guide:0251dfcdad: Difference between revisions
mNo edit summary |
mNo edit summary |
||
(3 intermediate revisions by the same user not shown) | |||
Line 157: | Line 157: | ||
\ | \newcommandi.i.d{i.i.d\@ifnextchar.{}{.\@\xspace} } | ||
\newcommand\MoveEqLeft[1][2]{\kern #1em & \kern -#1em} | \newcommand\MoveEqLeft[1][2]{\kern #1em & \kern -#1em} | ||
\newcommand{\leadeq}[2][4]{\MoveEqLeft[#1] #2 \nonumber} | \newcommand{\leadeq}[2][4]{\MoveEqLeft[#1] #2 \nonumber} | ||
Line 247: | Line 247: | ||
===Definition and first properties=== | ===Definition and first properties=== | ||
Gaussian tails are practical when controlling the tail of an average of independent random variables. Indeed, recall that if <math>X_1, \ldots, X_n</math> are \ | Gaussian tails are practical when controlling the tail of an average of independent random variables. Indeed, recall that if <math>X_1, \ldots, X_n</math> are \i.i.d <math>\cN(\mu,\sigma^2)</math>, then <math>\bar X=\frac{1}{n}\sum_{i=1}^nX_i\sim\cN(\mu,\sigma^2/n)</math>. Using [[#lem:subgauss |Lemma]] below for example, we get | ||
<math display="block"> | <math display="block"> | ||
Line 254: | Line 254: | ||
Equating the right-hand side with some confidence level <math>\delta > 0</math>, we find that with probability at least<ref group="Notes" >We will often commit the statement ‘`at least" for brevity</ref> <math>1-\delta</math>, | Equating the right-hand side with some confidence level <math>\delta > 0</math>, we find that with probability at least<ref group="Notes" >We will often commit the statement ‘`at least" for brevity</ref> <math>1-\delta</math>, | ||
<span id="EQ:subG:CI"/> | |||
<math display="block"> | <math display="block"> | ||
\begin{equation} | \begin{equation} | ||
Line 267: | Line 268: | ||
A random variable <math>X \in \R</math> is said to be ’'sub-Gaussian'' with variance proxy <math>\sigma^2</math> if <math>\E[X]=0</math> and its moment generating function satisfies | A random variable <math>X \in \R</math> is said to be ’'sub-Gaussian'' with variance proxy <math>\sigma^2</math> if <math>\E[X]=0</math> and its moment generating function satisfies | ||
<span id{{=}}"EQ:defsubgauss"/> | |||
<math display="block"> | <math display="block"> | ||
\begin{equation} | \begin{equation} | ||
Line 285: | Line 287: | ||
{{proofcard|Lemma|lem:subgauss|Let <math>X \sim \sg( \sigma^2)</math>. Then for any <math>t > 0</math>, it holds | {{proofcard|Lemma|lem:subgauss|Let <math>X \sim \sg( \sigma^2)</math>. Then for any <math>t > 0</math>, it holds | ||
<span id="EQ:LEM:subgausstails"/> | |||
<math display="block"> | <math display="block"> | ||
\begin{equation} | \begin{equation} | ||
Line 316: | Line 319: | ||
{{proofcard|Lemma|LEM:subgauss_moments|Let <math>X</math> be a random variable such that | {{proofcard|Lemma|LEM:subgauss_moments|Let <math>X</math> be a random variable such that | ||
<span id="LEM:subgauss_moments"/> | |||
<math display="block"> | <math display="block"> | ||
\begin{equation}\label{LEM:subgauss_moments}\end{equation} | \begin{equation}\label{LEM:subgauss_moments}\end{equation} | ||
Line 369: | Line 373: | ||
From the above Lemma, we see that sub-Gaussian random variables can be equivalently defined from their tail bounds and their moment generating functions, up to constants. | From the above Lemma, we see that sub-Gaussian random variables can be equivalently defined from their tail bounds and their moment generating functions, up to constants. | ||
===Sums of independent sub-Gaussian random variables=== | ===Sums of independent sub-Gaussian random variables=== | ||
Recall that if <math>X_1, \ldots, X_n</math> are \ | Recall that if <math>X_1, \ldots, X_n</math> are \i.i.d <math>\cN(0,\sigma^2)</math>, then for any <math>a \in \R^n</math>, | ||
<math display="block"> | <math display="block"> | ||
Line 525: | Line 529: | ||
</math>}} | </math>}} | ||
This leads to the following definition | This leads to the following definition | ||
{{defncard|label=id=|A random variable <math>X</math> is said to be sub-exponential with parameter <math>\lambda</math> (denoted <math>X \sim \subE(\lambda)</math>) if <math>\E[X]=0</math> and its moment generating function satisfies | {{defncard|label=|id=|A random variable <math>X</math> is said to be sub-exponential with parameter <math>\lambda</math> (denoted <math>X \sim \subE(\lambda)</math>) if <math>\E[X]=0</math> and its moment generating function satisfies | ||
<math display="block"> | <math display="block"> | ||
Line 579: | Line 583: | ||
We obtain the same bound for <math>\p(\bar X < -t)</math> which concludes the proof.}} | We obtain the same bound for <math>\p(\bar X < -t)</math> which concludes the proof.}} | ||
Note that usually, Bernstein's inequality refers to a slightly more precise result that is qualitatively the same as the one above: it exhibits a Gaussian tail <math>e^{-nt^2/(2\lambda^2)}</math> and an exponential tail <math>e^{-nt/(2\lambda)}</math>. See for example Theorem2.10 in <ref name="BouLugMas13">{{ | Note that usually, Bernstein's inequality refers to a slightly more precise result that is qualitatively the same as the one above: it exhibits a Gaussian tail <math>e^{-nt^2/(2\lambda^2)}</math> and an exponential tail <math>e^{-nt/(2\lambda)}</math>. See for example Theorem2.10 in <ref name="BouLugMas13">{{cite book|authors=|last1=Boucheron|first1=St\'ephane|last2=Lugosi|first2=G\'abor|last3=Massart|first3=Pascal|year=2013|title=Concentration inequalities|volume=13|number=2|publisher=Oxford University Press|isbn=0-521-38991-7|location=Oxford|pages=x+481}}</ref>. | ||
==Maximal inequalities== | ==Maximal inequalities== | ||
The exponential inequalities of the previous section are valid for linear combinations of independent random variables, and, in particular, for the average <math>\bar X</math>. In many instances, we will be interested in controlling the ''maximum'' over the parameters of such linear combinations (this is because of empirical risk minimization). The purpose of this section is to present such results. | The exponential inequalities of the previous section are valid for linear combinations of independent random variables, and, in particular, for the average <math>\bar X</math>. In many instances, we will be interested in controlling the ''maximum'' over the parameters of such linear combinations (this is because of empirical risk minimization). The purpose of this section is to present such results. | ||
Line 629: | Line 633: | ||
</math> | </math> | ||
where <math>X_{N+i}=-X_i</math> for <math>i=1, \ldots, N</math>.}} | where <math>X_{N+i}=-X_i</math> for <math>i=1, \ldots, N</math>.}} | ||
Extending these results to a maximum over an infinite set may be impossible. For example, if one is given an infinite sequence of \ | Extending these results to a maximum over an infinite set may be impossible. For example, if one is given an infinite sequence of \i.i.d <math>\cN(0, \sigma^2)</math> random variables <math>X_1, X_2, \ldots</math>, then for any <math>N \ge 1</math>, we have for any <math>t > 0</math>, | ||
<math display="block"> | <math display="block"> | ||
Line 642: | Line 646: | ||
Examples from statistics have structure and we encounter many examples where a maximum of random variables over an infinite set is in fact finite. This is due to the fact that the random variables that we are considering are not independent of each other. In the rest of this section, we review some of these examples. | Examples from statistics have structure and we encounter many examples where a maximum of random variables over an infinite set is in fact finite. This is due to the fact that the random variables that we are considering are not independent of each other. In the rest of this section, we review some of these examples. | ||
===Maximum over a convex polytope=== | ===Maximum over a convex polytope=== | ||
We use the definition of a polytope from <ref name="Gru03">{{ | We use the definition of a polytope from <ref name="Gru03">{{cite book|authors=|last=Grunbaum|first=Branko|year=2003|title=Convex polytopes|volume=221|number=4|publisher=Springer-Verlag|isbn=0-387-40409-0|location=New York|pages=xvi+468}}</ref>: a convex polytope <math>\mathsf{P}</math> is a compact set with a finite number of vertices <math>\cV(\mathsf{P})</math> called extreme points. It satisfies <math>\mathsf{P}=\conv(\cV(\mathsf{P}))</math>, where <math>\conv(\cV(\mathsf{P}))</math> denotes the convex hull of the vertices of <math>\mathsf{P}</math>. | ||
Let <math>X \in \R^d</math> be a random vector and consider the (infinite) family of random variables | Let <math>X \in \R^d</math> be a random vector and consider the (infinite) family of random variables | ||
Line 691: | Line 695: | ||
</math> | </math> | ||
Clearly, this ball is not a polytope and yet, we can control the maximum of random variables indexed by <math>\cB_2</math>. This is due to the fact that there exists a finite subset of <math>\cB_2</math> such that the maximum over this finite set is of the same order as the maximum over the entire ball. | Clearly, this ball is not a polytope and yet, we can control the maximum of random variables indexed by <math>\cB_2</math>. This is due to the fact that there exists a finite subset of <math>\cB_2</math> such that the maximum over this finite set is of the same order as the maximum over the entire ball. | ||
{{defncard|label=id=|Fix <math>K\subset \R^d</math> and <math>\eps > 0</math>. A set <math>\cN</math> is called an <math>\eps</math>-net of <math>K</math> with respect to a distance <math>d(\cdot, \cdot)</math> on <math>\R^d</math>, if <math>\cN \subset K</math> and for any <math>z \in K</math>, there exists <math>x \in \cN</math> such that <math>d(x,z)\le \eps</math>.}} | {{defncard|label=|id=|Fix <math>K\subset \R^d</math> and <math>\eps > 0</math>. A set <math>\cN</math> is called an <math>\eps</math>-net of <math>K</math> with respect to a distance <math>d(\cdot, \cdot)</math> on <math>\R^d</math>, if <math>\cN \subset K</math> and for any <math>z \in K</math>, there exists <math>x \in \cN</math> such that <math>d(x,z)\le \eps</math>.}} | ||
Therefore, if <math>\cN</math> is an <math>\eps</math>-net of <math>K</math> with respect to a norm <math>\|\cdot\|</math>, then every point of <math>K</math> is at distance at most <math>\eps</math> from a point in <math>\cN</math>. Clearly, every compact set admits a finite <math>\eps</math>-net. The following lemma gives an upper bound on the size of the smallest <math>\eps</math>-net of <math>\cB_2</math>. | Therefore, if <math>\cN</math> is an <math>\eps</math>-net of <math>K</math> with respect to a norm <math>\|\cdot\|</math>, then every point of <math>K</math> is at distance at most <math>\eps</math> from a point in <math>\cN</math>. Clearly, every compact set admits a finite <math>\eps</math>-net. The following lemma gives an upper bound on the size of the smallest <math>\eps</math>-net of <math>\cB_2</math>. | ||
{{proofcard|Lemma|lem:coveringell2|Fix <math>\eps\in(0,1)</math>. Then the unit Euclidean ball <math>\cB_2</math> has an <math>\eps</math>-net <math>\cN</math> with respect to the Euclidean distance of cardinality <math>|\cN|\le (3/\eps)^d</math> | {{proofcard|Lemma|lem:coveringell2|Fix <math>\eps\in(0,1)</math>. Then the unit Euclidean ball <math>\cB_2</math> has an <math>\eps</math>-net <math>\cN</math> with respect to the Euclidean distance of cardinality <math>|\cN|\le (3/\eps)^d</math> | ||
Line 757: | Line 761: | ||
==Sums of independent random matrices== | ==Sums of independent random matrices== | ||
In this section, we are going to explore how concentration statements can be extended to sums of matrices. | In this section, we are going to explore how concentration statements can be extended to sums of matrices. | ||
As an example, we are going to show a version of [[#TH:Bernstein |Bernstein's inequality]], for sums of independent matrices, closely following the presentation in <ref name="Tro12">{{cite journal|last=Tropp|first=Joel A.|journal=Foundations of computational mathematics|year=2012|title=User-Friendly Tail Bounds for Sums of Random Matrices|volume=12|number=4}}</ref>, which builds on previous work in <ref name="AhlWin02">{{cite journal|last1=Ahlswede|first1=Rudolf|last2=Winter|first2=Andreas|journal=IEEE Transactions on Information Theory|year=2002|title=Strong Converse for Identification via Quantum Channels|volume=48|number=3}}</ref>. | As an example, we are going to show a version of [[#TH:Bernstein |Bernstein's inequality]], for sums of independent matrices, closely following the presentation in <ref name="Tro12">{{cite journal|last=Tropp|first=Joel A.|journal=Foundations of computational mathematics|year=2012|title=User-Friendly Tail Bounds for Sums of Random Matrices|volume=12|number=4|doi=10.1214/aos/1034276635|publisher=Springer-Verlag|url-access=http://dx.doi.org/10.1214/aos/1034276635|eprint=1108.1170v6|pages=389--434}}</ref>, which builds on previous work in <ref name="AhlWin02">{{cite journal|last1=Ahlswede|first1=Rudolf|last2=Winter|first2=Andreas|journal=IEEE Transactions on Information Theory|year=2002|title=Strong Converse for Identification via Quantum Channels|volume=48|number=3|doi=10.1214/aos/1034276635|publisher=Springer-Verlag|url-access=http://dx.doi.org/10.1214/aos/1034276635|eprint=1108.1170v6|pages=569--579}}</ref>. | ||
Results of this type have been crucial in providing guarantees for low rank recovery, see for example <ref name="Gro11">{{cite journal|last=Gross|first=David|journal=IEEE Transactions on Information Theory|year=2011|title=Recovering Low-Rank Matrices from Few Coefficients in Any Basis|volume=57|number=3}}</ref>. | Results of this type have been crucial in providing guarantees for low rank recovery, see for example <ref name="Gro11">{{cite journal|last=Gross|first=David|journal=IEEE Transactions on Information Theory|year=2011|title=Recovering Low-Rank Matrices from Few Coefficients in Any Basis|volume=57|number=3|doi=10.1214/aos/1034276635|publisher=Springer-Verlag|url-access=http://dx.doi.org/10.1214/aos/1034276635|eprint=1108.1170v6|pages=1548--1566}}</ref>. | ||
In particular, we want to control the maximum eigenvalue of a sum of independent random symmetric matrices <math> \p(\lambda_{\mathrm{max}}(\sum_i \bX_i) > t)</math>. | In particular, we want to control the maximum eigenvalue of a sum of independent random symmetric matrices <math> \p(\lambda_{\mathrm{max}}(\sum_i \bX_i) > t)</math>. | ||
The tools involved will closely mimic those used for scalar random variables, but with the caveat that care must be taken when handling exponentials of matrices because <math> e^{A + B} \neq e^{A} e^{B} </math> if <math> A, B \in \R^d </math> and <math> AB \neq BA </math>. | The tools involved will closely mimic those used for scalar random variables, but with the caveat that care must be taken when handling exponentials of matrices because <math> e^{A + B} \neq e^{A} e^{B} </math> if <math> A, B \in \R^d </math> and <math> AB \neq BA </math>. | ||
Line 769: | Line 773: | ||
For a symmetric matrix <math> \bX </math>, we can define a functional calculus by applying a function <math> f : \R \to \R </math> to the diagonal elements of its spectral decomposition, \ie, if <math> \bX = \bQ \Lambda \bQ^\top </math>, then | For a symmetric matrix <math> \bX </math>, we can define a functional calculus by applying a function <math> f : \R \to \R </math> to the diagonal elements of its spectral decomposition, \ie, if <math> \bX = \bQ \Lambda \bQ^\top </math>, then | ||
<span id="eq:funccalc"/> | |||
<math display="block"> | <math display="block"> | ||
\begin{equation} | \begin{equation} | ||
Line 777: | Line 782: | ||
where | where | ||
<span id="eq:al"/> | |||
<math display="block"> | <math display="block"> | ||
\begin{equation} | \begin{equation} | ||
Line 786: | Line 792: | ||
From the definition, it is clear that for the spectrum of the resulting matrix, | From the definition, it is clear that for the spectrum of the resulting matrix, | ||
<span id="eq:specthm"/> | |||
<math display="block"> | <math display="block"> | ||
\begin{equation} | \begin{equation} | ||
Line 794: | Line 801: | ||
While inequalities between functions do not generally carry over to matrices, we have the following ''transfer rule'': | While inequalities between functions do not generally carry over to matrices, we have the following ''transfer rule'': | ||
<span id="eq:g"/> | |||
<math display="block"> | <math display="block"> | ||
\begin{equation} | \begin{equation} | ||
Line 813: | Line 821: | ||
For <math> \bA \preceq \bB </math>, | For <math> \bA \preceq \bB </math>, | ||
<span id="eq:h"/> | |||
<math display="block"> | <math display="block"> | ||
\begin{equation} | \begin{equation} | ||
Line 821: | Line 830: | ||
and for <math> 0 \prec \bA \preceq \bB </math>, | and for <math> 0 \prec \bA \preceq \bB </math>, | ||
<span id="eq:i"/> | |||
<math display="block"> | <math display="block"> | ||
\begin{equation} | \begin{equation} | ||
Line 841: | Line 851: | ||
|We multiply both sides of the inequality <math> \lambda_{\mathrm{max}}(\bY) \geq t </math> by <math> \theta </math>, take exponentials, apply the spectral theorem \eqref{eq:specthm} and then estimate the maximum eigenvalue by the sum over all eigenvalues, the trace. | |We multiply both sides of the inequality <math> \lambda_{\mathrm{max}}(\bY) \geq t </math> by <math> \theta </math>, take exponentials, apply the spectral theorem \eqref{eq:specthm} and then estimate the maximum eigenvalue by the sum over all eigenvalues, the trace. | ||
<span id="eq:f"/> | |||
<math display="block"> | <math display="block"> | ||
\begin{align*} | \begin{align*} | ||
Line 874: | Line 885: | ||
\end{equation*} | \end{equation*} | ||
</math> | </math> | ||
This is the approach followed by Ahlswede-Winter <ref name="AhlWin02" | This is the approach followed by Ahlswede-Winter <ref name="AhlWin02"/>, which leads to worse constants for concentration than the ones we obtain below. | ||
Instead, we are going to use the following deep theorem due to Lieb <ref name="Lie73">{{cite journal|last=Lieb|first=Elliott H.|journal=Advances in Mathematics|year=1973|title=Convex Trace Functions and the Wigner-Yanase-Dyson Conjecture|volume=11|number=3}}</ref>. A sketch of the proof can be found in the appendix of <ref name="Rus02">{{cite journal|last=Ruskai|first=Mary Beth|journal=Journal of Mathematical Physics|year=2002|title=Inequalities for Quantum Entropy: A Review with Conditions for Equality|volume=43|number=9}}</ref>. | Instead, we are going to use the following deep theorem due to Lieb <ref name="Lie73">{{cite journal|last=Lieb|first=Elliott H.|journal=Advances in Mathematics|year=1973|title=Convex Trace Functions and the Wigner-Yanase-Dyson Conjecture|volume=11|number=3|doi=10.1214/aos/1034276635|publisher=Springer-Verlag|url-access=http://dx.doi.org/10.1214/aos/1034276635|eprint=1108.1170v6|pages=267--288}}</ref>. A sketch of the proof can be found in the appendix of <ref name="Rus02">{{cite journal|last=Ruskai|first=Mary Beth|journal=Journal of Mathematical Physics|year=2002|title=Inequalities for Quantum Entropy: A Review with Conditions for Equality|volume=43|number=9|doi=10.1214/aos/1034276635|publisher=Springer-Verlag|url-access=http://dx.doi.org/10.1214/aos/1034276635|eprint=1108.1170v6|pages=4358--4375}}</ref>. | ||
{{proofcard|Theorem (Lieb)|thm:lieb|Let <math> \bH \in \R^{d \times d} </math> be symmetric. | {{proofcard|Theorem (Lieb)|thm:lieb|Let <math> \bH \in \R^{d \times d} </math> be symmetric. | ||
Then, | Then, | ||
Line 895: | Line 906: | ||
|Write <math> \bY = e^{\bX} </math> and use Jensen's inequality: | |Write <math> \bY = e^{\bX} </math> and use Jensen's inequality: | ||
<span id="eq:e"/> | |||
<math display="block"> | <math display="block"> | ||
\begin{align*} | \begin{align*} | ||
Line 1,043: | Line 1,055: | ||
The second inequality then follows from the fact that <math> h(u) \geq h_2(u) = \frac{u^2/2}{1+u/3} </math> for <math> u \geq 0 </math> which can be verified by comparing derivatives. | The second inequality then follows from the fact that <math> h(u) \geq h_2(u) = \frac{u^2/2}{1+u/3} </math> for <math> u \geq 0 </math> which can be verified by comparing derivatives. | ||
The third inequality follows from exploiting the properties of <math> h_2 </math>.}} | The third inequality follows from exploiting the properties of <math> h_2 </math>.}} | ||
With similar techniques, one can also prove a version of Hoeffding's inequality for matrices, see <ref name="Tro12" | With similar techniques, one can also prove a version of Hoeffding's inequality for matrices, see <ref name="Tro12"/>{{rp|at=Theorem 1.3}}. | ||
==General references== | ==General references== |
Latest revision as of 01:27, 3 June 2024
[math] \newcommand{\DS}{\displaystyle} \newcommand{\intbeta}{\lfloor \beta \rfloor} \newcommand{\cA}{\mathcal{A}} \newcommand{\cB}{\mathcal{B}} \newcommand{\cC}{\mathcal{C}} \newcommand{\cD}{\mathcal{D}} \newcommand{\cE}{\mathcal{E}} \newcommand{\cF}{\mathcal{F}} \newcommand{\cG}{\mathcal{G}} \newcommand{\cH}{\mathcal{H}} \newcommand{\cI}{\mathcal{I}} \newcommand{\cJ}{\mathcal{J}} \newcommand{\cK}{\mathcal{K}} \newcommand{\cL}{\mathcal{L}} \newcommand{\cM}{\mathcal{M}} \newcommand{\cN}{\mathcal{N}} \newcommand{\cO}{\mathcal{O}} \newcommand{\cP}{\mathcal{P}} \newcommand{\cQ}{\mathcal{Q}} \newcommand{\cS}{\mathcal{S}} \newcommand{\cT}{\mathcal{T}} \newcommand{\cU}{\mathcal{U}} \newcommand{\cV}{\mathcal{V}} \newcommand{\cX}{\mathcal{X}} \newcommand{\cY}{\mathcal{Y}} \newcommand{\cZ}{\mathcal{Z}} \newcommand{\sg}{\mathsf{subG}} \newcommand{\subE}{\mathsf{subE}} \newcommand{\bA}{\mathbf{A}} \newcommand{\bB}{\mathbf{B}} \newcommand{\bC}{\mathbf{C}} \newcommand{\bD}{\mathbf{D}} \newcommand{\bE}{\mathbf{E}} \newcommand{\bF}{\mathbf{F}} \newcommand{\bG}{\mathbf{G}} \newcommand{\bH}{\mathbf{H}} \newcommand{\bI}{\mathbf{I}} \newcommand{\bJ}{\mathbf{J}} \newcommand{\bK}{\mathbf{K}} \newcommand{\bM}{\mathbf{M}} \newcommand{\bN}{\mathbf{N}} \newcommand{\bO}{\mathbf{O}} \newcommand{\bP}{\mathbf{P}} \newcommand{\bp}{\mathbf{p}} \newcommand{\bQ}{\mathbf{Q}} \newcommand{\bS}{\mathbf{S}} \newcommand{\bT}{\mathbf{T}} \newcommand{\bU}{\mathbf{U}} \newcommand{\bV}{\mathbf{V}} \newcommand{\bX}{\mathbf{X}} \newcommand{\bY}{\mathbf{Y}} \newcommand{\bZ}{\mathbf{Z}} \newcommand{\bflambda}{\boldsymbol{\lambda}} \newcommand{\bftheta}{\boldsymbol{\theta}} \newcommand{\bfg}{\boldsymbol{g}} \newcommand{\bfy}{\boldsymbol{y}} \def\thetaphat{\hat{\bftheta}_\bp} \def\bflam{\boldsymbol{\lambda}} \def\Lam{\Lambda} \def\lam{\lambda} \def\bfpi{\boldsymbol{\pi}} \def\bfz{\boldsymbol{z}} \def\bfw{\boldsymbol{w}} \def\bfeta{\boldsymbol{\eta}} \newcommand{\R}{\mathrm{ I}\kern-0.18em\mathrm{ R}} \newcommand{\h}{\mathrm{ I}\kern-0.18em\mathrm{ H}} \newcommand{\K}{\mathrm{ I}\kern-0.18em\mathrm{ K}} \newcommand{\p}{\mathrm{ I}\kern-0.18em\mathrm{ P}} \newcommand{\E}{\mathrm{ I}\kern-0.18em\mathrm{ E}} %\newcommand{\Z}{\mathrm{ Z}\kern-0.26em\mathrm{ Z}} \newcommand{\1}{\mathrm{ 1}\kern-0.24em\mathrm{ I}} \newcommand{\N}{\mathrm{ I}\kern-0.18em\mathrm{ N}} \newcommand{\field}[1]{\mathbb{#1}} \newcommand{\q}{\field{Q}} \newcommand{\Z}{\field{Z}} \newcommand{\X}{\field{X}} \newcommand{\Y}{\field{Y}} \newcommand{\bbS}{\field{S}} \newcommand{\n}{\mathcal{N}} \newcommand{\x}{\mathcal{X}} \newcommand{\pp}{{\sf p}} \newcommand{\hh}{{\sf h}} \newcommand{\ff}{{\sf f}} \newcommand{\Bern}{\mathsf{Ber}} \newcommand{\Bin}{\mathsf{Bin}} \newcommand{\Lap}{\mathsf{Lap}} \newcommand{\tr}{\mathsf{Tr}} \newcommand{\phin}{\varphi_n} \newcommand{\phinb}{\overline \varphi_n(t)} \newcommand{\pn}{\p_{\kern-0.25em n}} \newcommand{\pnm}{\p_{\kern-0.25em n,m}} \newcommand{\psubm}{\p_{\kern-0.25em m}} \newcommand{\psubp}{\p_{\kern-0.25em p}} \newcommand{\cfi}{\cF_{\kern-0.25em \infty}} \newcommand{\e}{\mathrm{e}} \newcommand{\ic}{\mathrm{i}} \newcommand{\Leb}{\mathrm{Leb}_d} \newcommand{\Var}{\mathrm{Var}} \newcommand{\ddelta}{d_{\symdiffsmall}} \newcommand{\dsubh}{d_H} \newcommand{\indep}{\perp\kern-0.95em{\perp}} \newcommand{\supp}{\mathop{\mathrm{supp}}} \newcommand{\rank}{\mathop{\mathrm{rank}}} \newcommand{\vol}{\mathop{\mathrm{vol}}} \newcommand{\conv}{\mathop{\mathrm{conv}}} \newcommand{\card}{\mathop{\mathrm{card}}} \newcommand{\argmin}{\mathop{\mathrm{argmin}}} \newcommand{\argmax}{\mathop{\mathrm{argmax}}} \newcommand{\ud}{\mathrm{d}} \newcommand{\var}{\mathrm{var}} \newcommand{\re}{\mathrm{Re}} \newcommand{\MSE}{\mathsf{MSE}} \newcommand{\im}{\mathrm{Im}} \newcommand{\epr}{\hfill\hbox{\hskip 4pt\vrule width 5pt height 6pt depth 1.5pt}\vspace{0.5cm}\par} \newcommand{\bi}[1]{^{(#1)}} \newcommand{\eps}{\varepsilon} \newcommand{\Deq}{\stackrel{\mathcal{D}}{=}} \newcommand{\ubar}{\underbar} \newcommand{\Kbeta}{K_{\hspace{-0.3mm} \beta}} \newcommand{\crzero}[1]{\overset{r_0}{\underset{#1}{\longleftrightarrow}}} \newcommand{\hint}[1]{\texttt{[Hint:#1]}} \newcommand{\vp}{\vspace{.25cm}} \newcommand{\vm}{\vspace{.5cm}} \newcommand{\vg}{\vspace{1cm}} \newcommand{\vgneg}{\vspace{-1cm}} \newcommand{\vneg}{\vspace{-.5cm}} \newcommand{\vpneg}{\vspace{-.25cm}} \newcommand{\tp}{\ptsize{10}} \newcommand{\douzp}{\ptsize{12}} \newcommand{\np}{\ptsize{9}} \newcommand{\hp}{\ptsize{8}} \newcommand{\red}{\color{red}} \newcommand{\ndpr}[1]{{\textsf{\red[#1]}}} \newcommandi.i.d{i.i.d\@ifnextchar.{}{.\@\xspace} } \newcommand\MoveEqLeft[1][2]{\kern #1em & \kern -#1em} \newcommand{\leadeq}[2][4]{\MoveEqLeft[#1] #2 \nonumber} \newcommand{\leadeqnum}[2][4]{\MoveEqLeft[#1] #2} \newcommand\independent{\protect\mathpalette{\protect\independenT}{\perp}} \def\independenT#1#2{\mathrel{\rlap{$#1#2$}\mkern2mu{#1#2}}} \newcommand{\MIT}[1]{{\color{MITred} #1}} \newcommand{\dHyp}{\{-1,1\}^d} \newcommand{\thetahard}{\hat \theta^{hrd}} \newcommand{\thetasoft}{\hat \theta^{sft}} \newcommand{\thetabic}{\hat \theta^{bic}} \newcommand{\thetalasso}{\hat \theta^{\cL}} \newcommand{\thetaslope}{\hat \theta^{\cS}} \newcommand{\thetahard}{\hat \theta^{hrd}} \newcommand{\thetasoft}{\hat \theta^{sft}} \newcommand{\thetabic}{\hat \theta^{bic}} \newcommand{\thetalasso}{\hat \theta^{\cL}} \newcommand{\thetaslope}{\hat \theta^{\cS}} \newcommand{\thetals}{\hat \theta^{ls}} \newcommand{\thetalsm}{\tilde \theta^{ls_X}} \newcommand{\thetaridge}{\hat\theta^{\mathrm{ridge}}_\tau} \newcommand{\thetalsK}{\hat \theta^{ls}_K} \newcommand{\muls}{\hat \mu^{ls}} [/math]
Gaussian tails and MGF
Recall that a random variable [math]X \in \R[/math] has Gaussian distribution iff it has a density [math]p[/math] with respect to the Lebesgue measure on [math]\R[/math] given by
where [math]\mu=\E(X) \in \R[/math] and [math]\sigma^2=\var(X) \gt 0[/math] are the mean and variance of [math]X[/math]. We write [math]X \sim \cN(\mu, \sigma^2)[/math]. Note that [math]X=\sigma Z+\mu[/math] for [math]Z \sim \cN(0,1)[/math] (called standard Gaussian) and where the equality holds in distribution. Clearly, this distribution has unbounded support but it is well known that it has almost bounded support in the following sense: [math]\p(|X-\mu|\le 3\sigma)\simeq 0.997[/math]. This is due to the fast decay of the tails of [math]p[/math] as [math]|x|\to \infty[/math] (see [[#fig:gaussdens |Figure]). This decay can be quantified using the following proposition (Mill's inequality).
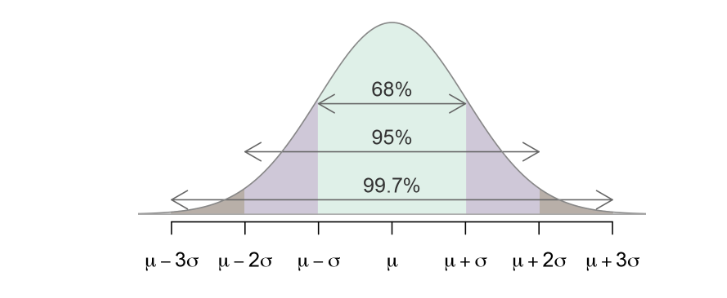
Let [math]X[/math] be a Gaussian random variable with mean [math]\mu[/math] and variance [math]\sigma^2[/math] then for any [math]t \gt 0[/math], it holds
Note that it is sufficient to prove the theorem for [math]\mu=0[/math] and [math]\sigma^2=1[/math] by simple translation and rescaling. We get for [math]Z\sim \cN(0,1)[/math],
The fact that a Gaussian random variable [math]Z[/math] has tails that decay to zero exponentially fast can also be seen in the moment generating function (MGF)
Indeed, in the case of a standard Gaussian random variable, we have
It follows that if [math]X\sim \cN(\mu,\sigma^2)[/math], then [math]\E[\exp(sX)]=\exp\big(s\mu+\frac{\sigma^2s^2}{2}\big)[/math].
Sub-Gaussian random variables and Chernoff bounds
Definition and first properties
Gaussian tails are practical when controlling the tail of an average of independent random variables. Indeed, recall that if [math]X_1, \ldots, X_n[/math] are \i.i.d [math]\cN(\mu,\sigma^2)[/math], then [math]\bar X=\frac{1}{n}\sum_{i=1}^nX_i\sim\cN(\mu,\sigma^2/n)[/math]. Using Lemma below for example, we get
Equating the right-hand side with some confidence level [math]\delta \gt 0[/math], we find that with probability at least[Notes 1] [math]1-\delta[/math],
This is almost the confidence interval that you used in introductory statistics. The only difference is that we used an approximation for the Gaussian tail whereas statistical tables or software use a much more accurate computation. Figure shows the ratio of the width of the confidence interval to that of the confidence interval computed by the software R. It turns out that intervals of the same form can be also derived for non-Gaussian random variables as long as they have sub-Gaussian tails.
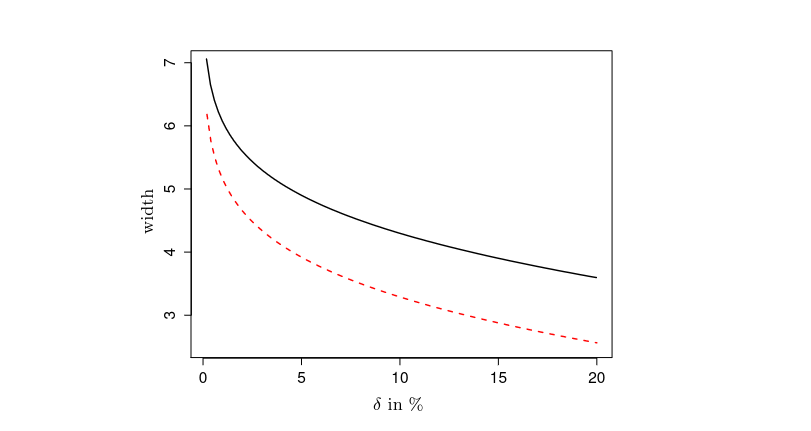
A random variable [math]X \in \R[/math] is said to be ’'sub-Gaussian with variance proxy [math]\sigma^2[/math] if [math]\E[X]=0[/math] and its moment generating function satisfies
This property can equivalently be expressed in terms of bounds on the tail of the random variable [math]X[/math].
Assume first that [math]X \sim \sg( \sigma^2)[/math]. We will employ a very useful technique called Chernoff bound that allows to translate a bound on the moment generating function into a tail bound. Using Markov's inequality, we have for any [math]s \gt 0[/math],
{{{4}}}
Moments
Recall that the absolute moments of [math]Z \sim \cN(0, \sigma^2)[/math] are given by
where [math]\Gamma(\cdot)[/math] denotes the Gamma function defined by
The next lemma shows that the tail bounds of Lemma are sufficient to show that the absolute moments of [math]X \sim \sg( \sigma^2)[/math] can be bounded by those of [math]Z\sim \cN(0,\sigma^2)[/math] up to multiplicative constants.
{{{4}}}
Using moments, we can prove the following reciprocal to Lemma.
If\eqref{EQ:LEM:subgausstails} holds and [math] \E[X] = 0 [/math], then for any [math]s \gt 0[/math], it holds
We use the Taylor expansion of the exponential function as follows. Observe that by the dominated convergence theorem
From the above Lemma, we see that sub-Gaussian random variables can be equivalently defined from their tail bounds and their moment generating functions, up to constants.
Sums of independent sub-Gaussian random variables
Recall that if [math]X_1, \ldots, X_n[/math] are \i.i.d [math]\cN(0,\sigma^2)[/math], then for any [math]a \in \R^n[/math],
If we only care about the tails, this property is preserved for sub-Gaussian random variables.
Let [math]X=(X_1, \ldots, X_n)[/math] be a vector of independent sub-Gaussian random variables that have variance proxy [math]\sigma^2[/math]. Then, the random vector [math]X[/math] is sub-Gaussian with variance proxy [math]\sigma^2[/math].
Let [math]u \in \cS^{n-1}[/math] be a unit vector, then
Using a Chernoff bound, we immediately get the following corollary
Let [math]X_1, \ldots, X_n[/math] be [math]n[/math] independent random variables such that [math]X_i \sim \sg( \sigma^2)[/math]. Then for any [math]a \in \R^n[/math], we have
Of special interest is the case where [math]a_i=1/n[/math] for all [math]i[/math]. Then, we get that the average [math]\bar X=\frac{1}{n}\sum_{i=1}^n X_i[/math], satisfies
just like for the Gaussian average.
Hoeffding's inequality
The class of sub-Gaussian random variables is actually quite large. Indeed, Hoeffding's lemma below implies that all random variables that are bounded uniformly are actually sub-Gaussian with a variance proxy that depends on the size of their support.
Let [math]X[/math] be a random variable such that [math]\E(X)=0[/math] and [math]X \in [a,b][/math] almost surely. Then, for any [math]s \in \R[/math], it holds
Define [math]\psi(s)=\log \E[e^{s X}][/math], and observe that and we can readily compute
Using a Chernoff bound, we get the following (extremely useful) result.
Let [math]X_1, \ldots, X_n[/math] be [math]n[/math] independent random variables such that almost surely,
Note that Hoeffding's lemma holds for any bounded random variable. For example, if one knows that [math]X[/math] is a Rademacher random variable,
Note that 2 is the best possible constant in the above approximation. For such variables, [math]a=-1, b=1[/math], and [math]\E(X)=0[/math], so Hoeffding's lemma yields
Hoeffding's inequality is very general but there is a price to pay for this generality. Indeed, if the random variables have small variance, we would like to see it reflected in the exponential tail bound (as for the Gaussian case) but the variance does not appear in Hoeffding's inequality. We need a more refined inequality.
Sub-exponential random variables
What can we say when a centered random variable is not sub-Gaussian? A typical example is the double exponential (or Laplace) distribution with parameter 1, denoted by [math]\Lap(1)[/math]. Let [math]X \sim \Lap(1)[/math] and observe that
In particular, the tails of this distribution do not decay as fast as the Gaussian ones (that decays as [math]e^{-t^2/2}[/math]). Such tails are said to be heavier than Gaussian. This tail behavior is also captured by the moment generating function of [math]X[/math]. Indeed, we have
and it is not defined for [math]s \ge 1[/math]. It turns out that a rather weak condition on the moment generating function is enough to partially reproduce some of the bounds that we have proved for sub-Gaussian random variables. Observe that for [math]X \sim \Lap(1)[/math]
In particular, the moment generating function of the Laplace distribution is bounded by that of a Gaussian in a neighborhood of 0 but does not even exist away from zero. It turns out that all distributions that have tails at most as heavy as that of a Laplace distribution satisfy such a property.
Let [math]X[/math] be a centered random variable such that [math]\p(|X| \gt t)\le 2e^{-2t/\lambda}[/math] for some [math]\lambda \gt 0[/math]. Then, for any positive integer [math]k\ge 1[/math],
This leads to the following definition
A random variable [math]X[/math] is said to be sub-exponential with parameter [math]\lambda[/math] (denoted [math]X \sim \subE(\lambda)[/math]) if [math]\E[X]=0[/math] and its moment generating function satisfies
A simple and useful example of of a sub-exponential random variable is given in the next lemma.
Let [math]X\sim\sg(\sigma^2)[/math] then the random variable [math]Z=X^2-\E[X^2][/math] is sub-exponential: [math]Z\sim \subE(16\sigma^2)[/math].
We have, by the dominated convergence theorem,
Sub-exponential random variables also give rise to exponential deviation inequalities such as Corollary (Chernoff bound) or Theorem (Hoeffding's inequality) for weighted sums of independent sub-exponential random variables. The significant difference here is that the larger deviations are controlled in by a weaker bound.
Bernstein's inequality
Let [math]X_1,\ldots,X_n[/math] be independent random variables such that [math]\E(X_i) = 0[/math] and [math]X_i \sim \subE(\lambda)[/math]. Define
Without loss of generality, assume that [math]\lambda=1[/math] (we can always replace [math]X_i[/math] by [math]X_i/\lambda[/math] and [math]t[/math] by [math]t/\lambda[/math]). Next, using a Chernoff bound, we get for any [math]s \gt 0[/math]
Note that usually, Bernstein's inequality refers to a slightly more precise result that is qualitatively the same as the one above: it exhibits a Gaussian tail [math]e^{-nt^2/(2\lambda^2)}[/math] and an exponential tail [math]e^{-nt/(2\lambda)}[/math]. See for example Theorem2.10 in [1].
Maximal inequalities
The exponential inequalities of the previous section are valid for linear combinations of independent random variables, and, in particular, for the average [math]\bar X[/math]. In many instances, we will be interested in controlling the maximum over the parameters of such linear combinations (this is because of empirical risk minimization). The purpose of this section is to present such results.
Maximum over a finite set
We begin by the simplest case possible: the maximum over a finite set.
Let [math]X_1, \ldots, X_N[/math] be [math]N[/math] random variables such that
For any [math]s \gt 0[/math],
Extending these results to a maximum over an infinite set may be impossible. For example, if one is given an infinite sequence of \i.i.d [math]\cN(0, \sigma^2)[/math] random variables [math]X_1, X_2, \ldots[/math], then for any [math]N \ge 1[/math], we have for any [math]t \gt 0[/math],
On the opposite side of the picture, if all the [math]X_i[/math]s are equal to the same random variable [math]X[/math], we have for any [math]t \gt 0[/math],
In the Gaussian case, lower bounds are also available. They illustrate the effect of the correlation between the [math]X_i[/math]s. Examples from statistics have structure and we encounter many examples where a maximum of random variables over an infinite set is in fact finite. This is due to the fact that the random variables that we are considering are not independent of each other. In the rest of this section, we review some of these examples.
Maximum over a convex polytope
We use the definition of a polytope from [2]: a convex polytope [math]\mathsf{P}[/math] is a compact set with a finite number of vertices [math]\cV(\mathsf{P})[/math] called extreme points. It satisfies [math]\mathsf{P}=\conv(\cV(\mathsf{P}))[/math], where [math]\conv(\cV(\mathsf{P}))[/math] denotes the convex hull of the vertices of [math]\mathsf{P}[/math]. Let [math]X \in \R^d[/math] be a random vector and consider the (infinite) family of random variables
where [math]\mathsf{P} \subset \R^d[/math] is a polytope with [math]N[/math] vertices. While the family [math]\cF[/math] is infinite, the maximum over [math]\cF[/math] can be reduced to the a finite maximum using the following useful lemma.
Consider a linear form [math]x \mapsto c^\top x[/math], [math]x, c \in \R^d[/math]. Then for any convex polytope [math]\mathsf{P} \subset \R^d[/math],
Assume that [math]\cV(\mathsf{P})=\{v_1, \ldots, v_N\}[/math]. For any [math]x \in \mathsf{P}=\conv(\cV(\mathsf{P}))[/math], there exist nonnegative numbers [math]\lambda_1, \ldots \lambda_N[/math] that sum up to 1 and such that [math]x=\lambda_1 v_1 + \cdots + \lambda_N v_N[/math]. Thus
It immediately yields the following theorem
Let [math]\mathsf{P}[/math] be a polytope with [math]N[/math] vertices [math]v^{(1)}, \ldots, v^{(N)} \in \R^d[/math] and let [math]X \in \R^d[/math] be a random vector such that [math][v^{(i)}]^\top X[/math], [math]i=1, \ldots, N[/math], are sub-Gaussian random variables with variance proxy [math]\sigma^2[/math]. Then
Of particular interest are polytopes that have a small number of vertices. A primary example is the [math]\ell_1[/math] ball of [math]\R^d[/math] defined by
Indeed, it has exactly [math]2d[/math] vertices.
Maximum over the [math]\ell_2[/math] ball
Recall that the unit [math]\ell_2[/math] ball of [math]\R^d[/math] is defined by the set of vectors [math]u[/math] that have Euclidean norm [math]|u|_2[/math] at most 1. Formally, it is defined by
Clearly, this ball is not a polytope and yet, we can control the maximum of random variables indexed by [math]\cB_2[/math]. This is due to the fact that there exists a finite subset of [math]\cB_2[/math] such that the maximum over this finite set is of the same order as the maximum over the entire ball.
Fix [math]K\subset \R^d[/math] and [math]\eps \gt 0[/math]. A set [math]\cN[/math] is called an [math]\eps[/math]-net of [math]K[/math] with respect to a distance [math]d(\cdot, \cdot)[/math] on [math]\R^d[/math], if [math]\cN \subset K[/math] and for any [math]z \in K[/math], there exists [math]x \in \cN[/math] such that [math]d(x,z)\le \eps[/math].
Therefore, if [math]\cN[/math] is an [math]\eps[/math]-net of [math]K[/math] with respect to a norm [math]\|\cdot\|[/math], then every point of [math]K[/math] is at distance at most [math]\eps[/math] from a point in [math]\cN[/math]. Clearly, every compact set admits a finite [math]\eps[/math]-net. The following lemma gives an upper bound on the size of the smallest [math]\eps[/math]-net of [math]\cB_2[/math].
Fix [math]\eps\in(0,1)[/math]. Then the unit Euclidean ball [math]\cB_2[/math] has an [math]\eps[/math]-net [math]\cN[/math] with respect to the Euclidean distance of cardinality [math]|\cN|\le (3/\eps)^d[/math]
Consider the following iterative construction if the [math]\eps[/math]-net. Choose [math]x_1=0[/math]. For any [math]i\ge 2[/math], take [math]x_i[/math] to be any [math]x \in \cB_2[/math] such that [math]|x -x_j|_2 \gt \eps[/math] for all [math]j \lt i[/math]. If no such [math]x[/math] exists, stop the procedure. Clearly, this will create an [math]\eps[/math]-net. We now control its size. Observe that since [math]|x -y|_2 \gt \eps[/math] for all [math]x,y \in \cN[/math], the Euclidean balls centered at [math]x \in \cN[/math] and with radius [math]\eps/2[/math] are disjoint. Moreover,
Let [math]X \in \R^d[/math] be a sub-Gaussian random vector with variance proxy [math]\sigma^2[/math]. Then
Let [math]\cN[/math] be a [math]1/2[/math]-net of [math]\cB_2[/math] with respect to the Euclidean norm that satisfies [math]|\cN|\le 6^d[/math]. Next, observe that for every [math]\theta \in \cB_2[/math], there exists [math]z \in \cN[/math] and [math]x[/math] such that [math]|x|_2\le 1/2[/math] and [math]\theta=z+x[/math]. Therefore,
\medskip The bound with high probability then follows because
Sums of independent random matrices
In this section, we are going to explore how concentration statements can be extended to sums of matrices. As an example, we are going to show a version of Bernstein's inequality, for sums of independent matrices, closely following the presentation in [3], which builds on previous work in [4]. Results of this type have been crucial in providing guarantees for low rank recovery, see for example [5]. In particular, we want to control the maximum eigenvalue of a sum of independent random symmetric matrices [math] \p(\lambda_{\mathrm{max}}(\sum_i \bX_i) \gt t)[/math]. The tools involved will closely mimic those used for scalar random variables, but with the caveat that care must be taken when handling exponentials of matrices because [math] e^{A + B} \neq e^{A} e^{B} [/math] if [math] A, B \in \R^d [/math] and [math] AB \neq BA [/math].
Preliminaries
We denote the set of symmetric, positive semi-definite, and positive definite matrices in [math] \R^{d \times d} [/math] by [math] \cS_d, \cS_d^{+} [/math], and [math] \cS_d^{++} [/math], respectively, and will omit the subscript when the dimensionality is clear. Here, positive semi-definite means that for a matrix [math] \bX \in \cS^+ [/math], [math] v^\top \bX v \geq 0 [/math] for all [math] v \in \R^d [/math], [math] |v|_2 = 1 [/math], and positive definite means that the equality holds strictly. This is equivalent to all eigenvalues of [math] \bX [/math] being larger or equal (strictly larger, respectively) than 0, [math] \lambda_j(\bX) \geq 0 [/math] for all [math] j = 1, \dots, d [/math]. The cone of positive definite matrices induces an order on matrices by setting [math] \bA \preceq \bB [/math] if [math] \bB - \bA \in \cS^+ [/math]. Since we want to extend the notion of exponentiating random variables that was essential to our derivation of the Chernoff bound to matrices, we will make use of the matrix exponential and the matrix logarithm. For a symmetric matrix [math] \bX [/math], we can define a functional calculus by applying a function [math] f : \R \to \R [/math] to the diagonal elements of its spectral decomposition, \ie, if [math] \bX = \bQ \Lambda \bQ^\top [/math], then
where
is only non-zero on the diagonal and [math] f(\bX) [/math] is well-defined because the spectral decomposition is unique up to the ordering of the eigenvalues and the basis of the eigenspaces, with respect to both of which \eqref{eq:funccalc} is invariant, as well. From the definition, it is clear that for the spectrum of the resulting matrix,
While inequalities between functions do not generally carry over to matrices, we have the following transfer rule:
We can now define the matrix exponential by \eqref{eq:funccalc} which is equivalent to defining it via a power series,
Similarly, we can define the matrix logarithm as the inverse function of [math] \exp [/math] on [math] \cS [/math], [math] \log(e^{A}) = A [/math], which defines it on [math] \cS^+ [/math]. Some nice properties of these functions on matrices are their monotonicity: For [math] \bA \preceq \bB [/math],
and for [math] 0 \prec \bA \preceq \bB [/math],
Note that the analog of \eqref{eq:i} is in general not true for the matrix exponential.
The Laplace transform method
For the remainder of this section, let [math] \bX_1, \dots, \bX_n \in \R^{d \times d} [/math] be independent random symmetric matrices. As a first step that can lead to different types of matrix concentration inequalities, we give a generalization of the Chernoff bound for the maximum eigenvalue of a matrix.
Let [math] \bY [/math] be a random symmetric matrix. Then, for all [math] t \in \R [/math],
{{{4}}}
Recall that the crucial step in proving Bernstein's and Hoeffding's inequality was to exploit the independence of the summands by the fact that the exponential function turns products into sums.
This property, [math] e^{A + B} = e^{A}e^{B} [/math], no longer holds true for matrices, unless they commute. We could try to replace it with a similar property, the Golden-Thompson inequality,
Unfortunately, this does not generalize to more than two matrices, and when trying to peel off factors, we would have to pull a maximum eigenvalue out of the trace,
This is the approach followed by Ahlswede-Winter [4], which leads to worse constants for concentration than the ones we obtain below. Instead, we are going to use the following deep theorem due to Lieb [6]. A sketch of the proof can be found in the appendix of [7].
Let [math] \bH \in \R^{d \times d} [/math] be symmetric. Then,
Let [math] \bX, \bH \in \mathcal{S}_d [/math] be two symmetric matrices such that [math] \bX [/math] is random and [math] \bH [/math] is fixed. Then,
{{{4}}}
With this, we can establish a better bound on the moment generating function of sums of independent matrices.
Let [math] \bX_1, \dots, \bX_n [/math] be [math] n [/math] independent, random symmetric matrices. Then,
Without loss of generality, we can assume [math] \theta = 1 [/math]. Write [math] \E_k [/math] for the expectation conditioned on [math] \bX_1, \dots, \bX_k [/math], [math] \E_k[\,.\,] = \E[\,.\,|\bX_1, \dots, \bX_k] [/math]. By the tower property,
Tail bounds for sums of independent matrices
For all [math] t \in \R [/math],
Combine Lemma with Proposition.
Assume that there is a function [math] g : (0, \infty) \to [0, \infty] [/math] and fixed symmetric matrices [math] \bA_i [/math] such that [math] \E[e^{\theta \bX_i}] \preceq e^{g(\theta) \bA_i} [/math] for all [math] i [/math]. Set
Using the operator monoticity of [math] \log [/math], \eqref{eq:i}, and the monotonicity of [math] \tr \exp [/math], \eqref{eq:h}, we can plug the estimates for the matrix mgfs into the master inequality, Theorem Theorem, to obtain
Now we are ready to prove Bernstein's inequality We just need to come up with a dominating function for Corollary Corollary.
If [math] \E[\bX] = 0 [/math] and [math] \lambda_{\mathrm{max}}(\bX) \leq 1 [/math], then
Define
Assume [math] \E \bX_i = 0 [/math] and [math] \lambda_{\mathrm{max}}(\bX_i) \leq R [/math] almost surely for all [math] i [/math] and set
Without loss of generality, take [math] R = 1 [/math]. By Lemma,
With similar techniques, one can also prove a version of Hoeffding's inequality for matrices, see [3](Theorem 1.3).
General references
Rigollet, Philippe; Hütter, Jan-Christian (2023). "High-Dimensional Statistics". arXiv:2310.19244 [math.ST].
Notes
References
- Boucheron, St\'ephane; Lugosi, G\'abor; Massart, Pascal (2013). Concentration inequalities. 13. Oxford: Oxford University Press. pp. x+481. ISBN 0-521-38991-7.
- Grunbaum, Branko (2003). Convex polytopes. 221. New York: Springer-Verlag. pp. xvi+468. ISBN 0-387-40409-0.
- 3.0 3.1 Tropp, Joel A. (2012). "User-Friendly Tail Bounds for Sums of Random Matrices". Foundations of computational mathematics 12: 389--434. Springer-Verlag. doi: .
- 4.0 4.1 "Strong Converse for Identification via Quantum Channels" (2002). IEEE Transactions on Information Theory 48: 569--579. Springer-Verlag. doi: .
- Gross, David (2011). "Recovering Low-Rank Matrices from Few Coefficients in Any Basis". IEEE Transactions on Information Theory 57: 1548--1566. Springer-Verlag. doi: .
- Lieb, Elliott H. (1973). "Convex Trace Functions and the Wigner-Yanase-Dyson Conjecture". Advances in Mathematics 11: 267--288. Springer-Verlag. doi: .
- Ruskai, Mary Beth (2002). "Inequalities for Quantum Entropy: A Review with Conditions for Equality". Journal of Mathematical Physics 43: 4358--4375. Springer-Verlag. doi: .