EQ:REcond} is sometimes called restricted eigenvalue (RE) condition. Its name comes from the following observation. Note that all [math]k[/math]-sparse vectors [math]\theta[/math] are in a cone [math]\cC_{S}[/math] with [math]|S|\le k[/math] so that the RE condition implies that the smallest eigenvalue of [math]\X_S[/math] satisfies [math]\lambda_\textrm{min}(\X_S)\ge n\kappa[/math] for all [math]S[/math] such that [math]|S|\le k[/math]. Clearly, the RE condition is weaker than incoherence and it can actually be shown that a design matrix [math]\X[/math] of i.i.d Rademacher random variables satisfies the RE conditions as soon as [math]n\ge Ck\log(d)[/math] with positive probability.
The Slope estimator
We noticed that the BIC estimator can actually obtain a rate of [math] k\log(ed/k) [/math], where [math] k = |\theta^\ast|_0 [/math], while the LASSO only achieves [math] k \log(ed) [/math]. This begs the question whether the same rate can be achieved by an efficiently computable estimator. The answer is yes, and the estimator achieving this is a slight modification of the LASSO, where the entries in the [math] \ell_1 [/math]-norm are weighted differently according to their size in order to get rid of a slight inefficiency in our bounds.
Let [math] \lambda = (\lambda_1, \dots, \lambda_d) [/math] be a non-increasing sequence of positive real numbers, [math] \lambda_1 \geq \lambda_2 \geq \dots \geq \lambda_d \gt 0 [/math]. For [math] \theta = (\theta_1, \dots, \theta_d) \in \R^d [/math], let [math] (\theta^\ast_1, \dots, \theta^\ast_d) [/math] be a non-increasing rearrangement of the modulus of the entries, [math] |\theta_1|, \dots, |\theta_d| [/math]. We define the sorted [math] \ell_1 [/math] norm of [math] \theta [/math] as
Slope stands for Sorted L-One Penalized Estimation and was introduced in [11], motivated by the quest for a penalized estimation procedure that could offer a control of false discovery rate for the hypotheses [math] H_{0,j}: \theta^\ast_j = 0 [/math]. We can check that [math] |\,.\,|_\ast [/math] is indeed a norm and that [math] \thetaslope [/math] can be computed efficiently, for example by proximal gradient algorithms, see [11]. In the following, we will consider
With this choice, we will exhibit a scaling in [math] \tau [/math] that leads to the desired high probability bounds, following the proofs in [12]. We begin with refined bounds on the suprema of Gaussians.
Let [math] g_1, \dots, g_d [/math] be zero-mean Gaussian variables with variance at most [math] \sigma^2 [/math]. Then, for any [math]k\le d[/math],
We consider the moment generating function to apply a Chernoff bound. Use Jensen's inequality on the sum to get
Define [math][d]:=\{1, \ldots, d\}[/math]. Under the same assumptions as in Lemma,
We can estimate [math] (g^\ast_k)^2 [/math] by the average of all larger variables,
For [math] t \gt 8 [/math],
In turn, this means that
Fix [math]n \ge 2[/math]. Assume that the linear model\eqref{EQ:regmod_matrix} holds where [math]\eps\sim \cN_n(0,\sigma^2 I_n)[/math]. Moreover, assume that [math]|\theta^*|_0\le k[/math] and that [math]\X[/math] satisfies assumption [math]\textsf{INC$(k')$}[/math] with [math] k' \geq 4k \log(2de/k) [/math]. Then the Slope estimator [math]\thetaslope[/math] with regularization parameter defined by
The multplicative depedence on [math] \log(1/\delta) [/math] in \eqref{EQ:fastMSESlope} is an artifact of the simplified proof we give here and can be improved to an additive one similar to the lasso case, \eqref{EQ:fastMSELasso}, by appealing to stronger and more general concentration inequalities. For more details, see [12].
By minimality of [math] \thetaslope [/math] in \eqref{eq:ad}, adding [math] n \tau |\thetaslope - \theta^\ast|_\ast [/math] on both sides,
By Lemma, we can estimate
Combined with [math] \tau = 8 \sqrt{2} \sigma \sqrt{\log(1/\delta)/n} [/math] and the basic inequality \eqref{eq:r}, we have that
We can now repeat the incoherence arguments from Lemma, with [math] S [/math] being the [math] k [/math] largest entries of [math] |u| [/math], to get the same conclusion under the restriction [math] \textsf{INC}(k') [/math].
First, by exactly the same argument as in Lemma, we have
Concluding, if [math] \textsf{INC}( k') [/math] holds with [math] k' \geq 4k \log(2de/k) [/math], taking into account that [math] \lambda_d \ge 1/2 [/math], we have
General references
Rigollet, Philippe; Hütter, Jan-Christian (2023). "High-Dimensional Statistics". arXiv:2310.19244 [math.ST].
Notes
- we could use Theorem directly here but at the cost of a factor 2 in the constant.
- Note that it minimizes the Bayes Information Criterion (BIC) employed in the traditional literature of asymptotic statistics if [math]\tau=\sqrt{\log(d)/n}[/math]. We will use the same value below, up to multiplicative constants (it's the price to pay to get non-asymptotic results).
References
- "Robust Face Recognition via Sparse Representation" (2009). IEEE Trans. Pattern Anal. Mach. Intell. 31. IEEE Computer Society.
- "Exponential screening and optimal rates of sparse estimation" (2011). Ann. Statist. 39.
- LeCam, L. (1973). "Convergence of estimates under dimensionality restrictions". Ann. Statist. 1.
- "{{{title}}}" .ffas|first1=StTemplate:\'ephane|last2=LecuTemplate:\'e|first2=Guillaume|journal=J. Mach. Learn. Res.|year=2011|title=Hyper-sparse optimal aggregation|volume=12}}
- Alex; re, B. (2004), Statistique appliqu\'ee
- "Regularization paths for generalized linear models via coordinate descent" . Journal of Statistical Software 33. NIH Public Access.
- "Least angle regression" (2004). Ann. Statist. 32.
- "A fast iterative shrinkage-thresholding algorithm for linear inverse problems" (2009). SIAM J. Imaging Sci. 2.
- Tibshirani, Robert (1996). "Regression shrinkage and selection via the lasso". J. Roy. Statist. Soc. Ser. B 58.
- Alex; re, B. (2004), Statistique appliqu\'ee
- 11.0 11.1 "Template:SLOPE\textemdash{}adaptive Variable Selection via Convex Optimization" (2015). The annals of applied statistics 9.
- 12.0 12.1 "Slope Meets Lasso: Improved Oracle Bounds and Optimality" (2016). arXiv preprint arXiv:1605.08651.