guide:Ec36399528: Difference between revisions
No edit summary |
mNo edit summary |
||
(5 intermediate revisions by the same user not shown) | |||
Line 127: | Line 127: | ||
\newcommand{\outrn}[1]{\mathcal{O}_{\sP_n}[#1]} | \newcommand{\outrn}[1]{\mathcal{O}_{\sP_n}[#1]} | ||
\newcommand{\email}[1]{\texttt{#1}} | \newcommand{\email}[1]{\texttt{#1}} | ||
\newcommand{\possessivecite}[1]{ | \newcommand{\possessivecite}[1]{\citeauthor{#1}'s \citeyear{#1}} | ||
\newcommand\xqed[1]{% | \newcommand\xqed[1]{% | ||
\leavevmode\unskip\penalty9999 \hbox{}\nobreak\hfill | \leavevmode\unskip\penalty9999 \hbox{}\nobreak\hfill | ||
Line 147: | Line 147: | ||
</math> | </math> | ||
</div> | </div> | ||
The literature reviewed in this chapter starts with the analysis of what can be learned about functionals of probability distributions that are well-defined in the absence of a model. | The literature reviewed in this chapter starts with the analysis of what can be learned about functionals of probability distributions that are well-defined in the absence of a model. | ||
The approach is nonparametric, and it is typically ''constructive'', in the sense that it leads to | The approach is nonparametric, and it is typically ''constructive'', in the sense that it leads to “plug-in” formulae for the bounds on the functionals of interest. | ||
==<span id="subsec:missing_data"></span>Selectively Observed Data== | |||
As in <ref name="man89"></ref>, suppose that a researcher is interested in learning the probability that an individual who is homeless at a given date has a home six months later. | As in <ref name="man89"><span style="font-variant-caps:small-caps">Manski, C.F.</span> (1989): “Anatomy of the Selection Problem” ''The Journal of Human Resources'', 24(3), 343--360.</ref>, suppose that a researcher is interested in learning the probability that an individual who is homeless at a given date has a home six months later. | ||
Here the population of interest is the people who are homeless at the initial date, and the outcome of interest <math>\ey</math> is an indicator of whether the individual has a home six months later (so that <math>\ey=1</math>) or remains homeless (so that <math>\ey=0</math>). | Here the population of interest is the people who are homeless at the initial date, and the outcome of interest <math>\ey</math> is an indicator of whether the individual has a home six months later (so that <math>\ey=1</math>) or remains homeless (so that <math>\ey=0</math>). | ||
A random sample of homeless individuals is interviewed at the initial date, so that individual background attributes <math>\ex</math> are observed, but six months later only a subset of the individuals originally sampled can be located. | A random sample of homeless individuals is interviewed at the initial date, so that individual background attributes <math>\ex</math> are observed, but six months later only a subset of the individuals originally sampled can be located. | ||
Line 158: | Line 158: | ||
Let <math>\ed</math> be an indicator of whether the individual can be located (hence <math>\ed=1</math>) or not (hence <math>\ed=0</math>). | Let <math>\ed</math> be an indicator of whether the individual can be located (hence <math>\ed=1</math>) or not (hence <math>\ed=0</math>). | ||
The question is what can the researcher learn about <math>\E_\sQ(\ey|\ex=x)</math>, with <math>\sQ</math> the distribution of <math>(\ey,\ex)</math>? | The question is what can the researcher learn about <math>\E_\sQ(\ey|\ex=x)</math>, with <math>\sQ</math> the distribution of <math>(\ey,\ex)</math>? | ||
<ref name="man89" | <ref name="man89"/> showed that <math>\E_\sQ(\ey|\ex=x)</math> is not point identified in the absence of additional assumptions, but informative nonparametric bounds on this quantity can be obtained. | ||
In this section I review his approach, and discuss several important extensions of his original idea. | In this section I review his approach, and discuss several important extensions of his original idea. | ||
Throughout the chapter, I formally state the structure of the problem under study as an | Throughout the chapter, I formally state the structure of the problem under study as an “Identification Problem”, and then provide a solution, either in the form of a sharp identification region, or of an outer region. | ||
To set the stage, and at the cost of some repetition, I do the same here, slightly generalizing the question stated in the previous paragraph. | To set the stage, and at the cost of some repetition, I do the same here, slightly generalizing the question stated in the previous paragraph. | ||
{{proofcard|Identification Problem (Conditional Expectation of Selectively Observed Data)|IP:bounds:mean:md| | |||
Let <math>\ey \in \mathcal{Y}\subset \R</math> and <math>\ex \in \mathcal{X}\subset \R^d</math> be, respectively, an outcome variable and a vector of covariates with support <math>\cY</math> and <math>\cX</math> respectively, with <math>\cY</math> a compact set. | Let <math>\ey \in \mathcal{Y}\subset \R</math> and <math>\ex \in \mathcal{X}\subset \R^d</math> be, respectively, an outcome variable and a vector of covariates with support <math>\cY</math> and <math>\cX</math> respectively, with <math>\cY</math> a compact set. | ||
Let <math>\ed \in \{0,1\}</math>. | Let <math>\ed \in \{0,1\}</math>. | ||
Line 171: | Line 170: | ||
Let <math>y_{j}\in\cY</math> be such that <math>g(y_j)=g_j</math>, <math>j=0,1</math>.<ref group="Notes" >The bounds <math>g_0,g_1</math> and the values <math>y_0,y_1</math> at which they are attained may differ for different functions <math>g(\cdot)</math>.</ref> | Let <math>y_{j}\in\cY</math> be such that <math>g(y_j)=g_j</math>, <math>j=0,1</math>.<ref group="Notes" >The bounds <math>g_0,g_1</math> and the values <math>y_0,y_1</math> at which they are attained may differ for different functions <math>g(\cdot)</math>.</ref> | ||
In the absence of additional information, what can the researcher learn about <math>\E_\sQ(g(\ey)|\ex=x)</math>, with <math>\sQ</math> the distribution of <math>(\ey,\ex)</math>? | In the absence of additional information, what can the researcher learn about <math>\E_\sQ(g(\ey)|\ex=x)</math>, with <math>\sQ</math> the distribution of <math>(\ey,\ex)</math>? | ||
|}} | |||
<ref name="man89" | <ref name="man89"/>’s analysis of this problem begins with a simple application of the law of total probability, that yields | ||
<math display="block"> | <math display="block"> | ||
Line 184: | Line 183: | ||
Hence, <math>\sQ(\ey|\ex=x)</math> is not point identified. | Hence, <math>\sQ(\ey|\ex=x)</math> is not point identified. | ||
If one were to assume ''exogenous selection'' (or data missing at random conditional on <math>\ex</math>), i.e., <math>\sR(\ey|\ex,\ed=0)=\sP(\ey|\ex,\ed=1)</math>, point identification would obtain. | If one were to assume ''exogenous selection'' (or data missing at random conditional on <math>\ex</math>), i.e., <math>\sR(\ey|\ex,\ed=0)=\sP(\ey|\ex,\ed=1)</math>, point identification would obtain. | ||
However, that assumption is non-refutable and it is well known that it may fail in [[guide:7b0105e1fc#sec:misspec | | However, that assumption is non-refutable and it is well known that it may fail in applications <ref group="Notes" >[[guide:7b0105e1fc#sec:misspec |Section]] discusses the consequences of model misspecification (with respect to refutable assumptions)</ref>. | ||
Let <math>\cT</math> denote the space of all probability measures with support in <math>\cY</math>. The unknown functional vector is <math>\{\tau(x),\upsilon(x)\}\equiv \{\sQ(\ey|\ex=x),\sR(\ey|\ex=x,\ed=0)\}</math>. | Let <math>\cT</math> denote the space of all probability measures with support in <math>\cY</math>. The unknown functional vector is <math>\{\tau(x),\upsilon(x)\}\equiv \{\sQ(\ey|\ex=x),\sR(\ey|\ex=x,\ed=0)\}</math>. | ||
What the researcher can learn, in the absence of additional restrictions on <math>\sR(\ey|\ex=x,\ed=0)</math>, is the region of ''observationally equivalent'' distributions for <math>\ey|\ex=x</math>, and the associated set of expectations taken with respect to these distributions. | What the researcher can learn, in the absence of additional restrictions on <math>\sR(\ey|\ex=x,\ed=0)</math>, is the region of ''observationally equivalent'' distributions for <math>\ey|\ex=x</math>, and the associated set of expectations taken with respect to these distributions. | ||
{{proofcard|Theorem (Conditional Expectations of Selectively Observed Data)|SIR:prob:E:md|Under the assumptions in Identification [[#IP:bounds:mean:md |Problem]], | |||
Under the assumptions in Identification [[#IP:bounds:mean:md |Problem]], | |||
<math display="block"> | <math display="block"> | ||
Line 198: | Line 196: | ||
</math> | </math> | ||
is the sharp identification region for <math>\E_\sQ(g(\ey)|\ex=x)</math>. | is the sharp identification region for <math>\E_\sQ(g(\ey)|\ex=x)</math>. | ||
| | |||
Due to the discussion following equation \eqref{eq:LTP_md}, the collection of observationally equivalent distribution functions for <math>\ey|\ex=x</math> is | Due to the discussion following equation \eqref{eq:LTP_md}, the collection of observationally equivalent distribution functions for <math>\ey|\ex=x</math> is | ||
Line 210: | Line 208: | ||
Next, observe that the lower bound in equation \eqref{eq:bounds:mean:md} is achieved by integrating <math>g(\ey)</math> against the distribution <math>\tau(x)</math> that results when <math>\upsilon(x)</math> places probability one on <math>y_0</math>. The upper bound is achieved by integrating <math>g(\ey)</math> against the distribution <math>\tau(x)</math> that results when <math>\upsilon(x)</math> places probability one on <math>y_1</math>. | Next, observe that the lower bound in equation \eqref{eq:bounds:mean:md} is achieved by integrating <math>g(\ey)</math> against the distribution <math>\tau(x)</math> that results when <math>\upsilon(x)</math> places probability one on <math>y_0</math>. The upper bound is achieved by integrating <math>g(\ey)</math> against the distribution <math>\tau(x)</math> that results when <math>\upsilon(x)</math> places probability one on <math>y_1</math>. | ||
Both are contained in the set <math>\idr{\sQ(\ey|\ex=x)}</math> in equation \eqref{eq:Tau_md}. | Both are contained in the set <math>\idr{\sQ(\ey|\ex=x)}</math> in equation \eqref{eq:Tau_md}. | ||
}} | |||
These are the ''worst case bounds'', so called because assumptions free and therefore representing the widest possible range of values for the parameter of interest that are consistent with the observed data. | These are the ''worst case bounds'', so called because assumptions free and therefore representing the widest possible range of values for the parameter of interest that are consistent with the observed data. | ||
A simple | A simple “plug-in” estimator for <math>\idr{\E_\sQ(g(\ey)|\ex=x)}</math> replaces all unknown quantities in \eqref{eq:bounds:mean:md} with consistent estimators, obtained, e.g., by kernel or sieve regression. | ||
I return to consistent estimation of partially identified parameters in [[guide:6d1a428897#sec:inference |Section]]. | I return to consistent estimation of partially identified parameters in [[guide:6d1a428897#sec:inference |Section]]. | ||
Here I emphasize that identification problems are fundamentally distinct from finite sample inference problems. | Here I emphasize that identification problems are fundamentally distinct from finite sample inference problems. | ||
The latter are typically reduced as sample size increase (because, e.g., the variance of the estimator becomes smaller). | The latter are typically reduced as sample size increase (because, e.g., the variance of the estimator becomes smaller). | ||
The former do not improve, unless a different and better type of data is collected, e.g. with a smaller prevalence of missing data (see <ref name="dom:man17"></ref>{{rp|at=for a discussion}}). | The former do not improve, unless a different and better type of data is collected, e.g. with a smaller prevalence of missing data (see <ref name="dom:man17"><span style="font-variant-caps:small-caps">Dominitz, J., <span style="font-variant-caps:normal">and</span> C.F. Manski</span> (2017): “More Data or Better Data? A Statistical Decision Problem” ''The Review of Economic Studies'', 84(4), 1583--1605.</ref>{{rp|at=for a discussion}}). | ||
<ref name="man03"></ref>{{rp|at=Section 1.3}} shows that the proof of Theorem [[#SIR:prob:E:md |SIR-]] can be extended to obtain the smallest and largest points in the sharp identification region of any parameter that respects stochastic dominance.<ref group="Notes" > | <ref name="man03"><span style="font-variant-caps:small-caps">Manski, C.F.</span> (2003): ''Partial Identification of Probability Distributions'', Springer Series in Statistics. Springer.</ref>{{rp|at=Section 1.3}} shows that the proof of Theorem [[#SIR:prob:E:md |SIR-]] can be extended to obtain the smallest and largest points in the sharp identification region of any parameter that respects stochastic dominance.<ref group="Notes" > | ||
Recall that a probability distribution <math>\sF\in\cT</math> stochastically dominates <math>\sF^\prime\in\cT</math> if <math>\sF(-\infty,t]\le \sF^\prime(-\infty,t]</math> for all <math>t\in\R</math>. A real-valued functional <math>\sd:\cT\to\R</math> respects stochastic dominance if <math>\sd(\sF)\ge \sd(\sF^\prime)</math> whenever <math>\sF</math> stochastically dominates <math>\sF^\prime</math>.</ref> | Recall that a probability distribution <math>\sF\in\cT</math> stochastically dominates <math>\sF^\prime\in\cT</math> if <math>\sF(-\infty,t]\le \sF^\prime(-\infty,t]</math> for all <math>t\in\R</math>. A real-valued functional <math>\sd:\cT\to\R</math> respects stochastic dominance if <math>\sd(\sF)\ge \sd(\sF^\prime)</math> whenever <math>\sF</math> stochastically dominates <math>\sF^\prime</math>.</ref> | ||
This is especially useful to bound the quantiles of <math>\ey|\ex=x</math>. | This is especially useful to bound the quantiles of <math>\ey|\ex=x</math>. | ||
Line 242: | Line 240: | ||
By comparison, for any value of <math>\alpha</math>, <math>r(\alpha,x)</math> and <math>s(\alpha,x)</math> are | By comparison, for any value of <math>\alpha</math>, <math>r(\alpha,x)</math> and <math>s(\alpha,x)</math> are | ||
generically informative if, respectively, <math>\sP(\ed=1|\ex=x) > 1-\alpha</math> and <math>\sP(\ed=1|\ex=x) \ge \alpha</math>, regardless of the range of <math>g</math>. | generically informative if, respectively, <math>\sP(\ed=1|\ex=x) > 1-\alpha</math> and <math>\sP(\ed=1|\ex=x) \ge \alpha</math>, regardless of the range of <math>g</math>. | ||
<ref name="sto10"></ref> further extends partial identification analysis to the study of spread parameters in the presence of missing data (as well as interval data, data combinations, and other applications). | <ref name="sto10"><span style="font-variant-caps:small-caps">Stoye, J.</span> (2010): “Partial identification of spread parameters” ''Quantitative Economics'', 1(2), 323--357.</ref> further extends partial identification analysis to the study of spread parameters in the presence of missing data (as well as interval data, data combinations, and other applications). | ||
These parameters include ones that respect second order stochastic dominance, such as the variance, the Gini coefficient, and other inequality measures, as well as other measures of dispersion which do not respect second order stochastic dominance, such as interquartile range and ratio.<ref group="Notes" > | These parameters include ones that respect second order stochastic dominance, such as the variance, the Gini coefficient, and other inequality measures, as well as other measures of dispersion which do not respect second order stochastic dominance, such as interquartile range and ratio.<ref group="Notes" > | ||
Earlier related work includes, e.g., | Earlier related work includes, e.g., {{ref|name=gas72}} and {{ref|name=cow91}}, who obtain worst case bounds on the sample Gini coefficient under the assumption that one knows the income bracket but not the exact income of every household.</ref> | ||
<ref name="sto10" | <ref name="sto10"/> shows that the sharp identification region for these parameters can be obtained by fixing the mean or quantile of the variable of interest at a specific value within its sharp identification region, and deriving a distribution consistent with this value which is ``compressed" with respect to the ones which bound the cumulative distribution function (CDF) of the variable of interest, and one which is ``dispersed" with respect to them. | ||
Heuristically, the compressed distribution minimizes spread, while the dispersed one maximizes it (the sense in which this optimization occurs is formally defined in the paper). | Heuristically, the compressed distribution minimizes spread, while the dispersed one maximizes it (the sense in which this optimization occurs is formally defined in the paper). | ||
The intuition for this is that a compressed CDF is first below and then above any non-compressed one; a dispersed CDF is first above and then below any non-dispersed one. | The intuition for this is that a compressed CDF is first below and then above any non-compressed one; a dispersed CDF is first above and then below any non-dispersed one. | ||
Line 251: | Line 249: | ||
The main results of the paper are sharp identification regions for the expectation and variance, for the median and interquartile ratio, and for many other combinations of parameters. | The main results of the paper are sharp identification regions for the expectation and variance, for the median and interquartile ratio, and for many other combinations of parameters. | ||
'''Key Insight (Identification is not a binary event):''' | |||
<span id="big_idea:id_not_binary"/> | |||
Identification [[#IP:bounds:mean:md |Problem]] is mathematically simple, but it puts forward a new approach to empirical research. | <i>Identification [[#IP:bounds:mean:md |Problem]] is mathematically simple, but it puts forward a new approach to empirical research. | ||
The traditional approach aims at finding a sufficient (possibly minimal) set of assumptions guaranteeing point identification of parameters, viewing identification as an | The traditional approach aims at finding a sufficient (possibly minimal) set of assumptions guaranteeing point identification of parameters, viewing identification as an “all or nothing” notion, where either the functional of interest can be learned exactly or nothing of value can be learned. | ||
The partial identification approach pioneered by <ref name="man89" | The partial identification approach pioneered by <ref name="man89"/> points out that much can be learned from combination of data and assumptions that restrict the functionals of interest to a set of observationally equivalent values, even if this set is not a singleton. | ||
Along the way, <ref name="man89" | Along the way, <ref name="man89"/> points out that in Identification [[#IP:bounds:mean:md |Problem]] the observed outcome is the singleton <math>\ey</math> when <math>\ed=1</math>, and the set <math>\cY</math> when <math>\ed=0</math>. | ||
This is a random closed set, see [[guide:379e0dcd67#def:rcs |Definition]]. | This is a random closed set, see [[guide:379e0dcd67#def:rcs |Definition]]. | ||
I return to this connection in Section [[#subsec:interval_data |Interval Data]]. | I return to this connection in Section [[#subsec:interval_data |Interval Data]].</i> | ||
Despite how transparent the framework in Identification [[#IP:bounds:mean:md |Problem]] is, important subtleties arise even in this seemingly simple context. | Despite how transparent the framework in Identification [[#IP:bounds:mean:md |Problem]] is, important subtleties arise even in this seemingly simple context. | ||
For a given <math>t\in\R</math>, consider the function <math>g(\ey)=\one(\ey\le t)</math>, with <math>\one(A)</math> the indicator function taking the value one if the logical condition in parentheses holds and zero otherwise. | For a given <math>t\in\R</math>, consider the function <math>g(\ey)=\one(\ey\le t)</math>, with <math>\one(A)</math> the indicator function taking the value one if the logical condition in parentheses holds and zero otherwise. | ||
Line 271: | Line 269: | ||
\end{multline} | \end{multline} | ||
</math> | </math> | ||
Yet, the collection of CDFs that belong to the band defined by \eqref{eq:pointwise_bounds_F_md} is ''not'' the sharp identification region for the CDF of <math>\ey|\ex=x</math>. Rather, it constitutes an ''outer region'', as originally pointed out by <ref name="man94"></ref>{{rp|at=p. 149 and note 2}}. | Yet, the collection of CDFs that belong to the band defined by \eqref{eq:pointwise_bounds_F_md} is ''not'' the sharp identification region for the CDF of <math>\ey|\ex=x</math>. Rather, it constitutes an ''outer region'', as originally pointed out by <ref name="man94"><span style="font-variant-caps:small-caps">Manski, C.F.</span> (1994): “The selection problem” in ''Advances in Econometrics: Sixth World Congress'', ed. by C.A. Sims, vol.1 of ''Econometric Society Monographs'', pp. 143--170. Cambridge University Press.</ref>{{rp|at=p. 149 and note 2}}. | ||
{{proofcard|Theorem (Cumulative Distribution Function of Selectively Observed Data)|OR:CDF_md|Let <math>\cC</math> denote the collection of cumulative distribution functions on <math>\cY</math>. | |||
Let <math>\cC</math> denote the collection of cumulative distribution functions on <math>\cY</math>. | |||
Then, under the assumptions in Identification [[#IP:bounds:mean:md |Problem]], | Then, under the assumptions in Identification [[#IP:bounds:mean:md |Problem]], | ||
Line 285: | Line 282: | ||
</math> | </math> | ||
is an outer region for the CDF of <math>\ey|\ex=x</math>. | is an outer region for the CDF of <math>\ey|\ex=x</math>.| | ||
Any admissible CDF for <math>\ey|\ex=x</math> belongs to the family of functions in equation \eqref{eq:outer_cdf_md}. However, the bound in equation \eqref{eq:outer_cdf_md} does not impose the restriction that for any <math>t_0\le t_1</math>, | Any admissible CDF for <math>\ey|\ex=x</math> belongs to the family of functions in equation \eqref{eq:outer_cdf_md}. However, the bound in equation \eqref{eq:outer_cdf_md} does not impose the restriction that for any <math>t_0\le t_1</math>, | ||
Line 297: | Line 292: | ||
</math> | </math> | ||
This restriction is implied by the maintained assumptions, but is not necessarily satisfied by all CDFs in <math>\outr{\sF(\ey|\ex=x)}</math>, as illustrated in the following simple example. | This restriction is implied by the maintained assumptions, but is not necessarily satisfied by all CDFs in <math>\outr{\sF(\ey|\ex=x)}</math>, as illustrated in the following simple example. | ||
}} | |||
<div id="fig:boundsCDF:md" class="d-flex justify-content-center"> | <div id="fig:boundsCDF:md" class="d-flex justify-content-center"> | ||
[[File:guide_d9532_fig_boundsCDF_md.png | | [[File:guide_d9532_fig_boundsCDF_md.png | 700px | thumb | The tube defined by inequalities \eqref{eq:pointwise_bounds_F_md} in the set-up of [[#example:CDF_md |Example]], and the CDF in \eqref{eq:CDF_counterexample_md}. ]] | ||
</div> | </div> | ||
<span id="example:CDF_md"/> | |||
'''Example''' | |||
Omit <math>\ex</math> for simplicity, let <math>\sP(\ed=1)=\frac{2}{3}</math>, and let | Omit <math>\ex</math> for simplicity, let <math>\sP(\ed=1)=\frac{2}{3}</math>, and let | ||
<math display="block"> | <math display="block"> | ||
\sP(\ey\le t|\ed=1)\left\{ | \sP(\ey\le t|\ed=1)\left\{ | ||
\begin{ | \begin{array}{lll} | ||
0 & \textrm{if} & t < 0,\\ | |||
\frac{1}{3}t & \textrm{if} & 0\le t < 3,\\ | |||
1 & \textrm{if} & t\ge 3. | |||
\end{ | \end{array} | ||
\right. | \right. | ||
</math> | </math> | ||
The bounding functions and associated tube from the inequalities in \eqref{eq:pointwise_bounds_F_md} are depicted in [[#fig:boundsCDF:md|Figure]]. | The bounding functions and associated tube from the inequalities in \eqref{eq:pointwise_bounds_F_md} are depicted in [[#fig:boundsCDF:md|Figure]]. | ||
Consider the cumulative distribution function | Consider the cumulative distribution function | ||
Line 324: | Line 322: | ||
\sF(t)= | \sF(t)= | ||
\left\{ | \left\{ | ||
\begin{ | \begin{array}{lll} | ||
0 & \textrm{if}\,\, & t < 0,\\ | |||
\frac{5}{9}t & \textrm{if} & 0\le t < 1,\\ | |||
\frac{1}{9}t+\frac{4}{9} & \textrm{if} & 1\le t < 2,\\ | |||
\frac{1}{3}t & \textrm{if} & 2\le t < 3,\\ | |||
1 & \textrm{if} & t\ge 3. | |||
\end{ | \end{array} | ||
\right. | \right. | ||
\end{align} | \end{align} | ||
</math> | </math> | ||
For each <math>t\in\R</math>, <math>\sF(t)</math> lies in the tube defined by equation \eqref{eq:pointwise_bounds_F_md}. | For each <math>t\in\R</math>, <math>\sF(t)</math> lies in the tube defined by equation \eqref{eq:pointwise_bounds_F_md}. | ||
However, it cannot be the CDF of <math>\ey</math>, because <math>\sF(2)-\sF(1)=\frac{1}{9} < \sP(1\le\ey\le 2|\ed=1)\sP(\ed=1)</math>, directly contradicting equation \eqref{eq:CDF_md_Kinterval}. | However, it cannot be the CDF of <math>\ey</math>, because <math>\sF(2)-\sF(1)=\frac{1}{9} < \sP(1\le\ey\le 2|\ed=1)\sP(\ed=1)</math>, directly contradicting equation \eqref{eq:CDF_md_Kinterval}. | ||
How can one characterize the sharp identification region for the CDF of <math>\ey|\ex=x</math> under the assumptions in Identification [[#IP:bounds:mean:md |Problem]]? | How can one characterize the sharp identification region for the CDF of <math>\ey|\ex=x</math> under the assumptions in Identification [[#IP:bounds:mean:md |Problem]]? | ||
In general, there is not a single answer to this question: different methodologies can be used. | In general, there is not a single answer to this question: different methodologies can be used. | ||
Here I use results in <ref name="man03" | Here I use results in <ref name="man03"/>{{rp|at=Corollary 1.3.1}} and <ref name="mol:mol18"><span style="font-variant-caps:small-caps">Molchanov, I., <span style="font-variant-caps:normal">and</span> F.Molinari</span> (2018): ''Random Sets in Econometrics''. Econometric Society Monograph Series, Cambridge University Press, Cambridge UK.</ref>{{rp|at=Theorem 2.25}}, which yield an alternative characterization of <math>\idr{\sQ(\ey|\ex=x)}</math> that translates directly into a characterization of <math>\idr{\sF(\ey|\ex=x)}</math>.<ref group="Notes" >Whereas {{ref|name=man94}} is very clear that the collection of CDFs in \eqref{eq:pointwise_bounds_F_md} is an outer region for the CDF of <math>\ey|\ex=x</math>, and {{ref|name=man03}} provides the sharp characterization in \eqref{eq:sharp_id_P_md_Manski}, {{ref|name=man07a}}{{rp|at=p. 39}} does not state all the requirements that characterize <math>\idr{\sF(\ey|\ex=x)}</math>.</ref> | ||
{{proofcard|Theorem (Conditional Distribution and CDF of Selectively Observed Data)|SIR:CDF_md| | |||
Given <math>\tau\in\cT</math>, let <math>\tau_K(x)</math> denote the probability that distribution <math>\tau</math> assigns to set <math>K</math> conditional on <math>\ex=x</math>, with <math>\tau_y(x)\equiv\tau_{\{y\}}(x)</math>. | Given <math>\tau\in\cT</math>, let <math>\tau_K(x)</math> denote the probability that distribution <math>\tau</math> assigns to set <math>K</math> conditional on <math>\ex=x</math>, with <math>\tau_y(x)\equiv\tau_{\{y\}}(x)</math>. | ||
Under the assumptions in Identification [[#IP:bounds:mean:md |Problem]], | Under the assumptions in Identification [[#IP:bounds:mean:md |Problem]], | ||
Line 367: | Line 365: | ||
\end{multline} | \end{multline} | ||
</math> | </math> | ||
| | |||
The characterization in \eqref{eq:sharp_id_P_md_Manski} follows from equation \eqref{eq:Tau_md}, observing that if <math>\tau(x)\in\idr{\sQ(\ey|\ex=x)}</math> as defined in equation \eqref{eq:Tau_md}, then there exists a distribution <math>\upsilon(x)\in\cT</math> such that <math>\tau(x) = \sP(\ey|\ex=x,\ed=1)\sP(\ed=1|\ex=x)+\upsilon(x)\sP(\ed=0|\ex=x)</math>. | The characterization in \eqref{eq:sharp_id_P_md_Manski} follows from equation \eqref{eq:Tau_md}, observing that if <math>\tau(x)\in\idr{\sQ(\ey|\ex=x)}</math> as defined in equation \eqref{eq:Tau_md}, then there exists a distribution <math>\upsilon(x)\in\cT</math> such that <math>\tau(x) = \sP(\ey|\ex=x,\ed=1)\sP(\ed=1|\ex=x)+\upsilon(x)\sP(\ed=0|\ex=x)</math>. | ||
Hence, by construction <math>\tau_K(x) \ge \sP(\ey\in K|\ex=x,\ed=1)\sP(\ed=1|\ex=x)</math>, <math>\forall K\subset \cY</math>. Conversely, if one has <math>\tau_K(x) \ge \sP(\ey\in K|\ex=x,\ed=1)\sP(\ed=1|\ex=x)</math>, <math>\forall K\subset \cY</math>, one can define <math>\upsilon(x)=\frac{\tau(x) - \sP(\ey|\ex=x,\ed=1)\sP(\ed=1|\ex=x)}{\sP(\ed=0|\ex=x)}</math>. | Hence, by construction <math>\tau_K(x) \ge \sP(\ey\in K|\ex=x,\ed=1)\sP(\ed=1|\ex=x)</math>, <math>\forall K\subset \cY</math>. Conversely, if one has <math>\tau_K(x) \ge \sP(\ey\in K|\ex=x,\ed=1)\sP(\ed=1|\ex=x)</math>, <math>\forall K\subset \cY</math>, one can define <math>\upsilon(x)=\frac{\tau(x) - \sP(\ey|\ex=x,\ed=1)\sP(\ed=1|\ex=x)}{\sP(\ed=0|\ex=x)}</math>. | ||
Line 380: | Line 378: | ||
\end{multline} | \end{multline} | ||
</math> | </math> | ||
The result in equation \eqref{eq:sharp_id_P_md_interval} is proven in <ref name="mol:mol18" | The result in equation \eqref{eq:sharp_id_P_md_interval} is proven in <ref name="mol:mol18"/>{{rp|at=Theorem 2.25}} using elements of random set theory, to which I return in Section [[#subsec:interval_data |Interval Data]]. | ||
Using elements of random set theory it is also possible to show that the characterization in \eqref{eq:sharp_id_P_md_Manski} requires only to check the inequalities for <math>K</math> the compact subsets of <math>\cY</math>. | Using elements of random set theory it is also possible to show that the characterization in \eqref{eq:sharp_id_P_md_Manski} requires only to check the inequalities for <math>K</math> the compact subsets of <math>\cY</math>. | ||
}} | |||
This section provides sharp identification regions and outer regions for a variety of functionals of interest. | This section provides sharp identification regions and outer regions for a variety of functionals of interest. | ||
The computational complexity of these characterizations varies widely. | The computational complexity of these characterizations varies widely. | ||
Line 389: | Line 387: | ||
A sharp identification region on the CDF requires evaluating the probability that a certain distribution assigns to all intervals. | A sharp identification region on the CDF requires evaluating the probability that a certain distribution assigns to all intervals. | ||
I return to computational challenges in partial identification in [[guide:A85a6b6ff1#sec:computations |Section]]. | I return to computational challenges in partial identification in [[guide:A85a6b6ff1#sec:computations |Section]]. | ||
==<span id="subsec:programme:eval"></span>Treatment Effects with and without Instrumental Variables== | |||
The discussion of partial identification of probability distributions of selectively observed data naturally leads to the question of its implications for program evaluation. | The discussion of partial identification of probability distributions of selectively observed data naturally leads to the question of its implications for program evaluation. | ||
The literature on program evaluation is vast. | The literature on program evaluation is vast. | ||
Line 396: | Line 395: | ||
To keep this chapter to a manageable length, I discuss only partial identification of the average response to a treatment and of the average treatment effect (ATE). | To keep this chapter to a manageable length, I discuss only partial identification of the average response to a treatment and of the average treatment effect (ATE). | ||
There are many different parameters that received much interest in the literature. | There are many different parameters that received much interest in the literature. | ||
Examples include the ''local average treatment effect'' of <ref name="imb:ang94"></ref> and the ''marginal treatment effect'' of | Examples include the ''local average treatment effect'' of <ref name="imb:ang94"><span style="font-variant-caps:small-caps">Imbens, G.W., <span style="font-variant-caps:normal">and</span> J.D. Angrist</span> (1994): “Identification and Estimation of Local Average Treatment Effects” ''Econometrica'', 62(2), 467--475.</ref> and the ''marginal treatment effect'' of | ||
<ref name="hec:vyt99"></ref><ref name="hec:vyt01"></ref><ref name="hec:vyt05"></ref>. | <ref name="hec:vyt99"><span style="font-variant-caps:small-caps">Heckman, J.J., <span style="font-variant-caps:normal">and</span> E.J. Vytlacil</span> (1999): “Local Instrumental Variables and Latent Variable Models for Identifying and Bounding Treatment Effects” ''Proceedings of the National Academy of Sciences of the United States of America'', 96(8), 4730--4734.</ref><ref name="hec:vyt01"><span style="font-variant-caps:small-caps">Heckman, J.J., <span style="font-variant-caps:normal">and</span> E.J. Vytlacil</span> (2001): “Instrumental variables, selection models, and tight bounds on the average treatment effect” in ''Econometric Evaluation of Labour Market Policies'', ed. by M.Lechner, <span style="font-variant-caps:normal">and</span> F.Pfeiffer, pp. 1--15, Heidelberg. Physica-Verlag HD.</ref><ref name="hec:vyt05"><span style="font-variant-caps:small-caps">Heckman, J.J., <span style="font-variant-caps:normal">and</span> E.J. Vytlacil</span> (2005): “Structural Equations, Treatment Effects, and Econometric Policy Evaluation” ''Econometrica'', 73(3), 669--738.</ref>. | ||
For thorough discussions of the literature on program evaluation, I refer to the textbook treatments in <ref name="man95"></ref><ref name="man03" | For thorough discussions of the literature on program evaluation, I refer to the textbook treatments in <ref name="man95"><span style="font-variant-caps:small-caps">Manski, C.F.</span> (1995): ''Identification Problems in the Social Sciences''. Harvard University Press.</ref><ref name="man03"/><ref name="man07a"><span style="font-variant-caps:small-caps">Manski, C.F.</span> (2007a): ''Identification for Prediction and Decision''. Harvard University Press.</ref> and <ref name="imb:rub15"><span style="font-variant-caps:small-caps">Imbens, G.W., <span style="font-variant-caps:normal">and</span> D.B. Rubin</span> (2015): ''Causal Inference for Statistics, Social, and Biomedical Sciences: An Introduction''. Cambridge University Press.</ref>, to the Handbook chapters by <ref name="hec:vyt07I"><span style="font-variant-caps:small-caps">Heckman, J.J., <span style="font-variant-caps:normal">and</span> E.J. Vytlacil</span> (2007a): “Chapter 70 -- Econometric Evaluation of Social Programs, Part I: Causal Models, Structural Models and Econometric Policy Evaluation” in ''Handbook of Econometrics'', ed. by J.J. Heckman, <span style="font-variant-caps:normal">and</span> E.E. Leamer, vol.6, pp. 4779 -- 4874. Elsevier.</ref><ref name="hec:vyt07II"><span style="font-variant-caps:small-caps">Heckman, J.J., <span style="font-variant-caps:normal">and</span> E.J. Vytlacil</span> (2007b): “Chapter 71 -- Econometric Evaluation of Social Programs, Part II: Using the Marginal Treatment Effect to Organize Alternative Econometric Estimators to Evaluate Social Programs, and to Forecast their Effects in New Environments” in ''Handbook of Econometrics'', ed. by J.J. Heckman, <span style="font-variant-caps:normal">and</span> E.E. Leamer, vol.6, pp. 4875 -- 5143. Elsevier.</ref> and <ref name="abb:hec07"><span style="font-variant-caps:small-caps">Abbring, J.H., <span style="font-variant-caps:normal">and</span> J.J. Heckman</span> (2007): “Chapter 72 -- Econometric Evaluation of Social Programs, Part III: Distributional Treatment Effects, Dynamic Treatment Effects, Dynamic Discrete Choice, and General Equilibrium Policy Evaluation” in ''Handbook of Econometrics'', ed. by J.J. Heckman, <span style="font-variant-caps:normal">and</span> E.E. Leamer, vol.6, pp. 5145 -- 5303. Elsevier.</ref>, and to the review articles by <ref name="imb:woo09"><span style="font-variant-caps:small-caps">Imbens, G.W., <span style="font-variant-caps:normal">and</span> J.M. Wooldridge</span> (2009): “Recent Developments in the Econometrics of Program Evaluation” ''Journal of Economic Literature'', 47(1), 5--86.</ref> and <ref name="mog:tor18"><span style="font-variant-caps:small-caps">Mogstad, M., <span style="font-variant-caps:normal">and</span> A.Torgovitsky</span> (2018): “Identification and Extrapolation of Causal Effects with Instrumental Variables” ''Annual Review of Economics'', 10(1), 577--613.</ref>. | ||
Using standard notation (e.g., <ref name="ney23"></ref>), let <math>\ey:\T \mapsto \cY</math> be an individual-specific response function, with <math>\T=\{0,1,\dots,T\}</math> a finite set of mutually exclusive and exhaustive treatments, and let <math>\es</math> denote the individual's received treatment (taking its realizations in <math>\T</math>).<ref group="Notes" >Here the treatment response is a function only of the (scalar) treatment received by the given individual, an assumption known as ''stable unit treatment value assumption'' | |||
Using standard notation (e.g., <ref name="ney23"><span style="font-variant-caps:small-caps">Neyman, J.S.</span> (1923): “On the Application of Probability Theory to Agricultural Experiments. Essay on Principles. Section 9.” ''Roczniki Nauk Rolniczych'', X, 1--51, reprinted in \textit{Statistical Science}, 5(4), 465-472, translated and edited by D. M. Dabrowska and T. P. Speed from the Polish original.</ref>), let <math>\ey:\T \mapsto \cY</math> be an individual-specific response function, with <math>\T=\{0,1,\dots,T\}</math> a finite set of mutually exclusive and exhaustive treatments, and let <math>\es</math> denote the individual's received treatment (taking its realizations in <math>\T</math>).<ref group="Notes" >Here the treatment response is a function only of the (scalar) treatment received by the given individual, an assumption known as ''stable unit treatment value assumption'' {{ref|name=rub78}}.</ref> | |||
The researcher observes data <math>(\ey,\es,\ex)\sim\sP</math>, with <math>\ey\equiv\ey(\es)</math> the outcome corresponding to the received treatment <math>\es</math>, and <math>\ex</math> a vector of covariates. | The researcher observes data <math>(\ey,\es,\ex)\sim\sP</math>, with <math>\ey\equiv\ey(\es)</math> the outcome corresponding to the received treatment <math>\es</math>, and <math>\ex</math> a vector of covariates. | ||
The outcome <math>\ey(t)</math> for <math>\es\neq t</math> is counterfactual, and hence can be conceptualized as missing. | The outcome <math>\ey(t)</math> for <math>\es\neq t</math> is counterfactual, and hence can be conceptualized as missing. | ||
Therefore, we are in the framework of Identification [[#IP:bounds:mean:md |Problem]] and all the results from Section [[#subsec:missing_data |Selectively Observed Data]] apply in this context too, subject to adjustments in notation.<ref group="Notes" > | Therefore, we are in the framework of Identification [[#IP:bounds:mean:md |Problem]] and all the results from Section [[#subsec:missing_data |Selectively Observed Data]] apply in this context too, subject to adjustments in notation.<ref group="Notes" >{{ref|name=ber:mol:mol12}} and {{ref|name=mol:mol18}}{{rp|at=Section 2.5}} provide a characterization of the sharp identification region for the joint distribution of <math>[\ey(t),t\in\T]</math>.</ref> | ||
For example, using Theorem [[#SIR:prob:E:md |SIR-]], | For example, using Theorem [[#SIR:prob:E:md |SIR-]], | ||
Line 431: | Line 431: | ||
The resulting bounds have width equal to <math>(y_1-y_0)[2-\sP(\es=t_1|\ex=x)-\sP(\es=t_0|\ex=x)]\in[(y_1-y_0),2(y_1-y_0)]</math>, and hence are informative only if both <math>y_0 > -\infty</math> and <math>y_1 < \infty</math>. | The resulting bounds have width equal to <math>(y_1-y_0)[2-\sP(\es=t_1|\ex=x)-\sP(\es=t_0|\ex=x)]\in[(y_1-y_0),2(y_1-y_0)]</math>, and hence are informative only if both <math>y_0 > -\infty</math> and <math>y_1 < \infty</math>. | ||
As the largest logically possible value for the ATE (in the absence of information from data) cannot be larger than <math>(y_1-y_0)</math>, and the smallest cannot be smaller than <math>-(y_1-y_0)</math>, the sharp bounds on the ATE always cover zero. | As the largest logically possible value for the ATE (in the absence of information from data) cannot be larger than <math>(y_1-y_0)</math>, and the smallest cannot be smaller than <math>-(y_1-y_0)</math>, the sharp bounds on the ATE always cover zero. | ||
How should one think about the finding on the size of the worst case bounds on the ATE? | '''Key Insight:''' <i>How should one think about the finding on the size of the worst case bounds on the ATE? | ||
On the one hand, if both <math>y_0 < \infty</math> and <math>y_1 < \infty</math> the bounds are informative, because they are a strict subset of the ATE's possible realizations. | On the one hand, if both <math>y_0 < \infty</math> and <math>y_1 < \infty</math> the bounds are informative, because they are a strict subset of the ATE's possible realizations. | ||
On the other hand, they reveal that the data alone are silent on the sign of the ATE. | On the other hand, they reveal that the data alone are silent on the sign of the ATE. | ||
This means that assumptions play a crucial role in delivering stronger conclusions about this policy relevant parameter. | This means that assumptions play a crucial role in delivering stronger conclusions about this policy relevant parameter. | ||
The partial identification approach to empirical research recommends that as assumptions are added to the analysis, one systematically reports how each contributes to shrinking the bounds, making transparent their role in shaping inference. | The partial identification approach to empirical research recommends that as assumptions are added to the analysis, one systematically reports how each contributes to shrinking the bounds, making transparent their role in shaping inference. </i> | ||
What assumptions may researchers bring to bear to learn more about treatment effects of interest? | What assumptions may researchers bring to bear to learn more about treatment effects of interest? | ||
The literature has provided a wide array of well motivated and useful restrictions. | The literature has provided a wide array of well motivated and useful restrictions. | ||
Here I consider two examples. | Here I consider two examples. | ||
The first one entails ''shape restrictions'' on the treatment response function, leaving selection unrestricted. | The first one entails ''shape restrictions'' on the treatment response function, leaving selection unrestricted. | ||
<ref name="man97:monotone"></ref> obtains bounds on treatment effects under the assumption that the response functions are monotone, semi-monotone, or concave-monotone. | <ref name="man97:monotone"><span style="font-variant-caps:small-caps">Manski, C.F.</span> (1997b): “Monotone Treatment Response” ''Econometrica'', 65(6), 1311--1334.</ref> obtains bounds on treatment effects under the assumption that the response functions are monotone, semi-monotone, or concave-monotone. | ||
These restrictions are motivated by economic theory, where it is commonly presumed, e.g., that demand functions are downward sloping and supply functions are upward sloping. | These restrictions are motivated by economic theory, where it is commonly presumed, e.g., that demand functions are downward sloping and supply functions are upward sloping. | ||
Let the set <math>\T</math> be ordered in terms of degree of intensity. | Let the set <math>\T</math> be ordered in terms of degree of intensity. | ||
Then <ref name="man97:monotone" | Then <ref name="man97:monotone"/>'s ''monotone treatment response'' assumption requires that | ||
<math display="block"> | <math display="block"> | ||
Line 464: | Line 464: | ||
\end{align} | \end{align} | ||
</math> | </math> | ||
Hence, the sharp bounds on <math>\E_\sQ(\ey(t)|\ex=x)</math> are <ref name="man97:monotone" | Hence, the sharp bounds on <math>\E_\sQ(\ey(t)|\ex=x)</math> are <ref name="man97:monotone"/>{{rp|at=Proposition M1}} | ||
<math display="block"> | <math display="block"> | ||
Line 475: | Line 475: | ||
Under the monotone treatment response assumption, the bounds on <math>\E_\sQ(\ey(t)|\ex=x)</math> are obtained using information from all <math>(\ey,\es)</math> pairs (given <math>\ex=x</math>), while the bounds in \eqref{eq:WCB:treat} only use the information provided by <math>(\ey,\es)</math> pairs for which <math>\es=t</math> (given <math>\ex=x</math>). | Under the monotone treatment response assumption, the bounds on <math>\E_\sQ(\ey(t)|\ex=x)</math> are obtained using information from all <math>(\ey,\es)</math> pairs (given <math>\ex=x</math>), while the bounds in \eqref{eq:WCB:treat} only use the information provided by <math>(\ey,\es)</math> pairs for which <math>\es=t</math> (given <math>\ex=x</math>). | ||
As a consequence, the bounds in \eqref{eq:MTR:treat} are informative even if <math>\sP(\es= t|\ex=x)=0</math>, whereas the worst case bounds are not. | As a consequence, the bounds in \eqref{eq:MTR:treat} are informative even if <math>\sP(\es= t|\ex=x)=0</math>, whereas the worst case bounds are not. | ||
Concerning the ATE with <math>t_1 > t_0</math>, under monotone treatment response its lower bound is zero, and its upper bound is obtained by subtracting the lower bound on <math>\E_\sQ(\ey(t_0)|\ex=x)</math> from the upper bound on <math>\E_\sQ(\ey(t_1)|\ex=x)</math>, where both bounds are obtained as in \eqref{eq:MTR:treat} <ref name="man97:monotone" | Concerning the ATE with <math>t_1 > t_0</math>, under monotone treatment response its lower bound is zero, and its upper bound is obtained by subtracting the lower bound on <math>\E_\sQ(\ey(t_0)|\ex=x)</math> from the upper bound on <math>\E_\sQ(\ey(t_1)|\ex=x)</math>, where both bounds are obtained as in \eqref{eq:MTR:treat} <ref name="man97:monotone"/>{{rp|at=Proposition M2}}. | ||
The second example of assumptions used to tighten worst case bounds is that of ''exclusion restrictions'', as in, e.g., <ref name="man90"></ref>. | The second example of assumptions used to tighten worst case bounds is that of ''exclusion restrictions'', as in, e.g., <ref name="man90"><span style="font-variant-caps:small-caps">Manski, C.F.</span> (1990): “Nonparametric Bounds on Treatment Effects” ''The American Economic Review Papers and Proceedings'', 80(2), 319--323.</ref>. | ||
Suppose the researcher observes a random variable <math>\ez</math>, taking its realizations in <math>\cZ</math>, such that<ref group="Notes" >Stronger exclusion restrictions include statistical independence of the response function at each <math>t</math> with <math>\ez</math>: <math>\sQ(\ey(t)|\ez,\ex)=\sQ(\ey(t)|\ex)~\forall t \in\T,~\ex</math>-a.s.; and statistical independence of the entire response function with <math>\ez</math>: <math>\sQ([\ey(t),t \in\T]|\ez,\ex)=\sQ([\ey(t),t \in\T]|\ex),~\ex</math>-a.s. | Suppose the researcher observes a random variable <math>\ez</math>, taking its realizations in <math>\cZ</math>, such that<ref group="Notes" >Stronger exclusion restrictions include statistical independence of the response function at each <math>t</math> with <math>\ez</math>: <math>\sQ(\ey(t)|\ez,\ex)=\sQ(\ey(t)|\ex)~\forall t \in\T,~\ex</math>-a.s.; and statistical independence of the entire response function with <math>\ez</math>: <math>\sQ([\ey(t),t \in\T]|\ez,\ex)=\sQ([\ey(t),t \in\T]|\ex),~\ex</math>-a.s. | ||
Examples of partial identification analysis under these conditions can be found in | Examples of partial identification analysis under these conditions can be found in {{ref|name=bal:pea97}}, {{ref|name=man03}}, {{ref|name=kit09}}, {{ref|name=ber:mol:mol12}}, {{ref|name=mac:sha:vyt18}}, and many others.</ref> | ||
<math display="block"> | <math display="block"> | ||
Line 500: | Line 500: | ||
If the instrument affects the probability of being selected into treatment, or the average outcome for the subpopulation receiving treatment <math>t</math>, the bounds on <math>\E_\sQ(\ey(t)|\ex=x)</math> shrink. | If the instrument affects the probability of being selected into treatment, or the average outcome for the subpopulation receiving treatment <math>t</math>, the bounds on <math>\E_\sQ(\ey(t)|\ex=x)</math> shrink. | ||
If the bounds are empty, the mean independence assumption can be refuted (see [[guide:7b0105e1fc#sec:misspec |Section]] for a discussion of misspecification in partial identification). | If the bounds are empty, the mean independence assumption can be refuted (see [[guide:7b0105e1fc#sec:misspec |Section]] for a discussion of misspecification in partial identification). | ||
<ref name="man:pep00"></ref><ref name="man:pep09"></ref> generalize the notion of instrumental variable to ''monotone'' instrumental variable, and show how these can be used to obtain tighter bounds on treatment effect parameters.<ref group="Notes" >See | <ref name="man:pep00"><span style="font-variant-caps:small-caps">Manski, C.F., <span style="font-variant-caps:normal">and</span> J.V. Pepper</span> (2000): “Monotone Instrumental Variables: With an Application to the Returns to Schooling” ''Econometrica'', 68(4), 997--1010.</ref><ref name="man:pep09"><span style="font-variant-caps:small-caps">Manski, C.F., <span style="font-variant-caps:normal">and</span> J.V. Pepper</span> (2009): “More on monotone instrumental variables” ''The Econometrics Journal'', 12(s1), S200--S216.</ref> generalize the notion of instrumental variable to ''monotone'' instrumental variable, and show how these can be used to obtain tighter bounds on treatment effect parameters.<ref group="Notes" >See {{ref|name=che:ros19}}{{rp|at=Chapter XXX in this Volume}} for further discussion.</ref> | ||
They also show how shape restrictions and exclusion restrictions can jointly further tighten the bounds. | They also show how shape restrictions and exclusion restrictions can jointly further tighten the bounds. | ||
<ref name="man13social"></ref> generalizes these findings to the case where treatment response may have social interactions -- that is, each individual's outcome depends on the treatment received by all other individuals. | <ref name="man13social"><span style="font-variant-caps:small-caps">Manski, C.F.</span> (2013a): “Identification of treatment response with social interactions” ''The Econometrics Journal'', 16(1), S1--S23.</ref> generalizes these findings to the case where treatment response may have social interactions -- that is, each individual's outcome depends on the treatment received by all other individuals. | ||
==<span id="subsec:interval_data"></span>Interval Data== | |||
Identification [[#IP:bounds:mean:md |Problem]], as well as the treatment evaluation problem in Section [[#subsec:programme:eval |Treatment Effects with and without Instrumental Variables]], is an instance of the more general question of what can be learned about (functionals of) probability distributions of interest, in the presence of interval valued outcome and/or covariate data. | Identification [[#IP:bounds:mean:md |Problem]], as well as the treatment evaluation problem in Section [[#subsec:programme:eval |Treatment Effects with and without Instrumental Variables]], is an instance of the more general question of what can be learned about (functionals of) probability distributions of interest, in the presence of interval valued outcome and/or covariate data. | ||
Such data have become commonplace in Economics. | Such data have become commonplace in Economics. | ||
For example, since the early 1990s the Health and Retirement Study collects income data from survey respondents in the form of brackets, with degenerate (singleton) intervals for individuals who opt to fully reveal their income (see, e.g., <ref name="jus:suz95"></ref>). | For example, since the early 1990s the Health and Retirement Study collects income data from survey respondents in the form of brackets, with degenerate (singleton) intervals for individuals who opt to fully reveal their income (see, e.g., <ref name="jus:suz95"><span style="font-variant-caps:small-caps">Juster, F.T., <span style="font-variant-caps:normal">and</span> R.Suzman</span> (1995): “An Overview of the Health and Retirement Study” ''Journal of Human Resources'', 30 (Supplement), S7--S56.</ref>). | ||
Due to concerns for privacy, public use tax data are recorded as the number of tax payers which belong to each of a finite number of cells (see, e.g., <ref name="pic05"></ref>). | Due to concerns for privacy, public use tax data are recorded as the number of tax payers which belong to each of a finite number of cells (see, e.g., <ref name="pic05"><span style="font-variant-caps:small-caps">Picketty, T.</span> (2005): “Top Income Shares in the Long Run: An Overview” ''Journal of the European Economic Association'', 3, 382--392.</ref>). | ||
The Occupational Employment Statistics (OES) program at the Bureau of Labor Statistics <ref name="BLS"></ref> collects wage data from employers as intervals, and uses these data to construct estimates | The Occupational Employment Statistics (OES) program at the Bureau of Labor Statistics <ref name="BLS"><span style="font-variant-caps:small-caps">{Bureau of Labor Statistics}</span> (2018): “Occupational Employment Statistics” U.S. Department of Labor, available online at [www.bls.gov/oes/ www.bls.gov/oes/]; accessed 1/28/2018.</ref> collects wage data from employers as intervals, and uses these data to construct estimates | ||
for wage and salary workers in more than 800 detailed occupations. | for wage and salary workers in more than 800 detailed occupations. | ||
<ref name="man:mol10"></ref> and <ref name="giu:man:mol19round"></ref> document the extensive prevalence of rounding in survey responses to probabilistic expectation questions, and propose to use a person's response pattern across different questions to infer his rounding practice, the result being interpretation of reported numerical values as interval data. | <ref name="man:mol10"><span style="font-variant-caps:small-caps">Manski, C.F., <span style="font-variant-caps:normal">and</span> F.Molinari</span> (2010): “Rounding Probabilistic Expectations in Surveys” ''Journal of Business and Economic Statistics'', 28(2), 219--231.</ref> and <ref name="giu:man:mol19round"><span style="font-variant-caps:small-caps">Giustinelli, P., C.F. Manski, <span style="font-variant-caps:normal">and</span> F.Molinari</span> (2019b): “Tail and Center Rounding of Probabilistic Expectations in the Health and Retirement Study” available at [http://faculty.wcas.northwestern.edu/cfm754/gmm_rounding.pdf http://faculty.wcas.northwestern.edu/cfm754/gmm_rounding.pdf].</ref> document the extensive prevalence of rounding in survey responses to probabilistic expectation questions, and propose to use a person's response pattern across different questions to infer his rounding practice, the result being interpretation of reported numerical values as interval data. | ||
Other instances abound. | Other instances abound. | ||
Here I focus first on the case of interval outcome data. | Here I focus first on the case of interval outcome data. | ||
{{proofcard|Identification Problem (Interval Outcome Data)|IP:interval_outcome|Assume that in addition to being compact, either <math>\cY</math> is countable or <math>\cY=[y_0,y_1]</math>, with <math>y_0=\min_{y\in\cY}y</math> and <math>y_1=\max_{y\in\cY}y</math>. | |||
Let <math>(\yL,\yU,\ex)\sim\sP</math> be observable random variables and <math>\ey</math> be an unobservable random variable whose distribution (or features thereof) is of interest, with <math>\yL,\yU,\ey\in\cY</math>. | Let <math>(\yL,\yU,\ex)\sim\sP</math> be observable random variables and <math>\ey</math> be an unobservable random variable whose distribution (or features thereof) is of interest, with <math>\yL,\yU,\ey\in\cY</math>. | ||
Suppose that <math>(\yL,\yU,\ey)</math> are such that <math>\sR(\yL\le\ey\le\yU)=1</math>.<ref group="Notes" > | Suppose that <math>(\yL,\yU,\ey)</math> are such that <math>\sR(\yL\le\ey\le\yU)=1</math>.<ref group="Notes" ><span id="fn:missing_special_case_interval"/>In Identification [[#IP:bounds:mean:md |Problem]] the observable variables are <math>(\ey\ed,\ed,\ex)</math>, and <math>(\yL,\yU)</math> are determined as follows: <math>\yL=\ey\ed+y_0(1-\ed)</math>, <math>\yU=\ey\ed+y_1(1-\ed)</math>. For the analysis in Section [[#subsec:programme:eval |Treatment Effects with and without Instrumental Variables]], the data is <math>(\ey,\es,\ex)</math> and <math>\yL=\ey\one(\es=t)+y_0\one(\es\ne t)</math>, <math>\yU=\ey\one(\es=t)+y_1\one(\es\ne t)</math>. | ||
Hence, <math>\sP(\yL\le\ey\le\yU)=1</math> by construction.</ref> | Hence, <math>\sP(\yL\le\ey\le\yU)=1</math> by construction.</ref> | ||
In the absence of additional information, what can the researcher learn about features of <math>\sQ(\ey|\ex=x)</math>, the conditional distribution of <math>\ey</math> given <math>\ex=x</math>? | In the absence of additional information, what can the researcher learn about features of <math>\sQ(\ey|\ex=x)</math>, the conditional distribution of <math>\ey</math> given <math>\ex=x</math>? | ||
|}} | |||
It is immediate to obtain the sharp identification region | It is immediate to obtain the sharp identification region | ||
Line 549: | Line 550: | ||
\end{align*} | \end{align*} | ||
</math> | </math> | ||
Then <math>\eY</math> is a random closed set according to [[guide:379e0dcd67#def:rcs |Definition]].<ref group="Notes" >For a proof of this statement, see | Then <math>\eY</math> is a random closed set according to [[guide:379e0dcd67#def:rcs |Definition]].<ref group="Notes" >For a proof of this statement, see {{ref|name=mol:mol18}}{{rp|at=Example 1.11}}.</ref> The requirement <math>\sR(\yL\le\ey\le\yU)=1</math> can be equivalently expressed as | ||
<math display="block"> | <math display="block"> | ||
Line 558: | Line 559: | ||
</math> | </math> | ||
Equation \eqref{eq:y_in_Y}, together with knowledge of <math>\sP</math>, exhausts all the information in the data and maintained assumptions. | Equation \eqref{eq:y_in_Y}, together with knowledge of <math>\sP</math>, exhausts all the information in the data and maintained assumptions. | ||
In order to harness such information to characterize the set of observationally equivalent probability distributions for <math>\ey</math>, one can leverage a result due to <ref name="art83"></ref> (and <ref name="nor92"></ref>), reported in [[guide:379e0dcd67#thr:artstein |Theorem]] in [[guide:379e0dcd67#app:RCS |Appendix]], which allows one to translate \eqref{eq:y_in_Y} into a collection of conditional moment inequalities. | In order to harness such information to characterize the set of observationally equivalent probability distributions for <math>\ey</math>, one can leverage a result due to <ref name="art83"><span style="font-variant-caps:small-caps">Artstein, Z.</span> (1983): “Distributions of random sets and random selections” ''Israel Journal of Mathematics'', 46, 313--324.</ref> (and <ref name="nor92"><span style="font-variant-caps:small-caps">Norberg, T.</span> (1992): “On the existence of ordered couplings of random sets --- with applications” ''Israel Journal of Mathematics'', 77, 241--264.</ref>), reported in [[guide:379e0dcd67#thr:artstein |Theorem]] in [[guide:379e0dcd67#app:RCS |Appendix]], which allows one to translate \eqref{eq:y_in_Y} into a collection of conditional moment inequalities. | ||
Specifically, let <math>\cT</math> denote the space of all probability measures with support in <math>\cY</math>. | Specifically, let <math>\cT</math> denote the space of all probability measures with support in <math>\cY</math>. | ||
{{proofcard|Theorem (Conditional Distribution of Interval-Observed Outcome Data)|SIR:CDF_id| | |||
Given <math>\tau\in\cT</math>, let <math>\tau_K(x)</math> denote the probability that distribution <math>\tau</math> assigns to set <math>K</math> conditional on <math>\ex=x</math>. | Given <math>\tau\in\cT</math>, let <math>\tau_K(x)</math> denote the probability that distribution <math>\tau</math> assigns to set <math>K</math> conditional on <math>\ex=x</math>. | ||
Under the assumptions in Identification [[#IP:interval_outcome |Problem]], the sharp identification region for <math>\sQ(\ey|\ex=x)</math> is | Under the assumptions in Identification [[#IP:interval_outcome |Problem]], the sharp identification region for <math>\sQ(\ey|\ex=x)</math> is | ||
Line 577: | Line 577: | ||
\end{align} | \end{align} | ||
</math> | </math> | ||
| | |||
[[guide:379e0dcd67#thr:artstein |Theorem]] yields \eqref{eq:sharp_id_P_interval_1}. | [[guide:379e0dcd67#thr:artstein |Theorem]] yields \eqref{eq:sharp_id_P_interval_1}. | ||
If <math>\cY=[y_0,y_1]</math>, <ref name="mol:mol18" | If <math>\cY=[y_0,y_1]</math>, <ref name="mol:mol18"/>{{rp|at=Theorem 2.25}} show that it suffices to verify the inequalities in \eqref{eq:sharp_id_P_interval_2} for sets <math>K</math> that are intervals. | ||
}} | |||
Compare equation \eqref{eq:sharp_id_P_interval_1} with equation \eqref{eq:sharp_id_P_md_Manski}. | Compare equation \eqref{eq:sharp_id_P_interval_1} with equation \eqref{eq:sharp_id_P_md_Manski}. | ||
Under the set-up of Identification [[#IP:bounds:mean:md |Problem]], when <math>\ed=1</math> we have <math>\eY=\{\ey\}</math> and when <math>\ed=0</math> we have <math>\eY=\cY</math>. | Under the set-up of Identification [[#IP:bounds:mean:md |Problem]], when <math>\ed=1</math> we have <math>\eY=\{\ey\}</math> and when <math>\ed=0</math> we have <math>\eY=\cY</math>. | ||
Hence, for any <math>K \subsetneq \cY</math>, <math>\sP(\eY \subset K|\ex=x)=\sP(\ey\in K|\ex=x,\ed=1)\sP(\ed=1)</math>.<ref group="Notes" >For <math>K = \cY</math>, both \eqref{eq:sharp_id_P_interval_1} and \eqref{eq:sharp_id_P_md_Manski} hold trivially.</ref> | Hence, for any <math>K \subsetneq \cY</math>, <math>\sP(\eY \subset K|\ex=x)=\sP(\ey\in K|\ex=x,\ed=1)\sP(\ed=1)</math>.<ref group="Notes" >For <math>K = \cY</math>, both \eqref{eq:sharp_id_P_interval_1} and \eqref{eq:sharp_id_P_md_Manski} hold trivially.</ref> | ||
It follows that the characterizations in \eqref{eq:sharp_id_P_interval_1} and \eqref{eq:sharp_id_P_md_Manski} are equivalent. | It follows that the characterizations in \eqref{eq:sharp_id_P_interval_1} and \eqref{eq:sharp_id_P_md_Manski} are equivalent. | ||
If <math>\cY</math> is countable, it is easy to show that \eqref{eq:sharp_id_P_interval_1} simplifies to \eqref{eq:sharp_id_P_md_Manski} (see, e.g., <ref name="ber:mol:mol12"></ref>{{rp|at=Proposition 2.2}}). | If <math>\cY</math> is countable, it is easy to show that \eqref{eq:sharp_id_P_interval_1} simplifies to \eqref{eq:sharp_id_P_md_Manski} (see, e.g., <ref name="ber:mol:mol12"><span style="font-variant-caps:small-caps">Beresteanu, A., I.Molchanov, <span style="font-variant-caps:normal">and</span> F.Molinari</span> (2012): “Partial identification using random set theory” ''Journal of Econometrics'', 166(1), 17 -- 32, with errata at [https://molinari.economics.cornell.edu/docs/NOTE_BMM2012_v3.pdf https://molinari.economics.cornell.edu/docs/NOTE_BMM2012_v3.pdf].</ref>{{rp|at=Proposition 2.2}}). | ||
'''Key Insight (Random set theory and partial identification):'''<span id="big_idea:pi_and_rs"/><i>The mathematical framework for the analysis of random closed sets embodied in random set theory is naturally suited to conduct identification analysis and statistical inference in partially identified models. | |||
The mathematical framework for the analysis of random closed sets embodied in random set theory is naturally suited to conduct identification analysis and statistical inference in partially identified models. | This is because, as argued by <ref name="ber:mol08"><span style="font-variant-caps:small-caps">Beresteanu, A., <span style="font-variant-caps:normal">and</span> F.Molinari</span> (2008): “Asymptotic Properties for a Class of Partially Identified Models” ''Econometrica'', 76(4), 763--814.</ref> and <ref name="ber:mol:mol11"><span style="font-variant-caps:small-caps">Beresteanu, A., I.Molchanov, <span style="font-variant-caps:normal">and</span> F.Molinari</span> (2011): “Sharp identification regions in models with convex moment predictions” ''Econometrica'', 79(6), 1785--1821.</ref><ref name="ber:mol:mol12"/>, lack of point identification can often be traced back to a collection of random variables that are consistent with the available data and maintained assumptions. | ||
This is because, as argued by <ref name="ber:mol08"></ref> and <ref name="ber:mol:mol11"></ref><ref name="ber:mol:mol12" | |||
In turn, this collection of random variables is equal to the family of selections of a properly specified random closed set, so that random set theory applies. | In turn, this collection of random variables is equal to the family of selections of a properly specified random closed set, so that random set theory applies. | ||
The interval data case is a simple example that illustrates this point. | The interval data case is a simple example that illustrates this point. | ||
More examples are given throughout this chapter. | More examples are given throughout this chapter. | ||
As mentioned in the Introduction, the exercise of defining the random closed set that is relevant for the problem under consideration is routinely carried out in partial identification analysis, even when random set theory is not applied. | As mentioned in the Introduction, the exercise of defining the random closed set that is relevant for the problem under consideration is routinely carried out in partial identification analysis, even when random set theory is not applied. | ||
For example, in the case of treatment effect analysis with monotone response function, <ref name="man97:monotone" | For example, in the case of treatment effect analysis with monotone response function, <ref name="man97:monotone"/> derived the set in the right-hand-side of \eqref{eq:RCS:MTR}, which satisfies Definition [[guide:379e0dcd67#def:rcs |def:rcs]].</i> | ||
An attractive feature of the characterization in \eqref{eq:sharp_id_P_interval_1} is that it holds regardless of the specific assumptions on <math>\yL,\,\yU</math>, and <math>\cY</math>. | An attractive feature of the characterization in \eqref{eq:sharp_id_P_interval_1} is that it holds regardless of the specific assumptions on <math>\yL,\,\yU</math>, and <math>\cY</math>. | ||
Later sections in this chapter illustrate how [[guide:379e0dcd67#thr:artstein |Theorem]] delivers the sharp identification region in other more complex instances of partial identification of probability distributions, as well as in structural models. | Later sections in this chapter illustrate how [[guide:379e0dcd67#thr:artstein |Theorem]] delivers the sharp identification region in other more complex instances of partial identification of probability distributions, as well as in structural models. | ||
In Chapter '''XXX''' in this Volume, <ref name="che:ros19"></ref> apply [[guide:379e0dcd67#thr:artstein |Theorem]] to obtain sharp identification regions for functionals of interest in the important class of ''generalized instrumental variable models''. To avoid repetitions, I do not systematically discuss that class of models in this chapter. | In Chapter '''XXX''' in this Volume, <ref name="che:ros19"><span style="font-variant-caps:small-caps">Chesher, A., <span style="font-variant-caps:normal">and</span> A.M. Rosen</span> (2019): “Generalized instrumental variable models, methods, and applications” in ''Handbook of Econometrics''. Elsevier.</ref> apply [[guide:379e0dcd67#thr:artstein |Theorem]] to obtain sharp identification regions for functionals of interest in the important class of ''generalized instrumental variable models''. To avoid repetitions, I do not systematically discuss that class of models in this chapter. | ||
When addressing questions about features of <math>\sQ(\ey|\ex=x)</math> in the presence of interval outcome data, an alternative approach (e.g. <ref name="tam10"></ref><ref name="pon:tam11"></ref>) looks at all (random) mixtures of <math>\yL,\yU</math>. | When addressing questions about features of <math>\sQ(\ey|\ex=x)</math> in the presence of interval outcome data, an alternative approach (e.g. <ref name="tam10"><span style="font-variant-caps:small-caps">Tamer, E.</span> (2010): “Partial Identification in Econometrics” ''Annual Review of Economics'', 2, 167--195.</ref><ref name="pon:tam11"><span style="font-variant-caps:small-caps">Ponomareva, M., <span style="font-variant-caps:normal">and</span> E.Tamer</span> (2011): “Misspecification in moment inequality models: back to moment equalities?” ''The Econometrics Journal'', 14(2), 186--203.</ref>) looks at all (random) mixtures of <math>\yL,\yU</math>. | ||
The approach is based on a random variable <math>\eu</math> (a ''selection mechanism'' that picks an element of <math>\eY</math>) with values in <math>[0,1]</math>, whose distribution conditional on <math>\yL,\yU</math> is left completely unspecified. | The approach is based on a random variable <math>\eu</math> (a ''selection mechanism'' that picks an element of <math>\eY</math>) with values in <math>[0,1]</math>, whose distribution conditional on <math>\yL,\yU</math> is left completely unspecified. | ||
Using this random variable, one defines | Using this random variable, one defines | ||
Line 612: | Line 611: | ||
This is because each <math>\ey_\eu</math> is a (stochastic) convex combination of <math>\yL,\yU</math>, hence each of these random variables satisfies <math>\sR(\yL\le\ey_\eu\le\yU)=1</math>. | This is because each <math>\ey_\eu</math> is a (stochastic) convex combination of <math>\yL,\yU</math>, hence each of these random variables satisfies <math>\sR(\yL\le\ey_\eu\le\yU)=1</math>. | ||
While such characterization is sharp, it can be of difficult implementation in practice, because it requires working with all possible random variables <math>\ey_\eu</math> built using all possible random variables <math>\eu</math> with support in <math>[0,1]</math>. | While such characterization is sharp, it can be of difficult implementation in practice, because it requires working with all possible random variables <math>\ey_\eu</math> built using all possible random variables <math>\eu</math> with support in <math>[0,1]</math>. | ||
[[guide:379e0dcd67#thr:artstein |Theorem]] allows one to bypass the use of <math>\eu</math>, and obtain directly a characterization of the sharp identification region for <math>\sQ(\ey|\ex=x)</math> based on conditional moment inequalities.<ref group="Notes" >It can be shown that the collection of random variables <math>\ey_\eu</math> equals the collection of ''measurable selections'' of the random closed set <math>\eY\equiv [\yL,\yU]</math> (see [[guide:379e0dcd67#def:selection |Definition]]); see | [[guide:379e0dcd67#thr:artstein |Theorem]] allows one to bypass the use of <math>\eu</math>, and obtain directly a characterization of the sharp identification region for <math>\sQ(\ey|\ex=x)</math> based on conditional moment inequalities.<ref group="Notes" >It can be shown that the collection of random variables <math>\ey_\eu</math> equals the collection of ''measurable selections'' of the random closed set <math>\eY\equiv [\yL,\yU]</math> (see [[guide:379e0dcd67#def:selection |Definition]]); see {{ref|name=ber:mol:mol11}}{{rp|at=Lemma 2.1}}. | ||
[[guide:379e0dcd67#thr:artstein |Theorem]] provides a characterization of the distribution of any <math>\ey_\eu</math> that satisfies <math>\ey_\eu \in \eY</math> a.s., based on a dominance condition that relates the distribution of <math>\ey_\eu</math> to the distribution of the random set <math>\eY</math>. | [[guide:379e0dcd67#thr:artstein |Theorem]] provides a characterization of the distribution of any <math>\ey_\eu</math> that satisfies <math>\ey_\eu \in \eY</math> a.s., based on a dominance condition that relates the distribution of <math>\ey_\eu</math> to the distribution of the random set <math>\eY</math>. | ||
Such dominance condition is given by the inequalities in \eqref{eq:sharp_id_P_interval_1}. | Such dominance condition is given by the inequalities in \eqref{eq:sharp_id_P_interval_1}. | ||
</ref> | </ref> | ||
<ref name="hor:man98"></ref><ref name="hor:man00"></ref> study nonparametric conditional prediction problems with missing outcome and/or missing covariate data. | <ref name="hor:man98"><span style="font-variant-caps:small-caps">Horowitz, J.L., <span style="font-variant-caps:normal">and</span> C.F. Manski</span> (1998): “Censoring of outcomes and regressors due to survey nonresponse: Identification and estimation using weights and imputations” ''Journal of Econometrics'', 84(1), 37 -- 58.</ref><ref name="hor:man00"><span style="font-variant-caps:small-caps">Horowitz, J.L., <span style="font-variant-caps:normal">and</span> C.F. Manski</span> (2000): “Nonparametric Analysis of Randomized Experiments with Missing Covariate and Outcome Data” ''Journal of the American Statistical Association'', 95(449), 77--84.</ref> study nonparametric conditional prediction problems with missing outcome and/or missing covariate data. | ||
Their analysis shows that this problem is considerably more pernicious than the case where only outcome data are missing. | Their analysis shows that this problem is considerably more pernicious than the case where only outcome data are missing. | ||
For the case of interval covariate data, <ref name="man:tam02"></ref> provide a set of sufficient conditions under which simple and elegant sharp bounds on functionals of <math>\sQ(\ey|\ex)</math> can be obtained, even in this substantially harder identification problem. | For the case of interval covariate data, <ref name="man:tam02"><span style="font-variant-caps:small-caps">Manski, C.F., <span style="font-variant-caps:normal">and</span> E.Tamer</span> (2002): “Inference on Regressions with Interval Data on a Regressor or Outcome” ''Econometrica'', 70(2), 519--546.</ref> provide a set of sufficient conditions under which simple and elegant sharp bounds on functionals of <math>\sQ(\ey|\ex)</math> can be obtained, even in this substantially harder identification problem. | ||
Their assumptions are listed in Identification [[#IP:interval_covariate |Problem]], and their result (with proof) in Theorem [[#SIR:man:tam:nonpar |SIR-]]. | Their assumptions are listed in Identification [[#IP:interval_covariate |Problem]], and their result (with proof) in Theorem [[#SIR:man:tam:nonpar |SIR-]]. | ||
{{proofcard|Identification Problem (Interval Covariate Data)|IP:interval_covariate| | |||
Let <math>(\ey,\xL,\xU)\sim\sP</math> be observable random variables in <math>\R\times\R\times\R</math> and <math>\ex\in\R</math> be an unobservable random variable. | Let <math>(\ey,\xL,\xU)\sim\sP</math> be observable random variables in <math>\R\times\R\times\R</math> and <math>\ex\in\R</math> be an unobservable random variable. | ||
Suppose that <math>\sR</math>, the joint distribution of <math>(\ey,\ex,\xL,\xU)</math>, is such that: (I) <math>\sR(\xL\le\ex\le\xU)=1</math>; (M) <math>\E_\sQ(\ey|\ex=x)</math> is weakly increasing in <math>x</math>; and (MI) <math>\E_{\sR}(\ey|\ex,\xL,\xU)=\E_\sQ(\ey|\ex)</math>. | Suppose that <math>\sR</math>, the joint distribution of <math>(\ey,\ex,\xL,\xU)</math>, is such that: (I) <math>\sR(\xL\le\ex\le\xU)=1</math>; (M) <math>\E_\sQ(\ey|\ex=x)</math> is weakly increasing in <math>x</math>; and (MI) <math>\E_{\sR}(\ey|\ex,\xL,\xU)=\E_\sQ(\ey|\ex)</math>. | ||
In the absence of additional information, what can the researcher learn about <math>\E_\sQ(\ey|\ex=x)</math> for given <math>x\in\cX</math>? | In the absence of additional information, what can the researcher learn about <math>\E_\sQ(\ey|\ex=x)</math> for given <math>x\in\cX</math>? | ||
|}} | |||
Compared to the earlier discussion for the interval outcome case, here there are two additional assumptions. | Compared to the earlier discussion for the interval outcome case, here there are two additional assumptions. | ||
The monotonicity condition (M) is a simple shape restrictions, which however requires some prior knowledge about the joint distribution of <math>(\ey,\ex)</math>. | The monotonicity condition (M) is a simple shape restrictions, which however requires some prior knowledge about the joint distribution of <math>(\ey,\ex)</math>. | ||
The mean independence restriction (MI) requires that if <math>\ex</math> were observed, knowledge of <math>(\xL,\xU)</math> would not affect the conditional expectation of <math>\ey|\ex</math>. | The mean independence restriction (MI) requires that if <math>\ex</math> were observed, knowledge of <math>(\xL,\xU)</math> would not affect the conditional expectation of <math>\ey|\ex</math>. | ||
The assumption is not innocuous, as pointed out by the authors. | The assumption is not innocuous, as pointed out by the authors. | ||
For example, it may fail if censoring is endogenous.<ref group="Notes" > | For example, it may fail if censoring is endogenous.<ref group="Notes" ><span id="foot:auc:bug:hot17"/>For the case of missing covariate data, which is a special case of interval covariate data similarly to arguments in [[#fn:missing_special_case_interval |footnote]], {{ref|name=auc:bug:hot17}} show that the MI restriction implies the assumption that data is missing at random.</ref> | ||
{{proofcard|Theorem (Conditional Expectation with Interval-Observed Covariate Data)|SIR:man:tam:nonpar| | |||
Under the assumptions of Identification [[#IP:interval_covariate |Problem]], the sharp identification region for <math>\E_\sQ(\ey|\ex=x)</math> for given <math>x\in\cX</math> is | Under the assumptions of Identification [[#IP:interval_covariate |Problem]], the sharp identification region for <math>\E_\sQ(\ey|\ex=x)</math> for given <math>x\in\cX</math> is | ||
Line 642: | Line 639: | ||
\end{align} | \end{align} | ||
</math> | </math> | ||
| | |||
The law of iterated expectations and the independence assumption yield <math>\E_\sP(\ey|\xL,\xU)=\int \E_\sQ(\ey|\ex)d\sR(\ex|\xL,\xU)</math>. | The law of iterated expectations and the independence assumption yield <math>\E_\sP(\ey|\xL,\xU)=\int \E_\sQ(\ey|\ex)d\sR(\ex|\xL,\xU)</math>. | ||
For all <math>\underline{x}\le \bar{x}</math>, the monotonicity assumption and the fact that <math>\ex\in[\xL,\xU]</math>-a.s. yield <math>\E_\sQ(\ey|\ex=\underline{x})\le \int \E_\sQ(\ey|\ex)d\sR(\ex|\xL=\underline{x},\xU=\bar{x}) \le \E_\sQ(\ey|\ex=\bar{x})</math>. | For all <math>\underline{x}\le \bar{x}</math>, the monotonicity assumption and the fact that <math>\ex\in[\xL,\xU]</math>-a.s. yield <math>\E_\sQ(\ey|\ex=\underline{x})\le \int \E_\sQ(\ey|\ex)d\sR(\ex|\xL=\underline{x},\xU=\bar{x}) \le \E_\sQ(\ey|\ex=\bar{x})</math>. | ||
Line 650: | Line 647: | ||
The bound is weakly increasing as a function of <math>x</math>, so that the monotonicity assumption on <math>\E_\sQ(\ey|\ex=x)</math> holds and the bound is sharp. | The bound is weakly increasing as a function of <math>x</math>, so that the monotonicity assumption on <math>\E_\sQ(\ey|\ex=x)</math> holds and the bound is sharp. | ||
The argument for the upper bound can be concluded similarly. | The argument for the upper bound can be concluded similarly. | ||
}} | |||
Learning about functionals of <math>\sQ(\ey|\ex=x)</math> naturally implies learning about predictors of <math>\ey|\ex=x</math>. | Learning about functionals of <math>\sQ(\ey|\ex=x)</math> naturally implies learning about predictors of <math>\ey|\ex=x</math>. | ||
For example, <math>\idr{\E_\sQ(\ey|\ex=x)}</math> yields the collection of values for the best predictor under square loss; | For example, <math>\idr{\E_\sQ(\ey|\ex=x)}</math> yields the collection of values for the best predictor under square loss; | ||
Line 660: | Line 657: | ||
I return to such assumptions at the end of this section. | I return to such assumptions at the end of this section. | ||
For now, in the example of linearity and square loss, I am referring to best linear prediction, i.e., best linear approximation to <math>\E_\sQ(\ey|\ex)</math>. | For now, in the example of linearity and square loss, I am referring to best linear prediction, i.e., best linear approximation to <math>\E_\sQ(\ey|\ex)</math>. | ||
<ref name="man03" | <ref name="man03"/>{{rp|at=pp. 56-58}} discusses what can be learned about the best linear predictor of <math>\ey</math> conditional on <math>\ex</math>, when only interval data on <math>(\ey,\ex)</math> is available. | ||
I treat first the case of interval outcome and perfectly observed covariates. | I treat first the case of interval outcome and perfectly observed covariates. | ||
{{proofcard|Identification Problem (Parametric Prediction with Interval Outcome Data)|IP:param_pred_interval|Maintain the same assumptions as in Identification [[#IP:interval_outcome |Problem]]. | |||
Maintain the same assumptions as in Identification [[#IP:interval_outcome |Problem]]. | |||
Let <math>(\yL,\yU,\ex)\sim\sP</math> be observable random variables and <math>\ey</math> be an unobservable random variable, with <math>\sR(\yL\le\ey\le\yU)=1</math>. | Let <math>(\yL,\yU,\ex)\sim\sP</math> be observable random variables and <math>\ey</math> be an unobservable random variable, with <math>\sR(\yL\le\ey\le\yU)=1</math>. | ||
In the absence of additional information, what can the researcher learn about the best linear predictor of <math>\ey</math> given <math>\ex=x</math>? | In the absence of additional information, what can the researcher learn about the best linear predictor of <math>\ey</math> given <math>\ex=x</math>? | ||
|}} | |||
For simplicity suppose that <math>\ex</math> is a scalar, and let <math>\theta=[\theta_0~\theta_1]^\top\in\Theta\subset\R^2</math> denote the parameter vector of the best linear predictor of <math>\ey|\ex</math>. | For simplicity suppose that <math>\ex</math> is a scalar, and let <math>\theta=[\theta_0~\theta_1]^\top\in\Theta\subset\R^2</math> denote the parameter vector of the best linear predictor of <math>\ey|\ex</math>. | ||
Assume that <math>Var(\ex) > 0</math>. | Assume that <math>Var(\ex) > 0</math>. | ||
Line 690: | Line 685: | ||
\end{multline} | \end{multline} | ||
</math> | </math> | ||
<ref name="ber:mol08" | <ref name="ber:mol08"/>{{rp|at=Proposition 4.1}} show that \eqref{eq:manski_blp} can be re-written in an intuitive way that generalizes the well-known formula for the best linear predictor that arises when <math>\ey</math> is perfectly observed. | ||
Define the random segment <math>\eG</math> and the matrix <math>\Sigma_\sP</math> as | Define the random segment <math>\eG</math> and the matrix <math>\Sigma_\sP</math> as | ||
Line 707: | Line 702: | ||
</math> | </math> | ||
where <math>\Sel(\eY)</math> is the set of all measurable selections from <math>\eY</math>, see [[guide:379e0dcd67#def:selection |Definition]]. Then, | where <math>\Sel(\eY)</math> is the set of all measurable selections from <math>\eY</math>, see [[guide:379e0dcd67#def:selection |Definition]]. Then, | ||
{{proofcard|Theorem (Best Linear Predictor with Interval Outcome Data)|SIR:BLP_intervalY| | |||
Under the assumptions of Identification [[#IP:param_pred_interval |Problem]], the sharp identification region for the parameters of the best linear predictor of <math>\ey|\ex</math> is | Under the assumptions of Identification [[#IP:param_pred_interval |Problem]], the sharp identification region for the parameters of the best linear predictor of <math>\ey|\ex</math> is | ||
Line 718: | Line 712: | ||
</math> | </math> | ||
with <math>\E_\sP\eG</math> the Aumann (or selection) expectation of <math>\eG</math> as in [[guide:379e0dcd67#def:sel-exp |Definition]]. | with <math>\E_\sP\eG</math> the Aumann (or selection) expectation of <math>\eG</math> as in [[guide:379e0dcd67#def:sel-exp |Definition]]. | ||
| | |||
By [[guide:379e0dcd67#thr:artstein |Theorem]], <math>(\tilde\ey,\tilde\ex)\in(\eY\times\ex)</math> (up to an ordered coupling as discussed in [[guide:379e0dcd67#app:RCS |Appendix]]), if and only if the distribution of <math>(\tilde\ey,\tilde\ex)</math> belongs to <math>\idr{\sQ(\ey,\ex)}</math>. | By [[guide:379e0dcd67#thr:artstein |Theorem]], <math>(\tilde\ey,\tilde\ex)\in(\eY\times\ex)</math> (up to an ordered coupling as discussed in [[guide:379e0dcd67#app:RCS |Appendix]]), if and only if the distribution of <math>(\tilde\ey,\tilde\ex)</math> belongs to <math>\idr{\sQ(\ey,\ex)}</math>. | ||
The result follows. | The result follows. | ||
}} | |||
In either representation \eqref{eq:manski_blp} or \eqref{eq:ThetaI_BLP}, <math>\idr{\theta}</math> is the collection of best linear predictors for each selection of <math>\eY</math>.<ref group="Notes" >Under our assumption that <math>\cY</math> is a bounded interval, all the selections of <math>\eY</math> are integrable. | In either representation \eqref{eq:manski_blp} or \eqref{eq:ThetaI_BLP}, <math>\idr{\theta}</math> is the collection of best linear predictors for each selection of <math>\eY</math>.<ref group="Notes" >Under our assumption that <math>\cY</math> is a bounded interval, all the selections of <math>\eY</math> are integrable. {{ref|name=ber:mol08}} consider the more general case where <math>\cY</math> is not required to be bounded.</ref> | ||
Why should one bother with the representation in \eqref{eq:ThetaI_BLP}? | Why should one bother with the representation in \eqref{eq:ThetaI_BLP}? | ||
The reason is that <math>\idr{\theta}</math> is a convex set, as it can be evinced from representation \eqref{eq:ThetaI_BLP}: <math>\eG</math> has almost surely convex realizations that are segments and the Aumann expectation of a convex set is convex.<ref group="Notes" >In <math>\R^2</math> in our example, in <math>\R^d</math> if <math>\ex</math> is a <math>d-1</math> vector and the predictor includes an intercept.</ref> | The reason is that <math>\idr{\theta}</math> is a convex set, as it can be evinced from representation \eqref{eq:ThetaI_BLP}: <math>\eG</math> has almost surely convex realizations that are segments and the Aumann expectation of a convex set is convex.<ref group="Notes" >In <math>\R^2</math> in our example, in <math>\R^d</math> if <math>\ex</math> is a <math>d-1</math> vector and the predictor includes an intercept.</ref> | ||
Line 734: | Line 728: | ||
\end{align} | \end{align} | ||
</math> | </math> | ||
where <math>f(\ex,u)\equiv [1~\ex]\Sigma_\sP^{-1}u</math>.<ref group="Notes" >See | where <math>f(\ex,u)\equiv [1~\ex]\Sigma_\sP^{-1}u</math>.<ref group="Notes" >See {{ref|name=ber:mol08}}{{rp|at=p. 808}} and {{ref|name=bon:mag:mau12}}{{rp|at=p. 1136}}.</ref> | ||
The characterization in \eqref{eq:supfun:BLP} results from [[guide:379e0dcd67#thr:exp-supp |Theorem]], which yields <math>h_{\idr{\theta}}(u)=h_{\Sigma_\sP^{-1} \E_\sP\eG}(u)=\E_\sP h_{\Sigma_\sP^{-1} \eG}(u)</math>, and the fact that <math>\E_\sP h_{\Sigma_\sP^{-1} \eG}(u)</math> equals the expression in \eqref{eq:supfun:BLP}. | The characterization in \eqref{eq:supfun:BLP} results from [[guide:379e0dcd67#thr:exp-supp |Theorem]], which yields <math>h_{\idr{\theta}}(u)=h_{\Sigma_\sP^{-1} \E_\sP\eG}(u)=\E_\sP h_{\Sigma_\sP^{-1} \eG}(u)</math>, and the fact that <math>\E_\sP h_{\Sigma_\sP^{-1} \eG}(u)</math> equals the expression in \eqref{eq:supfun:BLP}. | ||
As I discuss in [[guide:6d1a428897#sec:inference |Section]] below, because the support function fully characterizes the boundary of <math>\idr{\theta}</math>, \eqref{eq:supfun:BLP} allows for a simple sample analog estimator, and for inference procedures with desirable properties. | As I discuss in [[guide:6d1a428897#sec:inference |Section]] below, because the support function fully characterizes the boundary of <math>\idr{\theta}</math>, \eqref{eq:supfun:BLP} allows for a simple sample analog estimator, and for inference procedures with desirable properties. | ||
It also immediately yields sharp bounds on linear combinations of <math>\theta</math> by judicious choice of <math>u</math>.<ref group="Notes" >For example, in the case that <math>\ex</math> is a scalar, sharp bounds on <math>\theta_1</math> can be obtained by choosing <math>u=[0~1]^\top</math> and <math>u=[0~-1]^\top</math>, which yield <math>\theta_1\in[\theta_{1L},\theta_{1U}]</math> with <math>\theta_{1L}=\min_{\ey\in[\yL,\yU]}\frac{Cov(\ex,\ey)}{Var(\ex)}=\frac{\E_\sP[(\ex-\E_\sP\ex)(\yL\one(\ex > \E_\sP\ex)+\yU\one(\ex\le\E\ex))]}{\E_\sP\ex^2-(\E_\sP\ex)^2}</math> and <math>\theta_{1U}=\max_{\ey\in[\yL,\yU]}\frac{Cov(\ex,\ey)}{Var(\ex)}=\frac{\E_\sP[(\ex-\E_\sP\ex)(\yL\one(\ex < \E_\sP\ex)+\yU\one(\ex\ge\E\ex))]}{\E_\sP\ex^2-(\E_\sP\ex)^2}</math>.</ref> | It also immediately yields sharp bounds on linear combinations of <math>\theta</math> by judicious choice of <math>u</math>.<ref group="Notes" >For example, in the case that <math>\ex</math> is a scalar, sharp bounds on <math>\theta_1</math> can be obtained by choosing <math>u=[0~1]^\top</math> and <math>u=[0~-1]^\top</math>, which yield <math>\theta_1\in[\theta_{1L},\theta_{1U}]</math> with <math>\theta_{1L}=\min_{\ey\in[\yL,\yU]}\frac{Cov(\ex,\ey)}{Var(\ex)}=\frac{\E_\sP[(\ex-\E_\sP\ex)(\yL\one(\ex > \E_\sP\ex)+\yU\one(\ex\le\E\ex))]}{\E_\sP\ex^2-(\E_\sP\ex)^2}</math> and <math>\theta_{1U}=\max_{\ey\in[\yL,\yU]}\frac{Cov(\ex,\ey)}{Var(\ex)}=\frac{\E_\sP[(\ex-\E_\sP\ex)(\yL\one(\ex < \E_\sP\ex)+\yU\one(\ex\ge\E\ex))]}{\E_\sP\ex^2-(\E_\sP\ex)^2}</math>.</ref> | ||
<ref name="sto07"></ref> and <ref name="mag:mau08"></ref> provide the same characterization as in \eqref{eq:supfun:BLP} using, respectively, direct optimization and the Frisch-Waugh-Lovell theorem. | <ref name="sto07"><span style="font-variant-caps:small-caps">Stoye, J.</span> (2007): “Bounds on Generalized Linear Predictors with Incomplete Outcome Data” ''Reliable Computing'', 13(3), 293--302.</ref> and <ref name="mag:mau08"><span style="font-variant-caps:small-caps">Magnac, T., <span style="font-variant-caps:normal">and</span> E.Maurin</span> (2008): “Partial Identification in Monotone Binary Models: Discrete Regressors and Interval Data” ''The Review of Economic Studies'', 75(3), 835--864.</ref> provide the same characterization as in \eqref{eq:supfun:BLP} using, respectively, direct optimization and the Frisch-Waugh-Lovell theorem. | ||
A natural generalization of Identification [[#IP:param_pred_interval |Problem]] allows for both outcome and covariate data to be interval valued. | A natural generalization of Identification [[#IP:param_pred_interval |Problem]] allows for both outcome and covariate data to be interval valued. | ||
{{proofcard|Identification Problem (Parametric Prediction with Interval Outcome and Covariate Data)|IP:param_pred_interval_out_cov| | |||
Maintain the same assumptions as in Identification [[#IP:param_pred_interval |Problem]], but with <math>\ex\in\cX\subset\R</math> unobservable. | Maintain the same assumptions as in Identification [[#IP:param_pred_interval |Problem]], but with <math>\ex\in\cX\subset\R</math> unobservable. | ||
Let the researcher observe <math>(\yL,\yU,\xL,\xU)</math> such that <math>\sR(\yL \leq \ey \leq \yU , \xL \leq \ex \leq \xU)=1</math>. | Let the researcher observe <math>(\yL,\yU,\xL,\xU)</math> such that <math>\sR(\yL \leq \ey \leq \yU , \xL \leq \ex \leq \xU)=1</math>. | ||
Let <math>\eX\equiv [\xL,\xU]</math> and let <math>\cX</math> be bounded. | Let <math>\eX\equiv [\xL,\xU]</math> and let <math>\cX</math> be bounded. | ||
In the absence of additional information, what can the researcher learn about the best linear predictor of <math>\ey</math> given <math>\ex=x</math>? | In the absence of additional information, what can the researcher learn about the best linear predictor of <math>\ey</math> given <math>\ex=x</math>? | ||
|}} | |||
Abstractly, <math>\idr{\theta}</math> is as given in \eqref{eq:manski_blp}, with | Abstractly, <math>\idr{\theta}</math> is as given in \eqref{eq:manski_blp}, with | ||
Line 758: | Line 751: | ||
</math> | </math> | ||
replacing \eqref{eq:Qyx} by an application of [[guide:379e0dcd67#thr:artstein |Theorem]]. | replacing \eqref{eq:Qyx} by an application of [[guide:379e0dcd67#thr:artstein |Theorem]]. | ||
While this characterization is sharp, it is cumbersome to apply in practice, see <ref name="hor:man:pon:sto03"></ref>. | While this characterization is sharp, it is cumbersome to apply in practice, see <ref name="hor:man:pon:sto03"><span style="font-variant-caps:small-caps">Horowitz, J.L., C.F. Manski, M.Ponomareva, <span style="font-variant-caps:normal">and</span> J.Stoye</span> (2003): “Computation of Bounds on Population Parameters When the Data Are Incomplete” ''Reliable Computing'', 9(6), 419--440.</ref>. | ||
On the other hand, when both <math>\ey</math> and <math>\ex</math> are perfectly observed, the best linear predictor is simply equal to the parameter vector that yields a mean zero prediction error that is uncorrelated with <math>\ex</math>. | On the other hand, when both <math>\ey</math> and <math>\ex</math> are perfectly observed, the best linear predictor is simply equal to the parameter vector that yields a mean zero prediction error that is uncorrelated with <math>\ex</math>. | ||
How can this basic observation help in the case of interval data? | How can this basic observation help in the case of interval data? | ||
The idea is that one can use the same insight applied to the set-valued data, and obtain <math>\idr{\theta}</math> as the collection of <math>\theta</math>'s for which there exists a selection <math>(\tilde{\ey},\tilde{\ex}) \in \Sel(\eY \times \eX)</math>, and associated prediction error <math>\eps_\theta=\tilde{\ey}-\theta_0-\theta_1 \tilde{\ex}</math>, satisfying <math>\E_\sP \eps_\theta=0</math> and <math>\E_\sP (\eps_\theta \tilde{\ex})=0</math> (as shown by <ref name="ber:mol:mol11" | The idea is that one can use the same insight applied to the set-valued data, and obtain <math>\idr{\theta}</math> as the collection of <math>\theta</math>'s for which there exists a selection <math>(\tilde{\ey},\tilde{\ex}) \in \Sel(\eY \times \eX)</math>, and associated prediction error <math>\eps_\theta=\tilde{\ey}-\theta_0-\theta_1 \tilde{\ex}</math>, satisfying <math>\E_\sP \eps_\theta=0</math> and <math>\E_\sP (\eps_\theta \tilde{\ex})=0</math> (as shown by <ref name="ber:mol:mol11"/>).<ref group="Notes" >Here for simplicity I suppose that both <math>\xL</math> and <math>\xU</math> have bounded support. | ||
{{ref|name=ber:mol:mol11}} do not make this simplifying assumption.</ref> | |||
To obtain the formal result, define the <math>\theta</math>-dependent set<ref group="Notes" >Note that while <math>\eG</math> is a convex set, <math>\Eps_\theta</math> is not.</ref> | To obtain the formal result, define the <math>\theta</math>-dependent set<ref group="Notes" >Note that while <math>\eG</math> is a convex set, <math>\Eps_\theta</math> is not.</ref> | ||
Line 769: | Line 762: | ||
(\tilde{\ey}-\theta_0-\theta_1 \tilde{\ex})\tilde{\ex} | (\tilde{\ey}-\theta_0-\theta_1 \tilde{\ex})\tilde{\ex} | ||
\end{pmatrix} \: : \, (\tilde{\ey},\tilde{\ex}) \in \Sel(\eY \times\eX) \right\rbrace. </math> | \end{pmatrix} \: : \, (\tilde{\ey},\tilde{\ex}) \in \Sel(\eY \times\eX) \right\rbrace. </math> | ||
{{proofcard|Theorem (Best Linear Predictor with Interval Outcome and Covariate Data)|SIR:blp_intervalYX|Under the assumptions of Identification [[#IP:param_pred_interval_out_cov |Problem]], the sharp identification region for the parameters of the best linear predictor of <math>\ey|\ex</math> is | |||
Under the assumptions of Identification [[#IP:param_pred_interval_out_cov |Problem]], the sharp identification region for the parameters of the best linear predictor of <math>\ey|\ex</math> is | |||
<math display="block"> | <math display="block"> | ||
Line 781: | Line 773: | ||
\end{align} | \end{align} | ||
</math> | </math> | ||
where <math>h_{\Eps_\theta}(u) = \max_{y\in\eY,x\in\eX} [u_1(y-\theta_0-\theta_1 x)+ u_2(yx-\theta_0 x-\theta_1 x^2)]</math> is the support function of the set <math>\Eps_\theta</math> in direction <math>u\in\Sphere</math>, see [[guide:379e0dcd67#def:sup-fun |Definition]]. | where <math>h_{\Eps_\theta}(u) = \max_{y\in\eY,x\in\eX} [u_1(y-\theta_0-\theta_1 x)+ u_2(yx-\theta_0 x-\theta_1 x^2)]</math> is the support function of the set <math>\Eps_\theta</math> in direction <math>u\in\Sphere</math>, see [[guide:379e0dcd67#def:sup-fun |Definition]].|By [[guide:379e0dcd67#thr:artstein |Theorem]], <math>(\tilde\ey,\tilde\ex)\in(\eY\times\eX)</math> (up to an ordered coupling as discussed in [[guide:379e0dcd67#app:RCS |Appendix]]), if and only if the distribution of <math>(\tilde\ey,\tilde\ex)</math> belongs to <math>\idr{\sQ(\ey,\ex)}</math>. | ||
By [[guide:379e0dcd67#thr:artstein |Theorem]], <math>(\tilde\ey,\tilde\ex)\in(\eY\times\eX)</math> (up to an ordered coupling as discussed in [[guide:379e0dcd67#app:RCS |Appendix]]), if and only if the distribution of <math>(\tilde\ey,\tilde\ex)</math> belongs to <math>\idr{\sQ(\ey,\ex)}</math>. | |||
For given <math>\theta</math>, one can find <math>(\tilde\ey,\tilde\ex)\in(\eY\times\eX)</math> such that <math>\E_\sP \eps_\theta=0</math> and <math>\E_\sP (\eps_\theta \tilde{\ex})=0</math> with <math>\eps_\theta\in\Eps_\theta</math> if and only if the zero vector belongs to <math>\E_\sP \Eps_\theta</math>. | For given <math>\theta</math>, one can find <math>(\tilde\ey,\tilde\ex)\in(\eY\times\eX)</math> such that <math>\E_\sP \eps_\theta=0</math> and <math>\E_\sP (\eps_\theta \tilde{\ex})=0</math> with <math>\eps_\theta\in\Eps_\theta</math> if and only if the zero vector belongs to <math>\E_\sP \Eps_\theta</math>. | ||
By [[guide:379e0dcd67#thr:exp-supp |Theorem]], <math>\E_\sP \Eps_\theta</math> is a convex set and by [[guide:379e0dcd67#eq:dom_Aumann |eq:dom_Aumann]], <math>\mathbf{0} \in \E_\sP \Eps_\theta</math> if and only if <math>0 \leq h_{\E_\sP \Eps_\theta}(u) \,\forall \, u \in \Ball</math>. | By [[guide:379e0dcd67#thr:exp-supp |Theorem]], <math>\E_\sP \Eps_\theta</math> is a convex set and by [[guide:379e0dcd67#eq:dom_Aumann |eq:dom_Aumann]], <math>\mathbf{0} \in \E_\sP \Eps_\theta</math> if and only if <math>0 \leq h_{\E_\sP \Eps_\theta}(u) \,\forall \, u \in \Ball</math>. | ||
The final characterization follows from [[guide:379e0dcd67#eq:supf |eq:supf]]. | The final characterization follows from [[guide:379e0dcd67#eq:supf |eq:supf]].}} | ||
The support function <math>h_{\Eps_\theta}(u)</math> is an easy to calculate convex sublinear function of <math>u</math>, regardless of whether the variables involved are continuous or discrete. | The support function <math>h_{\Eps_\theta}(u)</math> is an easy to calculate convex sublinear function of <math>u</math>, regardless of whether the variables involved are continuous or discrete. | ||
The optimization problem in ([[#eq:ThetaI:BLP |eq:ThetaI:BLP]]), determining whether <math>\theta \in \idr{\theta}</math>, is a convex program, hence easy to solve. | The optimization problem in ([[#eq:ThetaI:BLP |eq:ThetaI:BLP]]), determining whether <math>\theta \in \idr{\theta}</math>, is a convex program, hence easy to solve. | ||
See for example the CVX software by <ref name="gra:boy10"></ref>. | See for example the CVX software by <ref name="gra:boy10"><span style="font-variant-caps:small-caps">Grant, M., <span style="font-variant-caps:normal">and</span> S.Boyd</span> (2010): “{CVX}: Matlab Software for Disciplined Convex Programming, Version 1.21” available at [http://cvxr.com/cvx http://cvxr.com/cvx].</ref>. | ||
It should be noted, however, that the set <math>\idr{\theta}</math> itself is not necessarily convex. | It should be noted, however, that the set <math>\idr{\theta}</math> itself is not necessarily convex. | ||
Hence, tracing out its boundary is non-trivial. | Hence, tracing out its boundary is non-trivial. | ||
I discuss computational challenges in partial identification in [[guide:A85a6b6ff1#sec:computations |Section]]. | I discuss computational challenges in partial identification in [[guide:A85a6b6ff1#sec:computations |Section]]. | ||
I conclude this section by discussing parametric regression. | I conclude this section by discussing parametric regression. | ||
<ref name="man:tam02" | <ref name="man:tam02"/> study identification of parametric regression models under the assumptions in Identification [[#IP:man:tam02_param |Problem]]; Theorem [[#SIR:man:tam02_param |SIR-]] below reports the result. | ||
The proof is omitted because it follows immediately from the proof of Theorem [[#SIR:man:tam:nonpar |SIR-]]. | The proof is omitted because it follows immediately from the proof of Theorem [[#SIR:man:tam:nonpar |SIR-]]. | ||
{{proofcard|Identification Problem (Parametric Regression with Interval Covariate Data)|IP:man:tam02_param|Let <math>(\ey,\xL,\xU,\ew)\sim\sP</math> be observable random variables in <math>\R\times\R\times\R\times\R^d</math>, <math>d < \infty</math>, and let <math>\ex\in\R</math> be an unobservable random variable. | |||
Let <math>(\ey,\xL,\xU,\ew)\sim\sP</math> be observable random variables in <math>\R\times\R\times\R\times\R^d</math>, <math>d < \infty</math>, and let <math>\ex\in\R</math> be an unobservable random variable. | |||
Assume that the joint distribution <math>\sR</math> of <math>(\ey,\ex,\xL,\xU)</math> is such that <math>\sR(\xL\le\ex\le\xU)=1</math> and <math>\E_{\sR}(\ey|\ew,\ex,\xL,\xU)=\E_\sQ(\ey|\ew,\ex)</math>. | Assume that the joint distribution <math>\sR</math> of <math>(\ey,\ex,\xL,\xU)</math> is such that <math>\sR(\xL\le\ex\le\xU)=1</math> and <math>\E_{\sR}(\ey|\ew,\ex,\xL,\xU)=\E_\sQ(\ey|\ew,\ex)</math>. | ||
Suppose that <math>\E_\sQ(\ey|\ew,\ex)=f(\ew,\ex;\theta)</math>, with <math>f:\R^d\times\R\times\Theta \mapsto \R</math> a known function such that for each <math>w\in\R</math> and <math>\theta\in\Theta</math>, <math>f(w,x;\theta)</math> is weakly increasing in <math>x</math>. | Suppose that <math>\E_\sQ(\ey|\ew,\ex)=f(\ew,\ex;\theta)</math>, with <math>f:\R^d\times\R\times\Theta \mapsto \R</math> a known function such that for each <math>w\in\R</math> and <math>\theta\in\Theta</math>, <math>f(w,x;\theta)</math> is weakly increasing in <math>x</math>. In the absence of additional information, what can the researcher learn about <math>\theta</math>?|}} | ||
In the absence of additional information, what can the researcher learn about <math>\theta</math>? | |||
{{proofcard|Theorem (Parametric Regression with Interval Covariate Data)|SIR:man:tam02_param|Under the Assumptions of Identification [[#IP:man:tam02_param |Problem]], the sharp identification region for <math>\theta</math> is | |||
Under the Assumptions of Identification [[#IP:man:tam02_param |Problem]], the sharp identification region for <math>\theta</math> is | |||
<math display="block"> | <math display="block"> | ||
Line 814: | Line 798: | ||
\idr{\theta}=\big\{\vartheta\in \Theta: f(\ew,\xL;\vartheta)\le \E_\sP(\ey|\ew,\xL,\xU) \le f(\ew,\xU;\vartheta),~(\ew,\xL,\xU)\text{-a.s.} \big\}.\label{eq:ThetaI_man:tam02_param} | \idr{\theta}=\big\{\vartheta\in \Theta: f(\ew,\xL;\vartheta)\le \E_\sP(\ey|\ew,\xL,\xU) \le f(\ew,\xU;\vartheta),~(\ew,\xL,\xU)\text{-a.s.} \big\}.\label{eq:ThetaI_man:tam02_param} | ||
\end{multline} | \end{multline} | ||
</math> | </math>|}} | ||
<ref name="auc:bug:hot17"><span style="font-variant-caps:small-caps">Aucejo, E.M., F.A. Bugni, <span style="font-variant-caps:normal">and</span> V.J. Hotz</span> (2017): “Identification and inference on regressions with missing covariate data” ''Econometric Theory'', 33(1).</ref> study Identification [[#IP:man:tam02_param |Problem]] for the case of missing covariate data ''without'' imposing the mean independence restriction of <ref name="man:tam02"/> (Assumption MI in Identification [[#IP:interval_covariate |Problem]]). | |||
<ref name="auc:bug:hot17"></ref> study Identification [[#IP:man:tam02_param |Problem]] for the case of missing covariate data ''without'' imposing the mean independence restriction of <ref name="man:tam02" | |||
As discussed in [[#foot:auc:bug:hot17 |footnote]], restriction MI is undesirable in this context because it implies the assumption that data are missing at random. | As discussed in [[#foot:auc:bug:hot17 |footnote]], restriction MI is undesirable in this context because it implies the assumption that data are missing at random. | ||
<ref name="auc:bug:hot17" | <ref name="auc:bug:hot17"/> characterize <math>\idr{\theta}</math> under the weaker assumptions, but face the problem that this characterization is usually too complex to compute or to use for inference. | ||
They therefore provide outer regions that are easier to compute, and they show that these regions are informative and relatively easy to use. | They therefore provide outer regions that are easier to compute, and they show that these regions are informative and relatively easy to use. | ||
==<span id="subsec:meas_error"></span>Measurement Error and Data Combination== | |||
One of the first examples of bounding analysis appears in <ref name="fri34"></ref>, to assess the impact in linear regression of covariate measurement error. | One of the first examples of bounding analysis appears in <ref name="fri34"><span style="font-variant-caps:small-caps">Frisch, R.</span> (1934): ''Statistical Confluence Analysis by Means of Complete Regression Systems'', Okonomiske Institutt Oslo: Publikasjon. Universitetets {\O}konomiske Instituut.</ref>, to assess the impact in linear regression of covariate measurement error. | ||
This analysis was substantially extended in <ref name="gil:lea83"></ref>, <ref name="kle:lea84"></ref>, and <ref name="lea87"></ref>. | This analysis was substantially extended in <ref name="gil:lea83"><span style="font-variant-caps:small-caps">Gilstein, C.Z., <span style="font-variant-caps:normal">and</span> E.E. Leamer</span> (1983): “Robust Sets of Regression Estimates” ''Econometrica'', 51(2), 321--333.</ref>, <ref name="kle:lea84"><span style="font-variant-caps:small-caps">Klepper, S., <span style="font-variant-caps:normal">and</span> E.E. Leamer</span> (1984): “Consistent Sets of Estimates for Regressions with Errors in All Variables” ''Econometrica'', 52(1), 163--183.</ref>, and <ref name="lea87"><span style="font-variant-caps:small-caps">Leamer, E.E.</span> (1987): “Errors in Variables in Linear Systems” ''Econometrica'', 55(4), 893--909.</ref>. | ||
The more recent literature in partial identification has provided important advances to learn features of probability distributions when the observed variables are error-ridden measures of the variables of interest. | The more recent literature in partial identification has provided important advances to learn features of probability distributions when the observed variables are error-ridden measures of the variables of interest. | ||
Here I briefly mention some of the papers in this literature, and refer to Chapter '''XXX''' in this Volume by <ref name="sch19"></ref> for a thorough treatment of identification and inference with mismeasured and unobserved variables. | Here I briefly mention some of the papers in this literature, and refer to Chapter '''XXX''' in this Volume by <ref name="sch19"><span style="font-variant-caps:small-caps">Schennach, S.M.</span> (2019): “Mismeasured and unobserved variables” in ''Handbook of Econometrics''. Elsevier.</ref> for a thorough treatment of identification and inference with mismeasured and unobserved variables. | ||
In an influential paper, <ref name="hor:man95"></ref> study what can be learned about features of the distribution of <math>\ey|\ex</math> in the presence of contaminated or corrupted outcome data. | In an influential paper, <ref name="hor:man95"><span style="font-variant-caps:small-caps">Horowitz, J.L., <span style="font-variant-caps:normal">and</span> C.F. Manski</span> (1995): “Identification and Robustness with Contaminated and Corrupted Data” ''Econometrica'', 63(2), 281--302.</ref> study what can be learned about features of the distribution of <math>\ey|\ex</math> in the presence of contaminated or corrupted outcome data. | ||
Whereas a contaminated sampling model assumes that data errors are statistically independent of sample realizations from the population of interest, the corrupted sampling model does not. | Whereas a contaminated sampling model assumes that data errors are statistically independent of sample realizations from the population of interest, the corrupted sampling model does not. | ||
These models are regularly used in the important literature on robust estimation (e.g., <ref name="hub64"></ref><ref name="hub04"></ref><ref name="ham:ron:rou:sta11"></ref>). | These models are regularly used in the important literature on robust estimation (e.g., <ref name="hub64"><span style="font-variant-caps:small-caps">Huber, P.J.</span> (1964): “Robust Estimation of a Location Parameter” ''The Annals of Mathematical Statistics'', 35(1), 73--101.</ref><ref name="hub04"><span style="font-variant-caps:small-caps">Huber, P.J.</span> (2004): ''Robust Statistics'', Wiley Series in Probability and Statistics - Applied Probability and Statistics Section Series. Wiley.</ref><ref name="ham:ron:rou:sta11"><span style="font-variant-caps:small-caps">Hampel, F.R., E.M. Ronchetti, P.J. Rousseeuw, <span style="font-variant-caps:normal">and</span> W.A. Stahel</span> (2011): ''Robust Statistics: The Approach Based on Influence Functions''. Wiley.</ref>). | ||
However, the goal of that literature is to characterize how point estimators of population parameters behave when data errors are generated in specified ways. | However, the goal of that literature is to characterize how point estimators of population parameters behave when data errors are generated in specified ways. | ||
As such, the inference problem is approached ex-ante: before collecting the data, one looks for point estimators that are not greatly affected by error. | As such, the inference problem is approached ex-ante: before collecting the data, one looks for point estimators that are not greatly affected by error. | ||
The question addressed by <ref name="hor:man95" | The question addressed by <ref name="hor:man95"/> is conceptually distinct. | ||
It asks what can be learned about specific population parameters ex-post, that is, after the data has been collected. | It asks what can be learned about specific population parameters ex-post, that is, after the data has been collected. | ||
For example, whereas the mean is well known not to be a robust estimator in the presence of contaminated data, <ref name="hor:man95" | For example, whereas the mean is well known not to be a robust estimator in the presence of contaminated data, <ref name="hor:man95"/> show that it can be (non-trivially) bounded provided the probability of contamination is strictly less than one. | ||
<ref name="dom:she04"></ref><ref name="dom:she05"></ref> and <ref name="kre:pep07"></ref><ref name="kre:pep08"></ref> extend the results of <ref name="hor:man95" | <ref name="dom:she04"><span style="font-variant-caps:small-caps">Dominitz, J., <span style="font-variant-caps:normal">and</span> R.P. Sherman</span> (2004): “Sharp bounds under contaminated or corrupted sampling with verification, with an application to environmental pollutant data” ''Journal of Agricultural, Biological, and Environmental Statistics'', 9(3), 319--338.</ref><ref name="dom:she05"><span style="font-variant-caps:small-caps">Dominitz, J., <span style="font-variant-caps:normal">and</span> R.P. Sherman</span> (2005): “Identification and estimation of bounds on school performance measures: a nonparametric analysis of a mixture model with verification” ''Journal of Applied Econometrics'', 21(8), 1295--1326.</ref> and <ref name="kre:pep07"><span style="font-variant-caps:small-caps">Kreider, B., <span style="font-variant-caps:normal">and</span> J.V. Pepper</span> (2007): “Disability and Employment: Reevaluating the Evidence in Light of Reporting Errors” ''Journal of the American Statistical Association'', 102(478), 432--441.</ref><ref name="kre:pep08"><span style="font-variant-caps:small-caps">Kreider, B., <span style="font-variant-caps:normal">and</span> J.Pepper</span> (2008): “Inferring disability status from corrupt data” ''Journal of Applied Econometrics'', 23(3), 329--349.</ref> extend the results of <ref name="hor:man95"/> to allow for (partial) verification of the distribution from which the data are drawn. | ||
They apply the resulting sharp bounds to learn about school performance when the observed test scores may not be valid for all students. | They apply the resulting sharp bounds to learn about school performance when the observed test scores may not be valid for all students. | ||
<ref name="mol08"></ref> provides sharp bounds on the distribution of a misclassified outcome variable under an array of different assumptions on the extent and type of misclassification. | <ref name="mol08"><span style="font-variant-caps:small-caps">Molinari, F.</span> (2008): “Partial identification of probability distributions with misclassified data” ''Journal of Econometrics'', 144(1), 81 -- 117.</ref> provides sharp bounds on the distribution of a misclassified outcome variable under an array of different assumptions on the extent and type of misclassification. | ||
A completely different problem is that of data combination. | A completely different problem is that of data combination. | ||
Applied economists often face the problem that no single data set contains all the variables that are necessary to conduct inference on a population of interest. | Applied economists often face the problem that no single data set contains all the variables that are necessary to conduct inference on a population of interest. | ||
When this is the case, they need to integrate the information contained in different samples; for example, they might need to combine survey data with administrative data (see <ref name="rid:mof07"></ref>{{rp|at=for a | When this is the case, they need to integrate the information contained in different samples; for example, they might need to combine survey data with administrative data (see <ref name="rid:mof07"><span style="font-variant-caps:small-caps">Ridder, G., <span style="font-variant-caps:normal">and</span> R.Moffitt</span> (2007): “Chapter 75 -- The Econometrics of Data Combination” in ''Handbook of Econometrics'', ed. by J.J. Heckman, <span style="font-variant-caps:normal">and</span> E.E. Leamer, vol.6, pp. 5469 -- 5547. Elsevier.</ref>{{rp|at=for a | ||
survey of the econometrics of data combination}}). | survey of the econometrics of data combination}}). | ||
From a methodological perspective, the problem is that while the samples being combined might contain some common variables, other variables belong only to one of the samples. | From a methodological perspective, the problem is that while the samples being combined might contain some common variables, other variables belong only to one of the samples. | ||
Line 846: | Line 830: | ||
Formally, the problem is that one observes data that identify the joint distributions <math>\sP(\ey,\ex)</math> and <math>\sP(\ex,\ew)</math>, but not data that identifies the joint distribution <math>\sQ(\ey,\ex,\ew)</math> whose features one wants to learn. | Formally, the problem is that one observes data that identify the joint distributions <math>\sP(\ey,\ex)</math> and <math>\sP(\ex,\ew)</math>, but not data that identifies the joint distribution <math>\sQ(\ey,\ex,\ew)</math> whose features one wants to learn. | ||
The literature on ''statistical matching'' has aimed at using the | The literature on ''statistical matching'' has aimed at using the | ||
common variable(s) <math>\ex</math> as a bridge to create synthetic records containing <math>(\ey,\ex,\ew)</math> (see, e.g., <ref name="okn72"></ref>{{rp|at=for an early contribution}}). | common variable(s) <math>\ex</math> as a bridge to create synthetic records containing <math>(\ey,\ex,\ew)</math> (see, e.g., <ref name="okn72"><span style="font-variant-caps:small-caps">Okner, B.</span> (1972): “Constructing A New Data Base From Existing Microdata Sets: The 1966 Merge File” ''Annals of Economic and Social Measurement'', 1(3), 325--362.</ref>{{rp|at=for an early contribution}}). | ||
As <ref name="sim72"></ref> points out, the inherent assumption at the base of | As <ref name="sim72"><span style="font-variant-caps:small-caps">Sims, C.A.</span> (1972): “Comments and Rejoinder On Okner (1972)” ''Annals of Economic and Social Measurement'', 1(3), 343--345 and 355--357.</ref> points out, the inherent assumption at the base of | ||
statistical matching is that conditional on <math>\ex</math>, <math>\ey</math> and <math>\ew</math> are | statistical matching is that conditional on <math>\ex</math>, <math>\ey</math> and <math>\ew</math> are | ||
independent. | independent. | ||
Line 854: | Line 838: | ||
While it does guarantee point identification of features of the | While it does guarantee point identification of features of the | ||
conditional distributions <math>\sQ(\ey|\ex,\ew)</math>, it often finds very little justification in practice. | conditional distributions <math>\sQ(\ey|\ex,\ew)</math>, it often finds very little justification in practice. | ||
Early on, <ref name="dun:dav53"></ref> provided numerical illustrations on how one can bound the object of interest, when both <math>\ey</math> and <math>\ew</math> are binary variables. | Early on, <ref name="dun:dav53"><span style="font-variant-caps:small-caps">Duncan, O.D., <span style="font-variant-caps:normal">and</span> B.Davis</span> (1953): “An Alternative to Ecological Correlation” ''American Sociological Review'', 18(6), 665--666.</ref> provided numerical illustrations on how one can bound the object of interest, when both <math>\ey</math> and <math>\ew</math> are binary variables. | ||
<ref name="cro:man02"></ref> provide a general analysis of the problem. | <ref name="cro:man02"><span style="font-variant-caps:small-caps">Cross, P.J., <span style="font-variant-caps:normal">and</span> C.F. Manski</span> (2002): “Regressions, Short and Long” ''Econometrica'', 70(1), 357--368.</ref> provide a general analysis of the problem. | ||
They obtain bounds on the long regression <math>\E_\sQ(\ey|\ex,\ew)</math>, under the assumption that <math>\ew</math> has finite support. | They obtain bounds on the long regression <math>\E_\sQ(\ey|\ex,\ew)</math>, under the assumption that <math>\ew</math> has finite support. | ||
They show that sharp bounds on <math>\E_\sQ(\ey|\ex,\ew=w)</math> can be obtained using the results in <ref name="hor:man95" | They show that sharp bounds on <math>\E_\sQ(\ey|\ex,\ew=w)</math> can be obtained using the results in <ref name="hor:man95"/>, thereby establishing a connection with the analysis of contaminated data. | ||
They then derive sharp identification regions for <math>[\E_\sQ(\ey|\ex=x,\ew=w),x\in\cX,w\in\cW]</math>. | They then derive sharp identification regions for <math>[\E_\sQ(\ey|\ex=x,\ew=w),x\in\cX,w\in\cW]</math>. | ||
They show that these bounds are sharp when <math>\ey</math> has finite support, and <ref name="mol:pes06"></ref> establish sharpness without this restriction. | They show that these bounds are sharp when <math>\ey</math> has finite support, and <ref name="mol:pes06"><span style="font-variant-caps:small-caps">Molinari, F., <span style="font-variant-caps:normal">and</span> M.Peski</span> (2006): “Generalization of a Result on ``Regressions, short and long"” ''Econometric Theory'', 22(1), 159--163.</ref> establish sharpness without this restriction. | ||
<ref name="fan:she:shu14"></ref> address the question of what can be learned about counterfactual distributions and treatment effects under the data scenario just described, but with <math>\ex</math> replaced by <math>\es</math>, a binary indicator for the received treatment (using the notation of the previous section). | <ref name="fan:she:shu14"><span style="font-variant-caps:small-caps">Fan, Y., R.Sherman, <span style="font-variant-caps:normal">and</span> M.Shum</span> (2014): “Identifying Treatment Effects Under Data Combination” ''Econometrica'', 82(2), 811--822.</ref> address the question of what can be learned about counterfactual distributions and treatment effects under the data scenario just described, but with <math>\ex</math> replaced by <math>\es</math>, a binary indicator for the received treatment (using the notation of the previous section). | ||
In this case, the exogenous selection assumption (conditional on <math>\ew</math>) does not suffice for point identification of the objects of interest. | In this case, the exogenous selection assumption (conditional on <math>\ew</math>) does not suffice for point identification of the objects of interest. | ||
The authors derive, however, sharp bounds on these quantities using monotone rearrangement inequalities. | The authors derive, however, sharp bounds on these quantities using monotone rearrangement inequalities. | ||
<ref name="pac17"></ref> provides partial identification results for the coefficients in the linear projection of <math>\ey</math> on <math>(\ex,\ew)</math>. | <ref name="pac17"><span style="font-variant-caps:small-caps">Pacini, D.</span> (2017): “Two-sample least squares projection” ''Econometric Reviews'', 38(1), 95--123.</ref> provides partial identification results for the coefficients in the linear projection of <math>\ey</math> on <math>(\ex,\ew)</math>. | ||
==<span id="subsec:applications_PIPD"></span>Further Theoretical Advances and Empirical Applications== | |||
In order to discuss the partial identification approach to learning features of probability distributions in some level of detail while keeping this chapter to a manageable length, I have focused on a selection of papers. | In order to discuss the partial identification approach to learning features of probability distributions in some level of detail while keeping this chapter to a manageable length, I have focused on a selection of papers. | ||
In this section I briefly mention several other excellent theoretical contributions that could be discussed more closely, as well as several papers that have applied partial identification analysis to answer important empirical questions. | In this section I briefly mention several other excellent theoretical contributions that could be discussed more closely, as well as several papers that have applied partial identification analysis to answer important empirical questions. | ||
While selectively observed data are commonplace in observational studies, in randomized experiments subjects are randomly placed in designated treatment groups conditional on <math>\ex</math>, so that the assumption of exogenous selection is satisfied with respect to the assigned treatment. | While selectively observed data are commonplace in observational studies, in randomized experiments subjects are randomly placed in designated treatment groups conditional on <math>\ex</math>, so that the assumption of exogenous selection is satisfied with respect to the assigned treatment. | ||
Yet, identification of some highly policy relevant parameters can remain elusive in the absence of strong assumptions. | Yet, identification of some highly policy relevant parameters can remain elusive in the absence of strong assumptions. | ||
One challenge results from noncompliance, where individuals' received treatments differs from the randomly assigned ones. | One challenge results from noncompliance, where individuals' received treatments differs from the randomly assigned ones. | ||
<ref name="bal:pea97"></ref> derive sharp bounds on the ATE in this context, when <math>\cY=\T=\{0,1\}</math>. | <ref name="bal:pea97"><span style="font-variant-caps:small-caps">Balke, A., <span style="font-variant-caps:normal">and</span> J.Pearl</span> (1997): “Bounds on Treatment Effects From Studies With Imperfect Compliance” ''Journal of the American Statistical Association'', 92(439), 1171--1176.</ref> derive sharp bounds on the ATE in this context, when <math>\cY=\T=\{0,1\}</math>. | ||
Even if one is interested in the intention-to-treat parameter, selectively observed data may continue to be a problem. | Even if one is interested in the intention-to-treat parameter, selectively observed data may continue to be a problem. | ||
For example, <ref name="lee09"></ref> studies the wage effects of the Job Corps training program, which randomly assigns eligibility to participate in the program. | For example, <ref name="lee09"><span style="font-variant-caps:small-caps">Lee, D.S.</span> (2009): “Training, Wages, and Sample Selection: Estimating Sharp Bounds on Treatment Effects” ''The Review of Economic Studies'', 76(3), 1071--1102.</ref> studies the wage effects of the Job Corps training program, which randomly assigns eligibility to participate in the program. | ||
Individuals randomized to be eligible were not compelled to receive treatment, hence <ref name="lee09" | Individuals randomized to be eligible were not compelled to receive treatment, hence <ref name="lee09"/> focuses on the intention-to-treat effect. | ||
Because wages are only observable when individuals are employed, a selection problem persists despite the random assignment of eligibility to treatment, as employment status may be affected by the training program. | Because wages are only observable when individuals are employed, a selection problem persists despite the random assignment of eligibility to treatment, as employment status may be affected by the training program. | ||
<ref name="lee09" | <ref name="lee09"/> obtains sharp bounds on the intention-to-treat effect, through a trimming procedure that leverages results in <ref name="hor:man95"/>. | ||
<ref name="mol08MT"></ref> analyzes the problem of identification of the ATE and other treatment effects, when the received treatment is unobserved for a subset of the population. | <ref name="mol08MT"><span style="font-variant-caps:small-caps">Molinari, F.</span> (2010): “Missing Treatments” ''Journal of Business and Economic Statistics'', 28(1), 82--95.</ref> analyzes the problem of identification of the ATE and other treatment effects, when the received treatment is unobserved for a subset of the population. | ||
Missing treatment data may be due to item or survey nonresponse in observational studies, or noncompliance with randomly assigned treatments that are not directly monitored. | Missing treatment data may be due to item or survey nonresponse in observational studies, or noncompliance with randomly assigned treatments that are not directly monitored. | ||
She derives sharp worst case bounds leveraging results in <ref name="hor:man95" | She derives sharp worst case bounds leveraging results in <ref name="hor:man95"/>, and she shows that these are a function of the available prior information on the distribution of missing treatments. | ||
If the response function is assumed monotone as in \eqref{eq:MTR:treat}, she obtains informative bounds without restrictions on the distribution of missing treatments. | If the response function is assumed monotone as in \eqref{eq:MTR:treat}, she obtains informative bounds without restrictions on the distribution of missing treatments. | ||
Even randomly assigned treatments and perfect compliance with no missing data may not suffice for point identification of all policy relevant parameters. | Even randomly assigned treatments and perfect compliance with no missing data may not suffice for point identification of all policy relevant parameters. | ||
Important examples are given by <ref name="hec:smi:cle97"></ref> and <ref name="man97:mixing"></ref>. | Important examples are given by <ref name="hec:smi:cle97"><span style="font-variant-caps:small-caps">Heckman, J.J., J.Smith, <span style="font-variant-caps:normal">and</span> N.Clements</span> (1997): “Making the Most Out of Programme Evaluations and Social Experiments: Accounting for Heterogeneity in Programme Impacts” ''The Review of Economic Studies'', 64(4), 487--535.</ref> and <ref name="man97:mixing"><span style="font-variant-caps:small-caps">Manski, C.F.</span> (1997a): “The Mixing Problem in Programme Evaluation” ''The Review of Economic Studies'', 64(4), 537--553.</ref>. | ||
<ref name="hec:smi:cle97" | <ref name="hec:smi:cle97"/> show that features of the joint distribution of the potential outcomes of treatment and control, including the distribution of treatment effects impacts, cannot be point identified in the absence of strong restrictions. | ||
This is because although subjects are randomized to treatment and control, nobody's outcome is observed under both states. | This is because although subjects are randomized to treatment and control, nobody's outcome is observed under both states. | ||
Nonetheless, the authors obtain bounds for the functionals of interest. | Nonetheless, the authors obtain bounds for the functionals of interest. | ||
<ref name="mul18"></ref> derives related bounds on the probability that the potential outcome of one treatment is larger than that of the other treatment, and applies these results to health economics problems. | <ref name="mul18"><span style="font-variant-caps:small-caps">Mullahy, J.</span> (2018): “Individual results may vary: Inequality-probability bounds for some health-outcome treatment effects” ''Journal of Health Economics'', 61, 151 -- 162.</ref> derives related bounds on the probability that the potential outcome of one treatment is larger than that of the other treatment, and applies these results to health economics problems. | ||
<ref name="man97:mixing" | <ref name="man97:mixing"/> shows that features of outcome distributions under treatment rules in which treatment may vary within groups cannot be point identified in the absence of strong restrictions. | ||
This is because data resulting from randomized experiments with perfect compliance allow for point identification of the outcome distributions under treatment rules that assign all persons with the same <math>\ex</math> to the same treatment group. | This is because data resulting from randomized experiments with perfect compliance allow for point identification of the outcome distributions under treatment rules that assign all persons with the same <math>\ex</math> to the same treatment group. | ||
However, such data only allow for partial identification of outcome distributions under rules in which treatment may vary within groups. | However, such data only allow for partial identification of outcome distributions under rules in which treatment may vary within groups. | ||
<ref name="man97:mixing" | <ref name="man97:mixing"/> derives sharp bounds for functionals of these distributions. | ||
Analyses of data resulting from natural experiments also face identification challenges. | Analyses of data resulting from natural experiments also face identification challenges. | ||
<ref name="hot:mul:san97"></ref> study what can be learned about treatment effects when one uses a contaminated instrumental variable, i.e. when a mean-independence assumption holds in a population of interest, but the observed population is a mixture of the population of interest and one in which the assumption doesn't hold. | <ref name="hot:mul:san97"><span style="font-variant-caps:small-caps">Hotz, V.J., C.H. Mullin, <span style="font-variant-caps:normal">and</span> S.G. Sanders</span> (1997): “Bounding Causal Effects Using Data From a Contaminated Natural Experiment: Analysing the Effects of Teenage Chilbearing” ''The Review of Economic Studies'', 64(4), 575--603.</ref> study what can be learned about treatment effects when one uses a contaminated instrumental variable, i.e. when a mean-independence assumption holds in a population of interest, but the observed population is a mixture of the population of interest and one in which the assumption doesn't hold. | ||
They extend the results of <ref name="hor:man95" | They extend the results of <ref name="hor:man95"/> to learn about the causal effect of teenage childbearing on a teen mother's subsequent outcomes, using the natural experiment of miscarriages to form an instrumental variable for teen births. | ||
This instrument is contaminated because miscarriges may not occur randomly for a subset of the population (e.g., higher miscarriage rates are associated with smoking and drinking, and these behaviors may be correlated with the outcomes of interest). | This instrument is contaminated because miscarriges may not occur randomly for a subset of the population (e.g., higher miscarriage rates are associated with smoking and drinking, and these behaviors may be correlated with the outcomes of interest). | ||
Of course, analyses of selectively observed data present many challenges, including but not limited to the ones described in Section [[#subsec:missing_data |Selectively Observed Data]]. | Of course, analyses of selectively observed data present many challenges, including but not limited to the ones described in Section [[#subsec:missing_data |Selectively Observed Data]]. | ||
<ref name="ath:imb06"></ref> generalize the difference-in-difference (DID) design to a ''changes-in-changes'' (CIC) model, where the distribution of the unobservables is allowed to vary across groups, but not overtime within groups, and the additivity and linearity assumptions of the DID are dispensed with. | <ref name="ath:imb06"><span style="font-variant-caps:small-caps">Athey, S., <span style="font-variant-caps:normal">and</span> G.W. Imbens</span> (2006): “Identification and Inference in Nonlinear Difference-in-Differences Models” ''Econometrica'', 74(2), 431--497.</ref> generalize the difference-in-difference (DID) design to a ''changes-in-changes'' (CIC) model, where the distribution of the unobservables is allowed to vary across groups, but not overtime within groups, and the additivity and linearity assumptions of the DID are dispensed with. | ||
For the case that the outcomes have a continuous distribution, <ref name="ath:imb06" | For the case that the outcomes have a continuous distribution, <ref name="ath:imb06"/> provide conditions for point identification of the entire counterfactual distribution of effects of the treatment on the treatment group as well as the distribution of effects of the treatment on the control group, without restricting how these distributions differ from each other. | ||
For the case that the outcome variables are discrete, they provide partial identification results, as well as additional conditions compared to their baseline model under which point identification attains. | For the case that the outcome variables are discrete, they provide partial identification results, as well as additional conditions compared to their baseline model under which point identification attains. | ||
Motivated by the question of whether the age-adjusted mortality rate from cancer in 2000 was lower than that in the early 1970s, <ref name="hon:lle06"></ref> study partial identification of competing risk models (see <ref name="pet76"></ref>{{rp|at=for earlier partial identification results}}). | |||
Motivated by the question of whether the age-adjusted mortality rate from cancer in 2000 was lower than that in the early 1970s, <ref name="hon:lle06"><span style="font-variant-caps:small-caps">Honoré, B.E., <span style="font-variant-caps:normal">and</span> A.Lleras-Muney</span> (2006): “Bounds in Competing Risks Models and the War on Cancer” ''Econometrica'', 74(6), 1675--1698.</ref> study partial identification of competing risk models (see <ref name="pet76"><span style="font-variant-caps:small-caps">Peterson, A.V.</span> (1976): “Bounds for a Joint Distribution Function with Fixed Sub-Distribution Functions: Application to Competing Risks” ''Proceedings of the National Academy of Sciences of the United States of America'', 73(1), 11--13.</ref>{{rp|at=for earlier partial identification results}}). | |||
To answer this question, they need to contend with the fact that mortality rate from cardiovascular disease declined substantially over the same period of time, so that individuals that in the early 1970s might have died from cardiovascular disease before being diagnosed with cancer, do not in 2000. | To answer this question, they need to contend with the fact that mortality rate from cardiovascular disease declined substantially over the same period of time, so that individuals that in the early 1970s might have died from cardiovascular disease before being diagnosed with cancer, do not in 2000. | ||
In this context, it is important to carry out the analysis without assuming that the underlying risks are independent. | In this context, it is important to carry out the analysis without assuming that the underlying risks are independent. | ||
<ref name="hon:lle06" | <ref name="hon:lle06"/> show that bounds for the parameters of interest can be obtained as the solution to linear programming problems. | ||
The estimated bounds suggest much larger improvements in cancer mortality rates than previously estimated. | The estimated bounds suggest much larger improvements in cancer mortality rates than previously estimated. | ||
<ref name="blu:gos:ich:meg07"></ref> use UK data to study changes over time in the distribution of male and female wages, and in wage inequality. | <ref name="blu:gos:ich:meg07"><span style="font-variant-caps:small-caps">Blundell, R., A.Gosling, H.Ichimura, <span style="font-variant-caps:normal">and</span> C.Meghir</span> (2007): “Changes in the Distribution of Male and Female Wages Accounting for Employment Composition Using Bounds” ''Econometrica'', 75(2), 323--363.</ref> use UK data to study changes over time in the distribution of male and female wages, and in wage inequality. | ||
Because the composition of the workforce changes over time, it is difficult to disentangle that effect from changes in the distribution of wages, given that the latter are observed only for people in the workforce. | Because the composition of the workforce changes over time, it is difficult to disentangle that effect from changes in the distribution of wages, given that the latter are observed only for people in the workforce. | ||
<ref name="blu:gos:ich:meg07" | <ref name="blu:gos:ich:meg07"/> begin their empirical analysis by reporting worst case bounds (as in <ref name="man94"/>) on the CDF of wages conditional on covariates. | ||
They then consider various restrictions on treatment selection, e.g., a first order stochastic dominance assumption according to which people with higher wages are more likely to work, and derive tighter bounds under this assumption (and under weaker ones). | They then consider various restrictions on treatment selection, e.g., a first order stochastic dominance assumption according to which people with higher wages are more likely to work, and derive tighter bounds under this assumption (and under weaker ones). | ||
Finally, they bring to bear shape restrictions. | Finally, they bring to bear shape restrictions. | ||
At each step of the analysis, they report the resulting bounds, thereby illuminating the role played by each assumption in shaping the inference. | At each step of the analysis, they report the resulting bounds, thereby illuminating the role played by each assumption in shaping the inference. | ||
<ref name="cha:che:mol:sch18"></ref> provide best linear approximations to the identification region for the quantile gender wage gap using Current Population Survey repeated cross-sections data from 1975-2001, using treatment selection assumptions in the spirit of <ref name="blu:gos:ich:meg07" | <ref name="cha:che:mol:sch18"><span style="font-variant-caps:small-caps">Chandrasekhar, A., V.Chernozhukov, F.Molinari, <span style="font-variant-caps:normal">and</span> P.Schrimpf</span> (2018): “Best linear approximations to set identified functions: with an application to the gender wage gap” CeMMAP working paper CWP09/19, available at [https://www.cemmap.ac.uk/publication/id/13913 https://www.cemmap.ac.uk/publication/id/13913].</ref> provide best linear approximations to the identification region for the quantile gender wage gap using Current Population Survey repeated cross-sections data from 1975-2001, using treatment selection assumptions in the spirit of <ref name="blu:gos:ich:meg07"/> as well as exclusion restrictions. | ||
<ref name="bha:sha:vyt12"></ref> study the effect of Swan-Ganz catheterization on subsequent mortality.<ref group="Notes" >The Swan-Ganz catheter is a device placed in patients in the intensive care unit to guide therapy.</ref> | <ref name="bha:sha:vyt12"><span style="font-variant-caps:small-caps">Bhattacharya, J., A.M. Shaikh, <span style="font-variant-caps:normal">and</span> E.Vytlacil</span> (2012): “Treatment effect bounds: An application to Swan–Ganz catheterization” ''Journal of Econometrics'', 168(2), 223 -- 243.</ref> study the effect of Swan-Ganz catheterization on subsequent mortality.<ref group="Notes" >The Swan-Ganz catheter is a device placed in patients in the intensive care unit to guide therapy.</ref> | ||
Previous research had shown, using propensity score matching (assuming that there are no unobserved differences between catheterized and non catheterized patients) that Swan-Ganz catheterization increases the probability that patients die within 180 days from admission to the intensive care unit. | Previous research had shown, using propensity score matching (assuming that there are no unobserved differences between catheterized and non catheterized patients) that Swan-Ganz catheterization increases the probability that patients die within 180 days from admission to the intensive care unit. | ||
<ref name="bha:sha:vyt12" | <ref name="bha:sha:vyt12"/> re-analyze the data using (and extending) bounds results obtained by <ref name="sha:vyt11"><span style="font-variant-caps:small-caps">Shaikh, A.M., <span style="font-variant-caps:normal">and</span> E.J. Vytlacil</span> (2011): “Partial identification in triangular systems of equations with binary dependent variables” ''Econometrica'', 79(3), 949--955.</ref>. | ||
These results are based on exclusion restrictions combined with a threshold crossing structure for both the treatment and the outcome variables in problems where <math>\cY=\cT=\{0,1\}</math>. | These results are based on exclusion restrictions combined with a threshold crossing structure for both the treatment and the outcome variables in problems where <math>\cY=\cT=\{0,1\}</math>. | ||
<ref name="bha:sha:vyt12" | |||
<ref name="bha:sha:vyt12"/> use as instrument for Swan-Ganz catheterization the day of the week that the patient was admitted to the intensive care unit. | |||
The reasoning is that patients are less likely to be catheterized on the weekend, but the admission day to the intensive care unit is plausibly uncorrelated with subsequent mortality. | The reasoning is that patients are less likely to be catheterized on the weekend, but the admission day to the intensive care unit is plausibly uncorrelated with subsequent mortality. | ||
Their results confirm that for some diagnoses, Swan-Ganz catheterization increases mortality at 30 days after catheterization and beyond. | Their results confirm that for some diagnoses, Swan-Ganz catheterization increases mortality at 30 days after catheterization and beyond. | ||
<ref name="man:pep18"></ref> use data from Maryland, Virginia and Illinois to learn about the impact of laws allowing individuals to carry concealed handguns (right-to-carry laws) on violent and property crimes. | <ref name="man:pep18"><span style="font-variant-caps:small-caps">Manski, C.F., <span style="font-variant-caps:normal">and</span> J.V. Pepper</span> (2018): “How Do Right-to-Carry Laws Affect Crime Rates? Coping with Ambiguity Using Bounded-Variation Assumptions” ''The Review of Economics and Statistics'', 100(2), 232--244.</ref> use data from Maryland, Virginia and Illinois to learn about the impact of laws allowing individuals to carry concealed handguns (right-to-carry laws) on violent and property crimes. | ||
Point identification of these treatment effects is possible under invariance assumptions that certain features of treatment response are constant across states and years. | Point identification of these treatment effects is possible under invariance assumptions that certain features of treatment response are constant across states and years. | ||
<ref name="man:pep18" | <ref name="man:pep18"/> propose the use of weaker but more credible restrictions according to which these features exhibit bounded variation -- the invariance case being the limit where the bound on variation equals zero. | ||
They carry out their analysis under different combinations of the bounded variation assumptions, and at each step they report the resulting bounds, thereby illuminating the role played by each assumption in shaping the inference. | They carry out their analysis under different combinations of the bounded variation assumptions, and at each step they report the resulting bounds, thereby illuminating the role played by each assumption in shaping the inference. | ||
<ref name="mou:hen:mea18"></ref> provide sharp bounds on the joint distribution of potential (binary) outcomes in a Roy model with sector specific unobserved heterogeneity and self selection based on potential outcomes. | |||
<ref name="mou:hen:mea18"><span style="font-variant-caps:small-caps">Mourifi\'{e}, I., M.Henry, <span style="font-variant-caps:normal">and</span> R.M\'{e}ango</span> (2018): “Sharp Bounds and Testability of a Roy Model of STEM Major Choices” available at [https://ssrn.com/abstract=2043117 https://ssrn.com/abstract=2043117].</ref> provide sharp bounds on the joint distribution of potential (binary) outcomes in a Roy model with sector specific unobserved heterogeneity and self selection based on potential outcomes. | |||
The key maintained assumption is that the researcher has access to data that includes a stochastically monotone instrumental variable. | The key maintained assumption is that the researcher has access to data that includes a stochastically monotone instrumental variable. | ||
This is a selection shifter that is restricted to affect potential outcomes monotonically. | This is a selection shifter that is restricted to affect potential outcomes monotonically. | ||
An example is parental education, which may not be independent from potential wages, but plausibly does not negatively affect future wages. | An example is parental education, which may not be independent from potential wages, but plausibly does not negatively affect future wages. | ||
Under this assumption, <ref name="mou:hen:mea18" | Under this assumption, <ref name="mou:hen:mea18"/> show that all observable implications of the model are embodied in the stochastic monotonicity of observed outcomes in the instrument, hence Roy selection behavior can be tested by checking this stochastic monotonicity. | ||
They apply the method to estimate a Roy model of college major choice in Canada and Germany, with special interest in the under-representation of women in STEM. | They apply the method to estimate a Roy model of college major choice in Canada and Germany, with special interest in the under-representation of women in STEM. | ||
<ref name="mog:san:tor18"></ref> provide a general method to obtain sharp bounds on a certain class of treatment effects parameters. | <ref name="mog:san:tor18"><span style="font-variant-caps:small-caps">Mogstad, M., A.Santos, <span style="font-variant-caps:normal">and</span> A.Torgovitsky</span> (2018): “Using Instrumental Variables for Inference About Policy Relevant Treatment Parameters” ''Econometrica'', 86(5), 1589--1619.</ref> provide a general method to obtain sharp bounds on a certain class of treatment effects parameters. | ||
This class is comprised of parameters that can be expressed as weighted averages of marginal treatment effects <ref name="hec:vyt99" | This class is comprised of parameters that can be expressed as weighted averages of marginal treatment effects <ref name="hec:vyt99"/><ref name="hec:vyt01"/><ref name="hec:vyt05"/>. | ||
<ref name="tor19pies"></ref> provides a general method, based on copulas, to obtain sharp bounds on treatment effect parameters in semiparametric binary models. | |||
A notable feature of both <ref name="mog:san:tor18" | <ref name="tor19pies"><span style="font-variant-caps:small-caps">Torgovitsky, A.</span> (2019b): “Partial identification by extending subdistributions” ''Quantitative Economics'', 10(1), 105--144.</ref> provides a general method, based on copulas, to obtain sharp bounds on treatment effect parameters in semiparametric binary models. | ||
<ref name="fre:hor14"></ref> provide partial identification results and inference methods for a linear functional <math>\ell(g)</math> when <math>g:\cX\mapsto\R</math> is such that <math>\ey=g(\ex)+\epsilon</math> and <math>\E(\ey|\ez)=0</math>. | A notable feature of both <ref name="mog:san:tor18"/> and <ref name="tor19pies"/> is that the bounds are obtained as solutions to convex (even linear) optimization problems, rendering them computationally attractive. | ||
<ref name="fre:hor14"><span style="font-variant-caps:small-caps">Freyberger, J., <span style="font-variant-caps:normal">and</span> J.L. Horowitz</span> (2015): “Identification and shape restrictions in nonparametric instrumental variables estimation” ''Journal of Econometrics'', 189(1), 41--53.</ref> provide partial identification results and inference methods for a linear functional <math>\ell(g)</math> when <math>g:\cX\mapsto\R</math> is such that <math>\ey=g(\ex)+\epsilon</math> and <math>\E(\ey|\ez)=0</math>. | |||
The instrumental variable <math>\ez</math> and regressor <math>\ex</math> have discrete distributions, and <math>\ez</math> has fewer points of support than <math>\ex</math>, so that <math>\ell(g)</math> can only be partially identified. | The instrumental variable <math>\ez</math> and regressor <math>\ex</math> have discrete distributions, and <math>\ez</math> has fewer points of support than <math>\ex</math>, so that <math>\ell(g)</math> can only be partially identified. | ||
They impose shape restrictions on <math>g</math> (e.g., monotonicity or convexity) to achieve interval identification of <math>\ell(g)</math>, and they show that the lower and upper points of the interval can be obtained by solving linear programming problems. | They impose shape restrictions on <math>g</math> (e.g., monotonicity or convexity) to achieve interval identification of <math>\ell(g)</math>, and they show that the lower and upper points of the interval can be obtained by solving linear programming problems. |
Latest revision as of 20:23, 19 June 2024
The literature reviewed in this chapter starts with the analysis of what can be learned about functionals of probability distributions that are well-defined in the absence of a model. The approach is nonparametric, and it is typically constructive, in the sense that it leads to “plug-in” formulae for the bounds on the functionals of interest.
Selectively Observed Data
As in [1], suppose that a researcher is interested in learning the probability that an individual who is homeless at a given date has a home six months later. Here the population of interest is the people who are homeless at the initial date, and the outcome of interest [math]\ey[/math] is an indicator of whether the individual has a home six months later (so that [math]\ey=1[/math]) or remains homeless (so that [math]\ey=0[/math]). A random sample of homeless individuals is interviewed at the initial date, so that individual background attributes [math]\ex[/math] are observed, but six months later only a subset of the individuals originally sampled can be located. In other words, attrition from the sample creates a ’'selection problem whereby [math]\ey[/math] is observed only for a subset of the population. Let [math]\ed[/math] be an indicator of whether the individual can be located (hence [math]\ed=1[/math]) or not (hence [math]\ed=0[/math]). The question is what can the researcher learn about [math]\E_\sQ(\ey|\ex=x)[/math], with [math]\sQ[/math] the distribution of [math](\ey,\ex)[/math]? [1] showed that [math]\E_\sQ(\ey|\ex=x)[/math] is not point identified in the absence of additional assumptions, but informative nonparametric bounds on this quantity can be obtained. In this section I review his approach, and discuss several important extensions of his original idea. Throughout the chapter, I formally state the structure of the problem under study as an “Identification Problem”, and then provide a solution, either in the form of a sharp identification region, or of an outer region. To set the stage, and at the cost of some repetition, I do the same here, slightly generalizing the question stated in the previous paragraph.
Let [math]\ey \in \mathcal{Y}\subset \R[/math] and [math]\ex \in \mathcal{X}\subset \R^d[/math] be, respectively, an outcome variable and a vector of covariates with support [math]\cY[/math] and [math]\cX[/math] respectively, with [math]\cY[/math] a compact set.
Let [math]\ed \in \{0,1\}[/math].
Suppose that the researcher observes a random sample of realizations of [math](\ex,\ed)[/math] and, in addition, observes the realization of [math]\ey[/math] when [math]\ed=1[/math].
Hence, the observed data is [math](\ey\ed,\ed,\ex)\sim \sP[/math].
Let [math]g:\cY\mapsto\R[/math] be a measurable function that attains its lower and upper bounds [math]g_0=\min_{y\in\cY}g(y)[/math] and [math]g_1=\max_{y\in\cY}g(y)[/math], and assume that [math]-\infty \lt g_0 \lt g_1 \lt \infty[/math].
Let [math]y_{j}\in\cY[/math] be such that [math]g(y_j)=g_j[/math], [math]j=0,1[/math].[Notes 1]
In the absence of additional information, what can the researcher learn about [math]\E_\sQ(g(\ey)|\ex=x)[/math], with [math]\sQ[/math] the distribution of [math](\ey,\ex)[/math]?
[1]’s analysis of this problem begins with a simple application of the law of total probability, that yields
Equation \eqref{eq:LTP_md} lends a simple but powerful anatomy of the selection problem. While [math]\sP(\ey|\ex=x,\ed=1)[/math] and [math]\sP(\ed|\ex=x)[/math] can be learned from the observable distribution [math]\sP(\ey\ed,\ed,\ex)[/math], under the maintained assumptions the sampling process reveals nothing about [math]\sR(\ey|\ex=x,\ed=0)[/math]. Hence, [math]\sQ(\ey|\ex=x)[/math] is not point identified. If one were to assume exogenous selection (or data missing at random conditional on [math]\ex[/math]), i.e., [math]\sR(\ey|\ex,\ed=0)=\sP(\ey|\ex,\ed=1)[/math], point identification would obtain. However, that assumption is non-refutable and it is well known that it may fail in applications [Notes 2]. Let [math]\cT[/math] denote the space of all probability measures with support in [math]\cY[/math]. The unknown functional vector is [math]\{\tau(x),\upsilon(x)\}\equiv \{\sQ(\ey|\ex=x),\sR(\ey|\ex=x,\ed=0)\}[/math]. What the researcher can learn, in the absence of additional restrictions on [math]\sR(\ey|\ex=x,\ed=0)[/math], is the region of observationally equivalent distributions for [math]\ey|\ex=x[/math], and the associated set of expectations taken with respect to these distributions.
Under the assumptions in Identification Problem,
Due to the discussion following equation \eqref{eq:LTP_md}, the collection of observationally equivalent distribution functions for [math]\ey|\ex=x[/math] is
These are the worst case bounds, so called because assumptions free and therefore representing the widest possible range of values for the parameter of interest that are consistent with the observed data. A simple “plug-in” estimator for [math]\idr{\E_\sQ(g(\ey)|\ex=x)}[/math] replaces all unknown quantities in \eqref{eq:bounds:mean:md} with consistent estimators, obtained, e.g., by kernel or sieve regression. I return to consistent estimation of partially identified parameters in Section. Here I emphasize that identification problems are fundamentally distinct from finite sample inference problems. The latter are typically reduced as sample size increase (because, e.g., the variance of the estimator becomes smaller). The former do not improve, unless a different and better type of data is collected, e.g. with a smaller prevalence of missing data (see [2](for a discussion)).
[3](Section 1.3) shows that the proof of Theorem SIR- can be extended to obtain the smallest and largest points in the sharp identification region of any parameter that respects stochastic dominance.[Notes 3] This is especially useful to bound the quantiles of [math]\ey|\ex=x[/math]. For any given [math]\alpha \in (0,1)[/math], let [math]\sq_{\sP}^{g(\ey)}(\alpha,1,x)\equiv \left\{\min t:\sP(g(\ey)\le t|\ed=1,\ex=x)\ge \alpha\right\}[/math]. Then the smallest and largest admissible values for the [math]\alpha[/math]-quantile of [math]g(\ey)|\ex=x[/math] are, respectively,
The lower bound on [math]\E_\sQ(g(\ey)|\ex=x)[/math] is informative only if [math]g_0 \gt -\infty[/math], and the upper bound is informative only if [math]g_1 \lt \infty[/math]. By comparison, for any value of [math]\alpha[/math], [math]r(\alpha,x)[/math] and [math]s(\alpha,x)[/math] are generically informative if, respectively, [math]\sP(\ed=1|\ex=x) \gt 1-\alpha[/math] and [math]\sP(\ed=1|\ex=x) \ge \alpha[/math], regardless of the range of [math]g[/math]. [4] further extends partial identification analysis to the study of spread parameters in the presence of missing data (as well as interval data, data combinations, and other applications). These parameters include ones that respect second order stochastic dominance, such as the variance, the Gini coefficient, and other inequality measures, as well as other measures of dispersion which do not respect second order stochastic dominance, such as interquartile range and ratio.[Notes 4] [4] shows that the sharp identification region for these parameters can be obtained by fixing the mean or quantile of the variable of interest at a specific value within its sharp identification region, and deriving a distribution consistent with this value which is ``compressed" with respect to the ones which bound the cumulative distribution function (CDF) of the variable of interest, and one which is ``dispersed" with respect to them. Heuristically, the compressed distribution minimizes spread, while the dispersed one maximizes it (the sense in which this optimization occurs is formally defined in the paper). The intuition for this is that a compressed CDF is first below and then above any non-compressed one; a dispersed CDF is first above and then below any non-dispersed one. Second-stage optimization over the possible values of the mean or the quantile delivers unconstrained bounds. The main results of the paper are sharp identification regions for the expectation and variance, for the median and interquartile ratio, and for many other combinations of parameters.
Key Insight (Identification is not a binary event): Identification Problem is mathematically simple, but it puts forward a new approach to empirical research. The traditional approach aims at finding a sufficient (possibly minimal) set of assumptions guaranteeing point identification of parameters, viewing identification as an “all or nothing” notion, where either the functional of interest can be learned exactly or nothing of value can be learned. The partial identification approach pioneered by [1] points out that much can be learned from combination of data and assumptions that restrict the functionals of interest to a set of observationally equivalent values, even if this set is not a singleton. Along the way, [1] points out that in Identification Problem the observed outcome is the singleton [math]\ey[/math] when [math]\ed=1[/math], and the set [math]\cY[/math] when [math]\ed=0[/math]. This is a random closed set, see Definition. I return to this connection in Section Interval Data.
Despite how transparent the framework in Identification Problem is, important subtleties arise even in this seemingly simple context. For a given [math]t\in\R[/math], consider the function [math]g(\ey)=\one(\ey\le t)[/math], with [math]\one(A)[/math] the indicator function taking the value one if the logical condition in parentheses holds and zero otherwise. Then equation \eqref{eq:bounds:mean:md} yields ’'pointwise-sharp bounds on the CDF of [math]\ey[/math] at any fixed [math]t\in\R[/math]:
Yet, the collection of CDFs that belong to the band defined by \eqref{eq:pointwise_bounds_F_md} is not the sharp identification region for the CDF of [math]\ey|\ex=x[/math]. Rather, it constitutes an outer region, as originally pointed out by [5](p. 149 and note 2).
Let [math]\cC[/math] denote the collection of cumulative distribution functions on [math]\cY[/math]. Then, under the assumptions in Identification Problem,
is an outer region for the CDF of [math]\ey|\ex=x[/math].
Show ProofAny admissible CDF for [math]\ey|\ex=x[/math] belongs to the family of functions in equation \eqref{eq:outer_cdf_md}. However, the bound in equation \eqref{eq:outer_cdf_md} does not impose the restriction that for any [math]t_0\le t_1[/math],
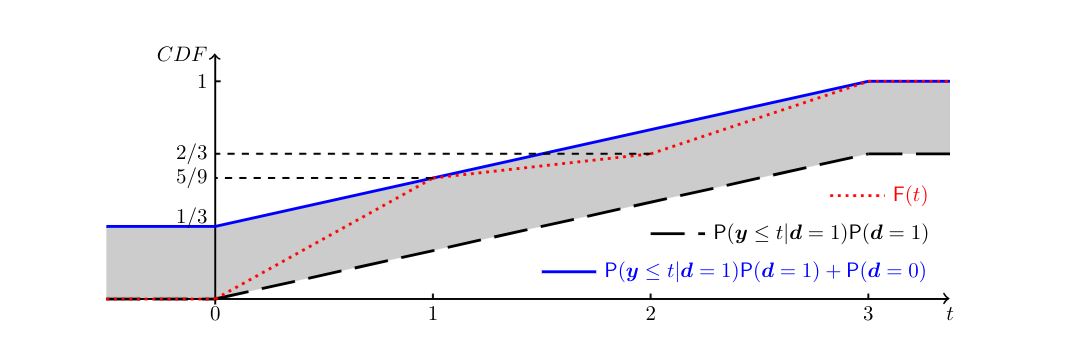
Example
Omit [math]\ex[/math] for simplicity, let [math]\sP(\ed=1)=\frac{2}{3}[/math], and let
The bounding functions and associated tube from the inequalities in \eqref{eq:pointwise_bounds_F_md} are depicted in Figure. Consider the cumulative distribution function
For each [math]t\in\R[/math], [math]\sF(t)[/math] lies in the tube defined by equation \eqref{eq:pointwise_bounds_F_md}. However, it cannot be the CDF of [math]\ey[/math], because [math]\sF(2)-\sF(1)=\frac{1}{9} \lt \sP(1\le\ey\le 2|\ed=1)\sP(\ed=1)[/math], directly contradicting equation \eqref{eq:CDF_md_Kinterval}.
How can one characterize the sharp identification region for the CDF of [math]\ey|\ex=x[/math] under the assumptions in Identification Problem?
In general, there is not a single answer to this question: different methodologies can be used.
Here I use results in [3](Corollary 1.3.1) and [6](Theorem 2.25), which yield an alternative characterization of [math]\idr{\sQ(\ey|\ex=x)}[/math] that translates directly into a characterization of [math]\idr{\sF(\ey|\ex=x)}[/math].[Notes 5]
Given [math]\tau\in\cT[/math], let [math]\tau_K(x)[/math] denote the probability that distribution [math]\tau[/math] assigns to set [math]K[/math] conditional on [math]\ex=x[/math], with [math]\tau_y(x)\equiv\tau_{\{y\}}(x)[/math].
Under the assumptions in Identification Problem,
The characterization in \eqref{eq:sharp_id_P_md_Manski} follows from equation \eqref{eq:Tau_md}, observing that if [math]\tau(x)\in\idr{\sQ(\ey|\ex=x)}[/math] as defined in equation \eqref{eq:Tau_md}, then there exists a distribution [math]\upsilon(x)\in\cT[/math] such that [math]\tau(x) = \sP(\ey|\ex=x,\ed=1)\sP(\ed=1|\ex=x)+\upsilon(x)\sP(\ed=0|\ex=x)[/math]. Hence, by construction [math]\tau_K(x) \ge \sP(\ey\in K|\ex=x,\ed=1)\sP(\ed=1|\ex=x)[/math], [math]\forall K\subset \cY[/math]. Conversely, if one has [math]\tau_K(x) \ge \sP(\ey\in K|\ex=x,\ed=1)\sP(\ed=1|\ex=x)[/math], [math]\forall K\subset \cY[/math], one can define [math]\upsilon(x)=\frac{\tau(x) - \sP(\ey|\ex=x,\ed=1)\sP(\ed=1|\ex=x)}{\sP(\ed=0|\ex=x)}[/math]. The resulting [math]\upsilon(x)[/math] is a probability measure, and hence [math]\tau(x)\in\idr{\sQ(\ey|\ex=x)}[/math] as defined in equation \eqref{eq:Tau_md}. When [math]\cY[/math] is countable, if [math]\tau_y(x) \ge \sP(\ey=y|\ex=x,\ed=1)\sP(\ed=1|\ex=x)[/math] it follows that for any [math]K\subset\cY[/math],
This section provides sharp identification regions and outer regions for a variety of functionals of interest. The computational complexity of these characterizations varies widely. Sharp bounds on parameters that respect stochastic dominance only require computing the parameters with respect to two probability distributions. An outer region on the CDF can be obtained by evaluating all tail probabilities of a certain distribution. A sharp identification region on the CDF requires evaluating the probability that a certain distribution assigns to all intervals. I return to computational challenges in partial identification in Section.
Treatment Effects with and without Instrumental Variables
The discussion of partial identification of probability distributions of selectively observed data naturally leads to the question of its implications for program evaluation. The literature on program evaluation is vast. The purpose of this section is exclusively to show how the ideas presented in Section Selectively Observed Data can be applied to learn features of treatment effects of interest, when no assumptions are imposed on treatment selection and outcomes. I also provide examples of assumptions that can be used to tighten the bounds. To keep this chapter to a manageable length, I discuss only partial identification of the average response to a treatment and of the average treatment effect (ATE). There are many different parameters that received much interest in the literature. Examples include the local average treatment effect of [7] and the marginal treatment effect of [8][9][10]. For thorough discussions of the literature on program evaluation, I refer to the textbook treatments in [11][3][12] and [13], to the Handbook chapters by [14][15] and [16], and to the review articles by [17] and [18].
Using standard notation (e.g., [19]), let [math]\ey:\T \mapsto \cY[/math] be an individual-specific response function, with [math]\T=\{0,1,\dots,T\}[/math] a finite set of mutually exclusive and exhaustive treatments, and let [math]\es[/math] denote the individual's received treatment (taking its realizations in [math]\T[/math]).[Notes 6] The researcher observes data [math](\ey,\es,\ex)\sim\sP[/math], with [math]\ey\equiv\ey(\es)[/math] the outcome corresponding to the received treatment [math]\es[/math], and [math]\ex[/math] a vector of covariates. The outcome [math]\ey(t)[/math] for [math]\es\neq t[/math] is counterfactual, and hence can be conceptualized as missing. Therefore, we are in the framework of Identification Problem and all the results from Section Selectively Observed Data apply in this context too, subject to adjustments in notation.[Notes 7] For example, using Theorem SIR-,
where [math]y_0\equiv\inf_{y\in\cY}y[/math], [math]y_1\equiv\sup_{y\in\cY}y[/math]. If [math]y_0 \lt \infty[/math] and/or [math]y_1 \lt \infty[/math], these worst case bounds are informative. When both are infinite, the data is uninformative in the absence of additional restrictions. If the researcher is interested in an Average Treatment Effect (ATE), e.g.
with [math]t_0,t_1\in\T[/math], sharp worst case bounds on this quantity can be obtained as follows. First, observe that the empirical evidence reveals [math]\E_\sP(\ey|\ex=x,\es=t_j)[/math] and [math]\sP(\es|\ex=x)[/math], but is uninformative about [math]\E_\sQ(\ey(t_j)|\ex=x,\es\neq t_j)[/math], [math]j=0,1[/math]. Each of the latter quantities (the expectations of [math]\ey(t_0)[/math] and [math]\ey(t_1)[/math] conditional on different realizations of [math]\es[/math] and [math]\ex=x[/math]) can take any value in [math][y_0,y_1][/math]. Hence, the sharp lower bound on the ATE is obtained by subtracting the upper bound on [math]\E_\sQ(\ey(t_0)|\ex=x)[/math] from the lower bound on [math]\E_\sQ(\ey(t_1)|\ex=x)[/math]. The sharp upper bound on the ATE is obtained by subtracting the lower bound on [math]\E_\sQ(\ey(t_0)|\ex=x)[/math] from the upper bound on [math]\E_\sQ(\ey(t_1)|\ex=x)[/math]. The resulting bounds have width equal to [math](y_1-y_0)[2-\sP(\es=t_1|\ex=x)-\sP(\es=t_0|\ex=x)]\in[(y_1-y_0),2(y_1-y_0)][/math], and hence are informative only if both [math]y_0 \gt -\infty[/math] and [math]y_1 \lt \infty[/math]. As the largest logically possible value for the ATE (in the absence of information from data) cannot be larger than [math](y_1-y_0)[/math], and the smallest cannot be smaller than [math]-(y_1-y_0)[/math], the sharp bounds on the ATE always cover zero.
Key Insight: How should one think about the finding on the size of the worst case bounds on the ATE? On the one hand, if both [math]y_0 \lt \infty[/math] and [math]y_1 \lt \infty[/math] the bounds are informative, because they are a strict subset of the ATE's possible realizations. On the other hand, they reveal that the data alone are silent on the sign of the ATE. This means that assumptions play a crucial role in delivering stronger conclusions about this policy relevant parameter. The partial identification approach to empirical research recommends that as assumptions are added to the analysis, one systematically reports how each contributes to shrinking the bounds, making transparent their role in shaping inference.
What assumptions may researchers bring to bear to learn more about treatment effects of interest? The literature has provided a wide array of well motivated and useful restrictions. Here I consider two examples. The first one entails shape restrictions on the treatment response function, leaving selection unrestricted. [20] obtains bounds on treatment effects under the assumption that the response functions are monotone, semi-monotone, or concave-monotone. These restrictions are motivated by economic theory, where it is commonly presumed, e.g., that demand functions are downward sloping and supply functions are upward sloping. Let the set [math]\T[/math] be ordered in terms of degree of intensity. Then [20]'s monotone treatment response assumption requires that
Under this assumption, one has a sharp characterization of what can be learned about [math]\ey(t)[/math]:
Hence, the sharp bounds on [math]\E_\sQ(\ey(t)|\ex=x)[/math] are [20](Proposition M1)
This finding highlights some important facts. Under the monotone treatment response assumption, the bounds on [math]\E_\sQ(\ey(t)|\ex=x)[/math] are obtained using information from all [math](\ey,\es)[/math] pairs (given [math]\ex=x[/math]), while the bounds in \eqref{eq:WCB:treat} only use the information provided by [math](\ey,\es)[/math] pairs for which [math]\es=t[/math] (given [math]\ex=x[/math]). As a consequence, the bounds in \eqref{eq:MTR:treat} are informative even if [math]\sP(\es= t|\ex=x)=0[/math], whereas the worst case bounds are not. Concerning the ATE with [math]t_1 \gt t_0[/math], under monotone treatment response its lower bound is zero, and its upper bound is obtained by subtracting the lower bound on [math]\E_\sQ(\ey(t_0)|\ex=x)[/math] from the upper bound on [math]\E_\sQ(\ey(t_1)|\ex=x)[/math], where both bounds are obtained as in \eqref{eq:MTR:treat} [20](Proposition M2). The second example of assumptions used to tighten worst case bounds is that of exclusion restrictions, as in, e.g., [21]. Suppose the researcher observes a random variable [math]\ez[/math], taking its realizations in [math]\cZ[/math], such that[Notes 8]
This assumption is treatment-specific, and requires that the treatment response to [math]t[/math] is mean independent with [math]\ez[/math]. It is easy to show that under the assumption in \eqref{eq:ass:MI}, the bounds on [math]\E_\sQ(\ey(t)|\ex=x)[/math] become
These are called intersection bounds because they are obtained as follows. Given [math]\ex[/math] and [math]\ez[/math], one uses \eqref{eq:WCB:treat} to obtain sharp bounds on [math]\E_\sQ(\ey(t)|\ez=z,\ex=x)[/math]. Due to the mean independence assumption in \eqref{eq:ass:MI}, [math]\E_\sQ(\ey(t)|\ex=x)[/math] must belong to each of these bounds [math]\ez[/math]-a.s., hence to their intersection. The expression in \eqref{eq:intersection:bounds} follows. If the instrument affects the probability of being selected into treatment, or the average outcome for the subpopulation receiving treatment [math]t[/math], the bounds on [math]\E_\sQ(\ey(t)|\ex=x)[/math] shrink. If the bounds are empty, the mean independence assumption can be refuted (see Section for a discussion of misspecification in partial identification). [22][23] generalize the notion of instrumental variable to monotone instrumental variable, and show how these can be used to obtain tighter bounds on treatment effect parameters.[Notes 9] They also show how shape restrictions and exclusion restrictions can jointly further tighten the bounds. [24] generalizes these findings to the case where treatment response may have social interactions -- that is, each individual's outcome depends on the treatment received by all other individuals.
Interval Data
Identification Problem, as well as the treatment evaluation problem in Section Treatment Effects with and without Instrumental Variables, is an instance of the more general question of what can be learned about (functionals of) probability distributions of interest, in the presence of interval valued outcome and/or covariate data. Such data have become commonplace in Economics. For example, since the early 1990s the Health and Retirement Study collects income data from survey respondents in the form of brackets, with degenerate (singleton) intervals for individuals who opt to fully reveal their income (see, e.g., [25]). Due to concerns for privacy, public use tax data are recorded as the number of tax payers which belong to each of a finite number of cells (see, e.g., [26]). The Occupational Employment Statistics (OES) program at the Bureau of Labor Statistics [27] collects wage data from employers as intervals, and uses these data to construct estimates for wage and salary workers in more than 800 detailed occupations. [28] and [29] document the extensive prevalence of rounding in survey responses to probabilistic expectation questions, and propose to use a person's response pattern across different questions to infer his rounding practice, the result being interpretation of reported numerical values as interval data. Other instances abound. Here I focus first on the case of interval outcome data.
Assume that in addition to being compact, either [math]\cY[/math] is countable or [math]\cY=[y_0,y_1][/math], with [math]y_0=\min_{y\in\cY}y[/math] and [math]y_1=\max_{y\in\cY}y[/math]. Let [math](\yL,\yU,\ex)\sim\sP[/math] be observable random variables and [math]\ey[/math] be an unobservable random variable whose distribution (or features thereof) is of interest, with [math]\yL,\yU,\ey\in\cY[/math]. Suppose that [math](\yL,\yU,\ey)[/math] are such that [math]\sR(\yL\le\ey\le\yU)=1[/math].[Notes 10] In the absence of additional information, what can the researcher learn about features of [math]\sQ(\ey|\ex=x)[/math], the conditional distribution of [math]\ey[/math] given [math]\ex=x[/math]?
It is immediate to obtain the sharp identification region
As in the previous section, it is also easy to obtain sharp bounds on parameters that respect stochastic dominance, and pointwise-sharp bounds on the CDF of [math]\ey[/math] at any fixed [math]t\in\R[/math]:
In this case too, however, as in Theorem OR-, the tube of CDFs satisfying equation \eqref{eq:pointwise_bounds_F} for all [math]t\in\R[/math] is an outer region for the CDF of [math]\ey|\ex=x[/math], rather than its sharp identification region. Indeed, also in this context it is easy to construct examples similar to Example. How can one characterize the sharp identification region for the probability distribution of [math]\ey|\ex[/math] when one observes [math](\yL,\yU,\ex)[/math] and assumes [math]\sR(\yL\le\ey\le\yU)=1[/math]? Again, there is not a single answer to this question. Depending on the specific problem at hand, e.g., the specifics of the interval data and whether [math]\ey[/math] is assumed discrete or continuous, different methods can be applied. I use random set theory to provide a characterization of [math]\idr{\sQ(\ey|\ex=x)}[/math]. Let
Then [math]\eY[/math] is a random closed set according to Definition.[Notes 11] The requirement [math]\sR(\yL\le\ey\le\yU)=1[/math] can be equivalently expressed as
Equation \eqref{eq:y_in_Y}, together with knowledge of [math]\sP[/math], exhausts all the information in the data and maintained assumptions. In order to harness such information to characterize the set of observationally equivalent probability distributions for [math]\ey[/math], one can leverage a result due to [30] (and [31]), reported in Theorem in Appendix, which allows one to translate \eqref{eq:y_in_Y} into a collection of conditional moment inequalities. Specifically, let [math]\cT[/math] denote the space of all probability measures with support in [math]\cY[/math].
Given [math]\tau\in\cT[/math], let [math]\tau_K(x)[/math] denote the probability that distribution [math]\tau[/math] assigns to set [math]K[/math] conditional on [math]\ex=x[/math].
Under the assumptions in Identification Problem, the sharp identification region for [math]\sQ(\ey|\ex=x)[/math] is
Compare equation \eqref{eq:sharp_id_P_interval_1} with equation \eqref{eq:sharp_id_P_md_Manski}. Under the set-up of Identification Problem, when [math]\ed=1[/math] we have [math]\eY=\{\ey\}[/math] and when [math]\ed=0[/math] we have [math]\eY=\cY[/math]. Hence, for any [math]K \subsetneq \cY[/math], [math]\sP(\eY \subset K|\ex=x)=\sP(\ey\in K|\ex=x,\ed=1)\sP(\ed=1)[/math].[Notes 12] It follows that the characterizations in \eqref{eq:sharp_id_P_interval_1} and \eqref{eq:sharp_id_P_md_Manski} are equivalent. If [math]\cY[/math] is countable, it is easy to show that \eqref{eq:sharp_id_P_interval_1} simplifies to \eqref{eq:sharp_id_P_md_Manski} (see, e.g., [32](Proposition 2.2)).
Key Insight (Random set theory and partial identification):The mathematical framework for the analysis of random closed sets embodied in random set theory is naturally suited to conduct identification analysis and statistical inference in partially identified models. This is because, as argued by [33] and [34][32], lack of point identification can often be traced back to a collection of random variables that are consistent with the available data and maintained assumptions. In turn, this collection of random variables is equal to the family of selections of a properly specified random closed set, so that random set theory applies. The interval data case is a simple example that illustrates this point. More examples are given throughout this chapter. As mentioned in the Introduction, the exercise of defining the random closed set that is relevant for the problem under consideration is routinely carried out in partial identification analysis, even when random set theory is not applied. For example, in the case of treatment effect analysis with monotone response function, [20] derived the set in the right-hand-side of \eqref{eq:RCS:MTR}, which satisfies Definition def:rcs.
An attractive feature of the characterization in \eqref{eq:sharp_id_P_interval_1} is that it holds regardless of the specific assumptions on [math]\yL,\,\yU[/math], and [math]\cY[/math]. Later sections in this chapter illustrate how Theorem delivers the sharp identification region in other more complex instances of partial identification of probability distributions, as well as in structural models. In Chapter XXX in this Volume, [35] apply Theorem to obtain sharp identification regions for functionals of interest in the important class of generalized instrumental variable models. To avoid repetitions, I do not systematically discuss that class of models in this chapter. When addressing questions about features of [math]\sQ(\ey|\ex=x)[/math] in the presence of interval outcome data, an alternative approach (e.g. [36][37]) looks at all (random) mixtures of [math]\yL,\yU[/math]. The approach is based on a random variable [math]\eu[/math] (a selection mechanism that picks an element of [math]\eY[/math]) with values in [math][0,1][/math], whose distribution conditional on [math]\yL,\yU[/math] is left completely unspecified. Using this random variable, one defines
The sharp identification region in Theorem SIR- can be characterized as the collection of conditional distributions of all possible random variables [math]\ey_\eu[/math] as defined in \eqref{eq:y_s}, given [math]\ex=x[/math]. This is because each [math]\ey_\eu[/math] is a (stochastic) convex combination of [math]\yL,\yU[/math], hence each of these random variables satisfies [math]\sR(\yL\le\ey_\eu\le\yU)=1[/math]. While such characterization is sharp, it can be of difficult implementation in practice, because it requires working with all possible random variables [math]\ey_\eu[/math] built using all possible random variables [math]\eu[/math] with support in [math][0,1][/math]. Theorem allows one to bypass the use of [math]\eu[/math], and obtain directly a characterization of the sharp identification region for [math]\sQ(\ey|\ex=x)[/math] based on conditional moment inequalities.[Notes 13] [38][39] study nonparametric conditional prediction problems with missing outcome and/or missing covariate data. Their analysis shows that this problem is considerably more pernicious than the case where only outcome data are missing. For the case of interval covariate data, [40] provide a set of sufficient conditions under which simple and elegant sharp bounds on functionals of [math]\sQ(\ey|\ex)[/math] can be obtained, even in this substantially harder identification problem. Their assumptions are listed in Identification Problem, and their result (with proof) in Theorem SIR-.
Let [math](\ey,\xL,\xU)\sim\sP[/math] be observable random variables in [math]\R\times\R\times\R[/math] and [math]\ex\in\R[/math] be an unobservable random variable.
Suppose that [math]\sR[/math], the joint distribution of [math](\ey,\ex,\xL,\xU)[/math], is such that: (I) [math]\sR(\xL\le\ex\le\xU)=1[/math]; (M) [math]\E_\sQ(\ey|\ex=x)[/math] is weakly increasing in [math]x[/math]; and (MI) [math]\E_{\sR}(\ey|\ex,\xL,\xU)=\E_\sQ(\ey|\ex)[/math].
In the absence of additional information, what can the researcher learn about [math]\E_\sQ(\ey|\ex=x)[/math] for given [math]x\in\cX[/math]?
Compared to the earlier discussion for the interval outcome case, here there are two additional assumptions. The monotonicity condition (M) is a simple shape restrictions, which however requires some prior knowledge about the joint distribution of [math](\ey,\ex)[/math]. The mean independence restriction (MI) requires that if [math]\ex[/math] were observed, knowledge of [math](\xL,\xU)[/math] would not affect the conditional expectation of [math]\ey|\ex[/math]. The assumption is not innocuous, as pointed out by the authors. For example, it may fail if censoring is endogenous.[Notes 14]
Under the assumptions of Identification Problem, the sharp identification region for [math]\E_\sQ(\ey|\ex=x)[/math] for given [math]x\in\cX[/math] is
The law of iterated expectations and the independence assumption yield [math]\E_\sP(\ey|\xL,\xU)=\int \E_\sQ(\ey|\ex)d\sR(\ex|\xL,\xU)[/math]. For all [math]\underline{x}\le \bar{x}[/math], the monotonicity assumption and the fact that [math]\ex\in[\xL,\xU][/math]-a.s. yield [math]\E_\sQ(\ey|\ex=\underline{x})\le \int \E_\sQ(\ey|\ex)d\sR(\ex|\xL=\underline{x},\xU=\bar{x}) \le \E_\sQ(\ey|\ex=\bar{x})[/math]. Putting this together with the previous result, [math]\E_\sQ(\ey|\ex=\underline{x})\le \E_\sP(\ey|\xL=\underline{x},\xU=\bar{x}) \le \E_\sQ(\ey|\ex=\bar{x})[/math]. Then (using again the monotonicity assumption) for any [math]x\ge \bar{x}[/math], [math]\E_{\sP}(\ey|\xL=\underline{x},\xU=\bar{x}) \le \E_\sQ(\ey|\ex=x)[/math] so that the lower bound holds. The bound is weakly increasing as a function of [math]x[/math], so that the monotonicity assumption on [math]\E_\sQ(\ey|\ex=x)[/math] holds and the bound is sharp. The argument for the upper bound can be concluded similarly.
Learning about functionals of [math]\sQ(\ey|\ex=x)[/math] naturally implies learning about predictors of [math]\ey|\ex=x[/math]. For example, [math]\idr{\E_\sQ(\ey|\ex=x)}[/math] yields the collection of values for the best predictor under square loss; [math]\idr{\M_\sQ(\ey|\ex=x)}[/math], with [math]\M_\sQ[/math] the median with respect to distribution [math]\sQ[/math], yields the collection of values for the best predictor under absolute loss. And so on. A related but distinct problem is that of parametric conditional prediction. Often researchers specify not only a loss function for the prediction problem, but also a parametric family of predictor functions, and wish to learn the member of this family that minimizes expected loss. To avoid confusion, let me clarify that here I am not referring to a parametric assumption on the best predictor, e.g., that [math]\E_\sQ(\ey|\ex)[/math] is a linear function of [math]\ex[/math]. I return to such assumptions at the end of this section. For now, in the example of linearity and square loss, I am referring to best linear prediction, i.e., best linear approximation to [math]\E_\sQ(\ey|\ex)[/math]. [3](pp. 56-58) discusses what can be learned about the best linear predictor of [math]\ey[/math] conditional on [math]\ex[/math], when only interval data on [math](\ey,\ex)[/math] is available. I treat first the case of interval outcome and perfectly observed covariates.
Maintain the same assumptions as in Identification Problem. Let [math](\yL,\yU,\ex)\sim\sP[/math] be observable random variables and [math]\ey[/math] be an unobservable random variable, with [math]\sR(\yL\le\ey\le\yU)=1[/math]. In the absence of additional information, what can the researcher learn about the best linear predictor of [math]\ey[/math] given [math]\ex=x[/math]?
For simplicity suppose that [math]\ex[/math] is a scalar, and let [math]\theta=[\theta_0~\theta_1]^\top\in\Theta\subset\R^2[/math] denote the parameter vector of the best linear predictor of [math]\ey|\ex[/math]. Assume that [math]Var(\ex) \gt 0[/math]. Combining the definition of best linear predictor with a characterization of the sharp identification region for the joint distribution of [math](\ey,\ex)[/math], we have that
where, using an argument similar to the one in Theorem SIR-,
[33](Proposition 4.1) show that \eqref{eq:manski_blp} can be re-written in an intuitive way that generalizes the well-known formula for the best linear predictor that arises when [math]\ey[/math] is perfectly observed. Define the random segment [math]\eG[/math] and the matrix [math]\Sigma_\sP[/math] as
where [math]\Sel(\eY)[/math] is the set of all measurable selections from [math]\eY[/math], see Definition. Then,
Under the assumptions of Identification Problem, the sharp identification region for the parameters of the best linear predictor of [math]\ey|\ex[/math] is
In either representation \eqref{eq:manski_blp} or \eqref{eq:ThetaI_BLP}, [math]\idr{\theta}[/math] is the collection of best linear predictors for each selection of [math]\eY[/math].[Notes 15] Why should one bother with the representation in \eqref{eq:ThetaI_BLP}? The reason is that [math]\idr{\theta}[/math] is a convex set, as it can be evinced from representation \eqref{eq:ThetaI_BLP}: [math]\eG[/math] has almost surely convex realizations that are segments and the Aumann expectation of a convex set is convex.[Notes 16] Hence, it can be equivalently represented through its support function [math]h_{\idr{\theta}}[/math], see Definition and equation eq:rocka. In particular, in this example,
where [math]f(\ex,u)\equiv [1~\ex]\Sigma_\sP^{-1}u[/math].[Notes 17] The characterization in \eqref{eq:supfun:BLP} results from Theorem, which yields [math]h_{\idr{\theta}}(u)=h_{\Sigma_\sP^{-1} \E_\sP\eG}(u)=\E_\sP h_{\Sigma_\sP^{-1} \eG}(u)[/math], and the fact that [math]\E_\sP h_{\Sigma_\sP^{-1} \eG}(u)[/math] equals the expression in \eqref{eq:supfun:BLP}. As I discuss in Section below, because the support function fully characterizes the boundary of [math]\idr{\theta}[/math], \eqref{eq:supfun:BLP} allows for a simple sample analog estimator, and for inference procedures with desirable properties. It also immediately yields sharp bounds on linear combinations of [math]\theta[/math] by judicious choice of [math]u[/math].[Notes 18] [41] and [42] provide the same characterization as in \eqref{eq:supfun:BLP} using, respectively, direct optimization and the Frisch-Waugh-Lovell theorem.
A natural generalization of Identification Problem allows for both outcome and covariate data to be interval valued.
Maintain the same assumptions as in Identification Problem, but with [math]\ex\in\cX\subset\R[/math] unobservable.
Let the researcher observe [math](\yL,\yU,\xL,\xU)[/math] such that [math]\sR(\yL \leq \ey \leq \yU , \xL \leq \ex \leq \xU)=1[/math].
Let [math]\eX\equiv [\xL,\xU][/math] and let [math]\cX[/math] be bounded.
In the absence of additional information, what can the researcher learn about the best linear predictor of [math]\ey[/math] given [math]\ex=x[/math]?
Abstractly, [math]\idr{\theta}[/math] is as given in \eqref{eq:manski_blp}, with
replacing \eqref{eq:Qyx} by an application of Theorem. While this characterization is sharp, it is cumbersome to apply in practice, see [43]. On the other hand, when both [math]\ey[/math] and [math]\ex[/math] are perfectly observed, the best linear predictor is simply equal to the parameter vector that yields a mean zero prediction error that is uncorrelated with [math]\ex[/math]. How can this basic observation help in the case of interval data? The idea is that one can use the same insight applied to the set-valued data, and obtain [math]\idr{\theta}[/math] as the collection of [math]\theta[/math]'s for which there exists a selection [math](\tilde{\ey},\tilde{\ex}) \in \Sel(\eY \times \eX)[/math], and associated prediction error [math]\eps_\theta=\tilde{\ey}-\theta_0-\theta_1 \tilde{\ex}[/math], satisfying [math]\E_\sP \eps_\theta=0[/math] and [math]\E_\sP (\eps_\theta \tilde{\ex})=0[/math] (as shown by [34]).[Notes 19] To obtain the formal result, define the [math]\theta[/math]-dependent set[Notes 20]
Under the assumptions of Identification Problem, the sharp identification region for the parameters of the best linear predictor of [math]\ey|\ex[/math] is
By Theorem, [math](\tilde\ey,\tilde\ex)\in(\eY\times\eX)[/math] (up to an ordered coupling as discussed in Appendix), if and only if the distribution of [math](\tilde\ey,\tilde\ex)[/math] belongs to [math]\idr{\sQ(\ey,\ex)}[/math]. For given [math]\theta[/math], one can find [math](\tilde\ey,\tilde\ex)\in(\eY\times\eX)[/math] such that [math]\E_\sP \eps_\theta=0[/math] and [math]\E_\sP (\eps_\theta \tilde{\ex})=0[/math] with [math]\eps_\theta\in\Eps_\theta[/math] if and only if the zero vector belongs to [math]\E_\sP \Eps_\theta[/math]. By Theorem, [math]\E_\sP \Eps_\theta[/math] is a convex set and by eq:dom_Aumann, [math]\mathbf{0} \in \E_\sP \Eps_\theta[/math] if and only if [math]0 \leq h_{\E_\sP \Eps_\theta}(u) \,\forall \, u \in \Ball[/math]. The final characterization follows from eq:supf.
The support function [math]h_{\Eps_\theta}(u)[/math] is an easy to calculate convex sublinear function of [math]u[/math], regardless of whether the variables involved are continuous or discrete. The optimization problem in (eq:ThetaI:BLP), determining whether [math]\theta \in \idr{\theta}[/math], is a convex program, hence easy to solve. See for example the CVX software by [44]. It should be noted, however, that the set [math]\idr{\theta}[/math] itself is not necessarily convex. Hence, tracing out its boundary is non-trivial. I discuss computational challenges in partial identification in Section. I conclude this section by discussing parametric regression. [40] study identification of parametric regression models under the assumptions in Identification Problem; Theorem SIR- below reports the result. The proof is omitted because it follows immediately from the proof of Theorem SIR-.
Let [math](\ey,\xL,\xU,\ew)\sim\sP[/math] be observable random variables in [math]\R\times\R\times\R\times\R^d[/math], [math]d \lt \infty[/math], and let [math]\ex\in\R[/math] be an unobservable random variable. Assume that the joint distribution [math]\sR[/math] of [math](\ey,\ex,\xL,\xU)[/math] is such that [math]\sR(\xL\le\ex\le\xU)=1[/math] and [math]\E_{\sR}(\ey|\ew,\ex,\xL,\xU)=\E_\sQ(\ey|\ew,\ex)[/math]. Suppose that [math]\E_\sQ(\ey|\ew,\ex)=f(\ew,\ex;\theta)[/math], with [math]f:\R^d\times\R\times\Theta \mapsto \R[/math] a known function such that for each [math]w\in\R[/math] and [math]\theta\in\Theta[/math], [math]f(w,x;\theta)[/math] is weakly increasing in [math]x[/math]. In the absence of additional information, what can the researcher learn about [math]\theta[/math]?
Under the Assumptions of Identification Problem, the sharp identification region for [math]\theta[/math] is
[45] study Identification Problem for the case of missing covariate data without imposing the mean independence restriction of [40] (Assumption MI in Identification Problem). As discussed in footnote, restriction MI is undesirable in this context because it implies the assumption that data are missing at random. [45] characterize [math]\idr{\theta}[/math] under the weaker assumptions, but face the problem that this characterization is usually too complex to compute or to use for inference. They therefore provide outer regions that are easier to compute, and they show that these regions are informative and relatively easy to use.
Measurement Error and Data Combination
One of the first examples of bounding analysis appears in [46], to assess the impact in linear regression of covariate measurement error. This analysis was substantially extended in [47], [48], and [49]. The more recent literature in partial identification has provided important advances to learn features of probability distributions when the observed variables are error-ridden measures of the variables of interest. Here I briefly mention some of the papers in this literature, and refer to Chapter XXX in this Volume by [50] for a thorough treatment of identification and inference with mismeasured and unobserved variables. In an influential paper, [51] study what can be learned about features of the distribution of [math]\ey|\ex[/math] in the presence of contaminated or corrupted outcome data. Whereas a contaminated sampling model assumes that data errors are statistically independent of sample realizations from the population of interest, the corrupted sampling model does not. These models are regularly used in the important literature on robust estimation (e.g., [52][53][54]). However, the goal of that literature is to characterize how point estimators of population parameters behave when data errors are generated in specified ways. As such, the inference problem is approached ex-ante: before collecting the data, one looks for point estimators that are not greatly affected by error. The question addressed by [51] is conceptually distinct. It asks what can be learned about specific population parameters ex-post, that is, after the data has been collected. For example, whereas the mean is well known not to be a robust estimator in the presence of contaminated data, [51] show that it can be (non-trivially) bounded provided the probability of contamination is strictly less than one. [55][56] and [57][58] extend the results of [51] to allow for (partial) verification of the distribution from which the data are drawn. They apply the resulting sharp bounds to learn about school performance when the observed test scores may not be valid for all students. [59] provides sharp bounds on the distribution of a misclassified outcome variable under an array of different assumptions on the extent and type of misclassification.
A completely different problem is that of data combination. Applied economists often face the problem that no single data set contains all the variables that are necessary to conduct inference on a population of interest. When this is the case, they need to integrate the information contained in different samples; for example, they might need to combine survey data with administrative data (see [60](for a survey of the econometrics of data combination)). From a methodological perspective, the problem is that while the samples being combined might contain some common variables, other variables belong only to one of the samples. When the data is collected at the same aggregation level (e.g., individual level, household level, etc.), if the common variables include a unique and correctly recorded identifier of the units constituting each sample, and there is a substantial overlap of units across all samples, then exact matching of the data sets is relatively straightforward, and the combined data set provides all the relevant information to identify features of the population of interest. However, it is rather common that there is a limited overlap in the units constituting each sample, or that variables that allow identification of units are not available in one or more of the input files, or that one sample provides information at the individual or household level (e.g., survey data) while the second sample provides information at a more aggregate level (e.g., administrative data providing information at the precinct or district level). Formally, the problem is that one observes data that identify the joint distributions [math]\sP(\ey,\ex)[/math] and [math]\sP(\ex,\ew)[/math], but not data that identifies the joint distribution [math]\sQ(\ey,\ex,\ew)[/math] whose features one wants to learn. The literature on statistical matching has aimed at using the common variable(s) [math]\ex[/math] as a bridge to create synthetic records containing [math](\ey,\ex,\ew)[/math] (see, e.g., [61](for an early contribution)). As [62] points out, the inherent assumption at the base of statistical matching is that conditional on [math]\ex[/math], [math]\ey[/math] and [math]\ew[/math] are independent. This conditional independence assumption is strong and untestable. While it does guarantee point identification of features of the conditional distributions [math]\sQ(\ey|\ex,\ew)[/math], it often finds very little justification in practice. Early on, [63] provided numerical illustrations on how one can bound the object of interest, when both [math]\ey[/math] and [math]\ew[/math] are binary variables. [64] provide a general analysis of the problem. They obtain bounds on the long regression [math]\E_\sQ(\ey|\ex,\ew)[/math], under the assumption that [math]\ew[/math] has finite support. They show that sharp bounds on [math]\E_\sQ(\ey|\ex,\ew=w)[/math] can be obtained using the results in [51], thereby establishing a connection with the analysis of contaminated data. They then derive sharp identification regions for [math][\E_\sQ(\ey|\ex=x,\ew=w),x\in\cX,w\in\cW][/math]. They show that these bounds are sharp when [math]\ey[/math] has finite support, and [65] establish sharpness without this restriction. [66] address the question of what can be learned about counterfactual distributions and treatment effects under the data scenario just described, but with [math]\ex[/math] replaced by [math]\es[/math], a binary indicator for the received treatment (using the notation of the previous section). In this case, the exogenous selection assumption (conditional on [math]\ew[/math]) does not suffice for point identification of the objects of interest. The authors derive, however, sharp bounds on these quantities using monotone rearrangement inequalities. [67] provides partial identification results for the coefficients in the linear projection of [math]\ey[/math] on [math](\ex,\ew)[/math].
Further Theoretical Advances and Empirical Applications
In order to discuss the partial identification approach to learning features of probability distributions in some level of detail while keeping this chapter to a manageable length, I have focused on a selection of papers. In this section I briefly mention several other excellent theoretical contributions that could be discussed more closely, as well as several papers that have applied partial identification analysis to answer important empirical questions.
While selectively observed data are commonplace in observational studies, in randomized experiments subjects are randomly placed in designated treatment groups conditional on [math]\ex[/math], so that the assumption of exogenous selection is satisfied with respect to the assigned treatment. Yet, identification of some highly policy relevant parameters can remain elusive in the absence of strong assumptions. One challenge results from noncompliance, where individuals' received treatments differs from the randomly assigned ones. [68] derive sharp bounds on the ATE in this context, when [math]\cY=\T=\{0,1\}[/math]. Even if one is interested in the intention-to-treat parameter, selectively observed data may continue to be a problem. For example, [69] studies the wage effects of the Job Corps training program, which randomly assigns eligibility to participate in the program. Individuals randomized to be eligible were not compelled to receive treatment, hence [69] focuses on the intention-to-treat effect. Because wages are only observable when individuals are employed, a selection problem persists despite the random assignment of eligibility to treatment, as employment status may be affected by the training program. [69] obtains sharp bounds on the intention-to-treat effect, through a trimming procedure that leverages results in [51]. [70] analyzes the problem of identification of the ATE and other treatment effects, when the received treatment is unobserved for a subset of the population. Missing treatment data may be due to item or survey nonresponse in observational studies, or noncompliance with randomly assigned treatments that are not directly monitored. She derives sharp worst case bounds leveraging results in [51], and she shows that these are a function of the available prior information on the distribution of missing treatments. If the response function is assumed monotone as in \eqref{eq:MTR:treat}, she obtains informative bounds without restrictions on the distribution of missing treatments.
Even randomly assigned treatments and perfect compliance with no missing data may not suffice for point identification of all policy relevant parameters. Important examples are given by [71] and [72]. [71] show that features of the joint distribution of the potential outcomes of treatment and control, including the distribution of treatment effects impacts, cannot be point identified in the absence of strong restrictions. This is because although subjects are randomized to treatment and control, nobody's outcome is observed under both states. Nonetheless, the authors obtain bounds for the functionals of interest. [73] derives related bounds on the probability that the potential outcome of one treatment is larger than that of the other treatment, and applies these results to health economics problems. [72] shows that features of outcome distributions under treatment rules in which treatment may vary within groups cannot be point identified in the absence of strong restrictions. This is because data resulting from randomized experiments with perfect compliance allow for point identification of the outcome distributions under treatment rules that assign all persons with the same [math]\ex[/math] to the same treatment group. However, such data only allow for partial identification of outcome distributions under rules in which treatment may vary within groups. [72] derives sharp bounds for functionals of these distributions.
Analyses of data resulting from natural experiments also face identification challenges. [74] study what can be learned about treatment effects when one uses a contaminated instrumental variable, i.e. when a mean-independence assumption holds in a population of interest, but the observed population is a mixture of the population of interest and one in which the assumption doesn't hold. They extend the results of [51] to learn about the causal effect of teenage childbearing on a teen mother's subsequent outcomes, using the natural experiment of miscarriages to form an instrumental variable for teen births. This instrument is contaminated because miscarriges may not occur randomly for a subset of the population (e.g., higher miscarriage rates are associated with smoking and drinking, and these behaviors may be correlated with the outcomes of interest).
Of course, analyses of selectively observed data present many challenges, including but not limited to the ones described in Section Selectively Observed Data. [75] generalize the difference-in-difference (DID) design to a changes-in-changes (CIC) model, where the distribution of the unobservables is allowed to vary across groups, but not overtime within groups, and the additivity and linearity assumptions of the DID are dispensed with. For the case that the outcomes have a continuous distribution, [75] provide conditions for point identification of the entire counterfactual distribution of effects of the treatment on the treatment group as well as the distribution of effects of the treatment on the control group, without restricting how these distributions differ from each other. For the case that the outcome variables are discrete, they provide partial identification results, as well as additional conditions compared to their baseline model under which point identification attains.
Motivated by the question of whether the age-adjusted mortality rate from cancer in 2000 was lower than that in the early 1970s, [76] study partial identification of competing risk models (see [77](for earlier partial identification results)). To answer this question, they need to contend with the fact that mortality rate from cardiovascular disease declined substantially over the same period of time, so that individuals that in the early 1970s might have died from cardiovascular disease before being diagnosed with cancer, do not in 2000. In this context, it is important to carry out the analysis without assuming that the underlying risks are independent. [76] show that bounds for the parameters of interest can be obtained as the solution to linear programming problems. The estimated bounds suggest much larger improvements in cancer mortality rates than previously estimated.
[78] use UK data to study changes over time in the distribution of male and female wages, and in wage inequality. Because the composition of the workforce changes over time, it is difficult to disentangle that effect from changes in the distribution of wages, given that the latter are observed only for people in the workforce. [78] begin their empirical analysis by reporting worst case bounds (as in [5]) on the CDF of wages conditional on covariates. They then consider various restrictions on treatment selection, e.g., a first order stochastic dominance assumption according to which people with higher wages are more likely to work, and derive tighter bounds under this assumption (and under weaker ones). Finally, they bring to bear shape restrictions. At each step of the analysis, they report the resulting bounds, thereby illuminating the role played by each assumption in shaping the inference. [79] provide best linear approximations to the identification region for the quantile gender wage gap using Current Population Survey repeated cross-sections data from 1975-2001, using treatment selection assumptions in the spirit of [78] as well as exclusion restrictions. [80] study the effect of Swan-Ganz catheterization on subsequent mortality.[Notes 21] Previous research had shown, using propensity score matching (assuming that there are no unobserved differences between catheterized and non catheterized patients) that Swan-Ganz catheterization increases the probability that patients die within 180 days from admission to the intensive care unit. [80] re-analyze the data using (and extending) bounds results obtained by [81]. These results are based on exclusion restrictions combined with a threshold crossing structure for both the treatment and the outcome variables in problems where [math]\cY=\cT=\{0,1\}[/math].
[80] use as instrument for Swan-Ganz catheterization the day of the week that the patient was admitted to the intensive care unit. The reasoning is that patients are less likely to be catheterized on the weekend, but the admission day to the intensive care unit is plausibly uncorrelated with subsequent mortality. Their results confirm that for some diagnoses, Swan-Ganz catheterization increases mortality at 30 days after catheterization and beyond. [82] use data from Maryland, Virginia and Illinois to learn about the impact of laws allowing individuals to carry concealed handguns (right-to-carry laws) on violent and property crimes. Point identification of these treatment effects is possible under invariance assumptions that certain features of treatment response are constant across states and years. [82] propose the use of weaker but more credible restrictions according to which these features exhibit bounded variation -- the invariance case being the limit where the bound on variation equals zero. They carry out their analysis under different combinations of the bounded variation assumptions, and at each step they report the resulting bounds, thereby illuminating the role played by each assumption in shaping the inference.
[83] provide sharp bounds on the joint distribution of potential (binary) outcomes in a Roy model with sector specific unobserved heterogeneity and self selection based on potential outcomes. The key maintained assumption is that the researcher has access to data that includes a stochastically monotone instrumental variable. This is a selection shifter that is restricted to affect potential outcomes monotonically. An example is parental education, which may not be independent from potential wages, but plausibly does not negatively affect future wages. Under this assumption, [83] show that all observable implications of the model are embodied in the stochastic monotonicity of observed outcomes in the instrument, hence Roy selection behavior can be tested by checking this stochastic monotonicity. They apply the method to estimate a Roy model of college major choice in Canada and Germany, with special interest in the under-representation of women in STEM. [84] provide a general method to obtain sharp bounds on a certain class of treatment effects parameters. This class is comprised of parameters that can be expressed as weighted averages of marginal treatment effects [8][9][10].
[85] provides a general method, based on copulas, to obtain sharp bounds on treatment effect parameters in semiparametric binary models. A notable feature of both [84] and [85] is that the bounds are obtained as solutions to convex (even linear) optimization problems, rendering them computationally attractive. [86] provide partial identification results and inference methods for a linear functional [math]\ell(g)[/math] when [math]g:\cX\mapsto\R[/math] is such that [math]\ey=g(\ex)+\epsilon[/math] and [math]\E(\ey|\ez)=0[/math]. The instrumental variable [math]\ez[/math] and regressor [math]\ex[/math] have discrete distributions, and [math]\ez[/math] has fewer points of support than [math]\ex[/math], so that [math]\ell(g)[/math] can only be partially identified. They impose shape restrictions on [math]g[/math] (e.g., monotonicity or convexity) to achieve interval identification of [math]\ell(g)[/math], and they show that the lower and upper points of the interval can be obtained by solving linear programming problems. They also show that the bootstrap can be used to carry out inference.
General references
Molinari, Francesca (2020). "Microeconometrics with Partial Identification". arXiv:2004.11751 [econ.EM].
Notes
- The bounds [math]g_0,g_1[/math] and the values [math]y_0,y_1[/math] at which they are attained may differ for different functions [math]g(\cdot)[/math].
- Section discusses the consequences of model misspecification (with respect to refutable assumptions)
- Recall that a probability distribution [math]\sF\in\cT[/math] stochastically dominates [math]\sF^\prime\in\cT[/math] if [math]\sF(-\infty,t]\le \sF^\prime(-\infty,t][/math] for all [math]t\in\R[/math]. A real-valued functional [math]\sd:\cT\to\R[/math] respects stochastic dominance if [math]\sd(\sF)\ge \sd(\sF^\prime)[/math] whenever [math]\sF[/math] stochastically dominates [math]\sF^\prime[/math].
- Earlier related work includes, e.g., [1] and [2], who obtain worst case bounds on the sample Gini coefficient under the assumption that one knows the income bracket but not the exact income of every household.
- Whereas [3] is very clear that the collection of CDFs in \eqref{eq:pointwise_bounds_F_md} is an outer region for the CDF of [math]\ey|\ex=x[/math], and [4] provides the sharp characterization in \eqref{eq:sharp_id_P_md_Manski}, [5](p. 39) does not state all the requirements that characterize [math]\idr{\sF(\ey|\ex=x)}[/math].
- Here the treatment response is a function only of the (scalar) treatment received by the given individual, an assumption known as stable unit treatment value assumption [6].
- [7] and [8](Section 2.5) provide a characterization of the sharp identification region for the joint distribution of [math][\ey(t),t\in\T][/math].
- Stronger exclusion restrictions include statistical independence of the response function at each [math]t[/math] with [math]\ez[/math]: [math]\sQ(\ey(t)|\ez,\ex)=\sQ(\ey(t)|\ex)~\forall t \in\T,~\ex[/math]-a.s.; and statistical independence of the entire response function with [math]\ez[/math]: [math]\sQ([\ey(t),t \in\T]|\ez,\ex)=\sQ([\ey(t),t \in\T]|\ex),~\ex[/math]-a.s. Examples of partial identification analysis under these conditions can be found in [9], [10], [11], [12], [13], and many others.
- See [14](Chapter XXX in this Volume) for further discussion.
- In Identification Problem the observable variables are [math](\ey\ed,\ed,\ex)[/math], and [math](\yL,\yU)[/math] are determined as follows: [math]\yL=\ey\ed+y_0(1-\ed)[/math], [math]\yU=\ey\ed+y_1(1-\ed)[/math]. For the analysis in Section Treatment Effects with and without Instrumental Variables, the data is [math](\ey,\es,\ex)[/math] and [math]\yL=\ey\one(\es=t)+y_0\one(\es\ne t)[/math], [math]\yU=\ey\one(\es=t)+y_1\one(\es\ne t)[/math]. Hence, [math]\sP(\yL\le\ey\le\yU)=1[/math] by construction.
- For a proof of this statement, see [15](Example 1.11).
- For [math]K = \cY[/math], both \eqref{eq:sharp_id_P_interval_1} and \eqref{eq:sharp_id_P_md_Manski} hold trivially.
- It can be shown that the collection of random variables [math]\ey_\eu[/math] equals the collection of measurable selections of the random closed set [math]\eY\equiv [\yL,\yU][/math] (see Definition); see [16](Lemma 2.1). Theorem provides a characterization of the distribution of any [math]\ey_\eu[/math] that satisfies [math]\ey_\eu \in \eY[/math] a.s., based on a dominance condition that relates the distribution of [math]\ey_\eu[/math] to the distribution of the random set [math]\eY[/math]. Such dominance condition is given by the inequalities in \eqref{eq:sharp_id_P_interval_1}.
- For the case of missing covariate data, which is a special case of interval covariate data similarly to arguments in footnote, [17] show that the MI restriction implies the assumption that data is missing at random.
- Under our assumption that [math]\cY[/math] is a bounded interval, all the selections of [math]\eY[/math] are integrable. [18] consider the more general case where [math]\cY[/math] is not required to be bounded.
- In [math]\R^2[/math] in our example, in [math]\R^d[/math] if [math]\ex[/math] is a [math]d-1[/math] vector and the predictor includes an intercept.
- See [19](p. 808) and [20](p. 1136).
- For example, in the case that [math]\ex[/math] is a scalar, sharp bounds on [math]\theta_1[/math] can be obtained by choosing [math]u=[0~1]^\top[/math] and [math]u=[0~-1]^\top[/math], which yield [math]\theta_1\in[\theta_{1L},\theta_{1U}][/math] with [math]\theta_{1L}=\min_{\ey\in[\yL,\yU]}\frac{Cov(\ex,\ey)}{Var(\ex)}=\frac{\E_\sP[(\ex-\E_\sP\ex)(\yL\one(\ex \gt \E_\sP\ex)+\yU\one(\ex\le\E\ex))]}{\E_\sP\ex^2-(\E_\sP\ex)^2}[/math] and [math]\theta_{1U}=\max_{\ey\in[\yL,\yU]}\frac{Cov(\ex,\ey)}{Var(\ex)}=\frac{\E_\sP[(\ex-\E_\sP\ex)(\yL\one(\ex \lt \E_\sP\ex)+\yU\one(\ex\ge\E\ex))]}{\E_\sP\ex^2-(\E_\sP\ex)^2}[/math].
- Here for simplicity I suppose that both [math]\xL[/math] and [math]\xU[/math] have bounded support. [21] do not make this simplifying assumption.
- Note that while [math]\eG[/math] is a convex set, [math]\Eps_\theta[/math] is not.
- The Swan-Ganz catheter is a device placed in patients in the intensive care unit to guide therapy.
References
- 1.0 1.1 1.2 1.3 1.4 Manski, C.F. (1989): “Anatomy of the Selection Problem” The Journal of Human Resources, 24(3), 343--360.
- Dominitz, J., and C.F. Manski (2017): “More Data or Better Data? A Statistical Decision Problem” The Review of Economic Studies, 84(4), 1583--1605.
- 3.0 3.1 3.2 3.3 Manski, C.F. (2003): Partial Identification of Probability Distributions, Springer Series in Statistics. Springer.
- 4.0 4.1 Stoye, J. (2010): “Partial identification of spread parameters” Quantitative Economics, 1(2), 323--357.
- 5.0 5.1 Manski, C.F. (1994): “The selection problem” in Advances in Econometrics: Sixth World Congress, ed. by C.A. Sims, vol.1 of Econometric Society Monographs, pp. 143--170. Cambridge University Press.
- 6.0 6.1 6.2 Molchanov, I., and F.Molinari (2018): Random Sets in Econometrics. Econometric Society Monograph Series, Cambridge University Press, Cambridge UK.
- Imbens, G.W., and J.D. Angrist (1994): “Identification and Estimation of Local Average Treatment Effects” Econometrica, 62(2), 467--475.
- 8.0 8.1 Heckman, J.J., and E.J. Vytlacil (1999): “Local Instrumental Variables and Latent Variable Models for Identifying and Bounding Treatment Effects” Proceedings of the National Academy of Sciences of the United States of America, 96(8), 4730--4734.
- 9.0 9.1 Heckman, J.J., and E.J. Vytlacil (2001): “Instrumental variables, selection models, and tight bounds on the average treatment effect” in Econometric Evaluation of Labour Market Policies, ed. by M.Lechner, and F.Pfeiffer, pp. 1--15, Heidelberg. Physica-Verlag HD.
- 10.0 10.1 Heckman, J.J., and E.J. Vytlacil (2005): “Structural Equations, Treatment Effects, and Econometric Policy Evaluation” Econometrica, 73(3), 669--738.
- Manski, C.F. (1995): Identification Problems in the Social Sciences. Harvard University Press.
- Manski, C.F. (2007a): Identification for Prediction and Decision. Harvard University Press.
- Imbens, G.W., and D.B. Rubin (2015): Causal Inference for Statistics, Social, and Biomedical Sciences: An Introduction. Cambridge University Press.
- Heckman, J.J., and E.J. Vytlacil (2007a): “Chapter 70 -- Econometric Evaluation of Social Programs, Part I: Causal Models, Structural Models and Econometric Policy Evaluation” in Handbook of Econometrics, ed. by J.J. Heckman, and E.E. Leamer, vol.6, pp. 4779 -- 4874. Elsevier.
- Heckman, J.J., and E.J. Vytlacil (2007b): “Chapter 71 -- Econometric Evaluation of Social Programs, Part II: Using the Marginal Treatment Effect to Organize Alternative Econometric Estimators to Evaluate Social Programs, and to Forecast their Effects in New Environments” in Handbook of Econometrics, ed. by J.J. Heckman, and E.E. Leamer, vol.6, pp. 4875 -- 5143. Elsevier.
- Abbring, J.H., and J.J. Heckman (2007): “Chapter 72 -- Econometric Evaluation of Social Programs, Part III: Distributional Treatment Effects, Dynamic Treatment Effects, Dynamic Discrete Choice, and General Equilibrium Policy Evaluation” in Handbook of Econometrics, ed. by J.J. Heckman, and E.E. Leamer, vol.6, pp. 5145 -- 5303. Elsevier.
- Imbens, G.W., and J.M. Wooldridge (2009): “Recent Developments in the Econometrics of Program Evaluation” Journal of Economic Literature, 47(1), 5--86.
- Mogstad, M., and A.Torgovitsky (2018): “Identification and Extrapolation of Causal Effects with Instrumental Variables” Annual Review of Economics, 10(1), 577--613.
- Neyman, J.S. (1923): “On the Application of Probability Theory to Agricultural Experiments. Essay on Principles. Section 9.” Roczniki Nauk Rolniczych, X, 1--51, reprinted in \textit{Statistical Science}, 5(4), 465-472, translated and edited by D. M. Dabrowska and T. P. Speed from the Polish original.
- 20.0 20.1 20.2 20.3 20.4 Manski, C.F. (1997b): “Monotone Treatment Response” Econometrica, 65(6), 1311--1334.
- Manski, C.F. (1990): “Nonparametric Bounds on Treatment Effects” The American Economic Review Papers and Proceedings, 80(2), 319--323.
- Manski, C.F., and J.V. Pepper (2000): “Monotone Instrumental Variables: With an Application to the Returns to Schooling” Econometrica, 68(4), 997--1010.
- Manski, C.F., and J.V. Pepper (2009): “More on monotone instrumental variables” The Econometrics Journal, 12(s1), S200--S216.
- Juster, F.T., and R.Suzman (1995): “An Overview of the Health and Retirement Study” Journal of Human Resources, 30 (Supplement), S7--S56.
- Picketty, T. (2005): “Top Income Shares in the Long Run: An Overview” Journal of the European Economic Association, 3, 382--392.
- {Bureau of Labor Statistics} (2018): “Occupational Employment Statistics” U.S. Department of Labor, available online at [www.bls.gov/oes/ www.bls.gov/oes/]; accessed 1/28/2018.
- Manski, C.F., and F.Molinari (2010): “Rounding Probabilistic Expectations in Surveys” Journal of Business and Economic Statistics, 28(2), 219--231.
- Giustinelli, P., C.F. Manski, and F.Molinari (2019b): “Tail and Center Rounding of Probabilistic Expectations in the Health and Retirement Study” available at http://faculty.wcas.northwestern.edu/cfm754/gmm_rounding.pdf.
- Artstein, Z. (1983): “Distributions of random sets and random selections” Israel Journal of Mathematics, 46, 313--324.
- Norberg, T. (1992): “On the existence of ordered couplings of random sets --- with applications” Israel Journal of Mathematics, 77, 241--264.
- 32.0 32.1 Beresteanu, A., I.Molchanov, and F.Molinari (2012): “Partial identification using random set theory” Journal of Econometrics, 166(1), 17 -- 32, with errata at https://molinari.economics.cornell.edu/docs/NOTE_BMM2012_v3.pdf.
- 33.0 33.1 Beresteanu, A., and F.Molinari (2008): “Asymptotic Properties for a Class of Partially Identified Models” Econometrica, 76(4), 763--814.
- 34.0 34.1 Beresteanu, A., I.Molchanov, and F.Molinari (2011): “Sharp identification regions in models with convex moment predictions” Econometrica, 79(6), 1785--1821.
- Chesher, A., and A.M. Rosen (2019): “Generalized instrumental variable models, methods, and applications” in Handbook of Econometrics. Elsevier.
- Tamer, E. (2010): “Partial Identification in Econometrics” Annual Review of Economics, 2, 167--195.
- Ponomareva, M., and E.Tamer (2011): “Misspecification in moment inequality models: back to moment equalities?” The Econometrics Journal, 14(2), 186--203.
- Horowitz, J.L., and C.F. Manski (1998): “Censoring of outcomes and regressors due to survey nonresponse: Identification and estimation using weights and imputations” Journal of Econometrics, 84(1), 37 -- 58.
- Horowitz, J.L., and C.F. Manski (2000): “Nonparametric Analysis of Randomized Experiments with Missing Covariate and Outcome Data” Journal of the American Statistical Association, 95(449), 77--84.
- 40.0 40.1 40.2 Manski, C.F., and E.Tamer (2002): “Inference on Regressions with Interval Data on a Regressor or Outcome” Econometrica, 70(2), 519--546.
- Stoye, J. (2007): “Bounds on Generalized Linear Predictors with Incomplete Outcome Data” Reliable Computing, 13(3), 293--302.
- Magnac, T., and E.Maurin (2008): “Partial Identification in Monotone Binary Models: Discrete Regressors and Interval Data” The Review of Economic Studies, 75(3), 835--864.
- Horowitz, J.L., C.F. Manski, M.Ponomareva, and J.Stoye (2003): “Computation of Bounds on Population Parameters When the Data Are Incomplete” Reliable Computing, 9(6), 419--440.
- Grant, M., and S.Boyd (2010): “{CVX}: Matlab Software for Disciplined Convex Programming, Version 1.21” available at http://cvxr.com/cvx.
- 45.0 45.1 Aucejo, E.M., F.A. Bugni, and V.J. Hotz (2017): “Identification and inference on regressions with missing covariate data” Econometric Theory, 33(1).
- Frisch, R. (1934): Statistical Confluence Analysis by Means of Complete Regression Systems, Okonomiske Institutt Oslo: Publikasjon. Universitetets {\O}konomiske Instituut.
- Gilstein, C.Z., and E.E. Leamer (1983): “Robust Sets of Regression Estimates” Econometrica, 51(2), 321--333.
- Klepper, S., and E.E. Leamer (1984): “Consistent Sets of Estimates for Regressions with Errors in All Variables” Econometrica, 52(1), 163--183.
- Leamer, E.E. (1987): “Errors in Variables in Linear Systems” Econometrica, 55(4), 893--909.
- Schennach, S.M. (2019): “Mismeasured and unobserved variables” in Handbook of Econometrics. Elsevier.
- 51.0 51.1 51.2 51.3 51.4 51.5 51.6 51.7 Horowitz, J.L., and C.F. Manski (1995): “Identification and Robustness with Contaminated and Corrupted Data” Econometrica, 63(2), 281--302.
- Huber, P.J. (1964): “Robust Estimation of a Location Parameter” The Annals of Mathematical Statistics, 35(1), 73--101.
- Huber, P.J. (2004): Robust Statistics, Wiley Series in Probability and Statistics - Applied Probability and Statistics Section Series. Wiley.
- Hampel, F.R., E.M. Ronchetti, P.J. Rousseeuw, and W.A. Stahel (2011): Robust Statistics: The Approach Based on Influence Functions. Wiley.
- Dominitz, J., and R.P. Sherman (2004): “Sharp bounds under contaminated or corrupted sampling with verification, with an application to environmental pollutant data” Journal of Agricultural, Biological, and Environmental Statistics, 9(3), 319--338.
- Dominitz, J., and R.P. Sherman (2005): “Identification and estimation of bounds on school performance measures: a nonparametric analysis of a mixture model with verification” Journal of Applied Econometrics, 21(8), 1295--1326.
- Kreider, B., and J.V. Pepper (2007): “Disability and Employment: Reevaluating the Evidence in Light of Reporting Errors” Journal of the American Statistical Association, 102(478), 432--441.
- Kreider, B., and J.Pepper (2008): “Inferring disability status from corrupt data” Journal of Applied Econometrics, 23(3), 329--349.
- Molinari, F. (2008): “Partial identification of probability distributions with misclassified data” Journal of Econometrics, 144(1), 81 -- 117.
- Ridder, G., and R.Moffitt (2007): “Chapter 75 -- The Econometrics of Data Combination” in Handbook of Econometrics, ed. by J.J. Heckman, and E.E. Leamer, vol.6, pp. 5469 -- 5547. Elsevier.
- Okner, B. (1972): “Constructing A New Data Base From Existing Microdata Sets: The 1966 Merge File” Annals of Economic and Social Measurement, 1(3), 325--362.
- Sims, C.A. (1972): “Comments and Rejoinder On Okner (1972)” Annals of Economic and Social Measurement, 1(3), 343--345 and 355--357.
- Duncan, O.D., and B.Davis (1953): “An Alternative to Ecological Correlation” American Sociological Review, 18(6), 665--666.
- Cross, P.J., and C.F. Manski (2002): “Regressions, Short and Long” Econometrica, 70(1), 357--368.
- Molinari, F., and M.Peski (2006): “Generalization of a Result on ``Regressions, short and long"” Econometric Theory, 22(1), 159--163.
- Fan, Y., R.Sherman, and M.Shum (2014): “Identifying Treatment Effects Under Data Combination” Econometrica, 82(2), 811--822.
- Pacini, D. (2017): “Two-sample least squares projection” Econometric Reviews, 38(1), 95--123.
- Balke, A., and J.Pearl (1997): “Bounds on Treatment Effects From Studies With Imperfect Compliance” Journal of the American Statistical Association, 92(439), 1171--1176.
- 69.0 69.1 69.2 Lee, D.S. (2009): “Training, Wages, and Sample Selection: Estimating Sharp Bounds on Treatment Effects” The Review of Economic Studies, 76(3), 1071--1102.
- Molinari, F. (2010): “Missing Treatments” Journal of Business and Economic Statistics, 28(1), 82--95.
- 71.0 71.1 Heckman, J.J., J.Smith, and N.Clements (1997): “Making the Most Out of Programme Evaluations and Social Experiments: Accounting for Heterogeneity in Programme Impacts” The Review of Economic Studies, 64(4), 487--535.
- 72.0 72.1 72.2 Manski, C.F. (1997a): “The Mixing Problem in Programme Evaluation” The Review of Economic Studies, 64(4), 537--553.
- Mullahy, J. (2018): “Individual results may vary: Inequality-probability bounds for some health-outcome treatment effects” Journal of Health Economics, 61, 151 -- 162.
- Hotz, V.J., C.H. Mullin, and S.G. Sanders (1997): “Bounding Causal Effects Using Data From a Contaminated Natural Experiment: Analysing the Effects of Teenage Chilbearing” The Review of Economic Studies, 64(4), 575--603.
- 75.0 75.1 Athey, S., and G.W. Imbens (2006): “Identification and Inference in Nonlinear Difference-in-Differences Models” Econometrica, 74(2), 431--497.
- 76.0 76.1 Honoré, B.E., and A.Lleras-Muney (2006): “Bounds in Competing Risks Models and the War on Cancer” Econometrica, 74(6), 1675--1698.
- Peterson, A.V. (1976): “Bounds for a Joint Distribution Function with Fixed Sub-Distribution Functions: Application to Competing Risks” Proceedings of the National Academy of Sciences of the United States of America, 73(1), 11--13.
- 78.0 78.1 78.2 Blundell, R., A.Gosling, H.Ichimura, and C.Meghir (2007): “Changes in the Distribution of Male and Female Wages Accounting for Employment Composition Using Bounds” Econometrica, 75(2), 323--363.
- Chandrasekhar, A., V.Chernozhukov, F.Molinari, and P.Schrimpf (2018): “Best linear approximations to set identified functions: with an application to the gender wage gap” CeMMAP working paper CWP09/19, available at https://www.cemmap.ac.uk/publication/id/13913.
- 80.0 80.1 80.2 Bhattacharya, J., A.M. Shaikh, and E.Vytlacil (2012): “Treatment effect bounds: An application to Swan–Ganz catheterization” Journal of Econometrics, 168(2), 223 -- 243.
- Shaikh, A.M., and E.J. Vytlacil (2011): “Partial identification in triangular systems of equations with binary dependent variables” Econometrica, 79(3), 949--955.
- 82.0 82.1 Manski, C.F., and J.V. Pepper (2018): “How Do Right-to-Carry Laws Affect Crime Rates? Coping with Ambiguity Using Bounded-Variation Assumptions” The Review of Economics and Statistics, 100(2), 232--244.
- 83.0 83.1 Mourifi\'{e}, I., M.Henry, and R.M\'{e}ango (2018): “Sharp Bounds and Testability of a Roy Model of STEM Major Choices” available at https://ssrn.com/abstract=2043117.
- 84.0 84.1 Mogstad, M., A.Santos, and A.Torgovitsky (2018): “Using Instrumental Variables for Inference About Policy Relevant Treatment Parameters” Econometrica, 86(5), 1589--1619.
- 85.0 85.1 Torgovitsky, A. (2019b): “Partial identification by extending subdistributions” Quantitative Economics, 10(1), 105--144.
- Freyberger, J., and J.L. Horowitz (2015): “Identification and shape restrictions in nonparametric instrumental variables estimation” Journal of Econometrics, 189(1), 41--53.