Overview
Statistical learning theory is a framework for machine learning drawing from the fields of statistics and functional analysis.[1][2][3] Statistical learning theory deals with the statistical inference problem of finding a predictive function based on data.
Introduction
The goals of learning are understanding and prediction. Learning falls into many categories, including supervised learning, unsupervised learning, online learning, and reinforcement learning. From the perspective of statistical learning theory, supervised learning is best understood.[4] Supervised learning involves learning from a training set of data. Every point in the training is an input-output pair, where the input maps to an output. The learning problem consists of inferring the function that maps between the input and the output, such that the learned function can be used to predict the output from future input.
Depending on the type of output, supervised learning problems are either problems of regression or problems of classification. If the output takes a continuous range of values, it is a regression problem. Using Ohm's Law as an example, a regression could be performed with voltage as input and current as an output. The regression would find the functional relationship between voltage and current to be math>R</math>, such that
Classification problems are those for which the output will be an element from a discrete set of labels. Classification is very common for machine learning applications. In facial recognition, for instance, a picture of a person's face would be the input, and the output label would be that person's name. The input would be represented by a large multidimensional vector whose elements represent pixels in the picture.
After learning a function based on the training set data, that function is validated on a test set of data, data that did not appear in the training set.
Formal description
Take [math]X[/math] to be the vector space of all possible inputs, and [math]Y[/math] to be the vector space of all possible outputs. Statistical learning theory takes the perspective that there is some unknown probability distribution over the product space [math]Z = X \times Y[/math], i.e. there exists some unknown [math]p(z) = p(\vec{x},y)[/math]. The training set is made up of [math]n[/math] samples from this probability distribution, and is notated
Every [math]\vec{x}_i[/math] is an input vector from the training data, and [math]y_i[/math]is the output that corresponds to it.
In this formalism, the inference problem consists of finding a function [math]f: X \to Y[/math] such that [math]f(\vec{x}) \sim y[/math]. Let [math]\mathcal{H}[/math] be a space of functions [math]f: X \to Y[/math] called the hypothesis space. The hypothesis space is the space of functions the algorithm will search through. Let [math]V(f(\vec{x}),y)[/math] be the loss function, a metric for the difference between the predicted value [math]f(\vec{x})[/math] and the actual value [math]y[/math]. The expected risk is defined to be
The target function, the best possible function [math]f[/math] that can be chosen, is given by the [math]f[/math] that satisfies
Because the probability distribution [math]p(\vec{x},y)[/math] is unknown, a proxy measure for the expected risk must be used. This measure is based on the training set, a sample from this unknown probability distribution. It is called the empirical risk
A learning algorithm that chooses the function [math]f_S[/math] that minimizes the empirical risk is called empirical risk minimization.
Loss functions
The choice of loss function is a determining factor on the function [math]f_S[/math] that will be chosen by the learning algorithm. The loss function also affects the convergence rate for an algorithm. It is important for the loss function to be convex.[5]
Different loss functions are used depending on whether the problem is one of regression or one of classification.
Regression
The most common loss function for regression is the square loss function (also known as the L2-norm). This familiar loss function is used in Ordinary Least Squares regression. The form is:
The absolute value loss (also known as the L1-norm) is also sometimes used:
Classification
In some sense the 0-1 indicator function is the most natural loss function for classification. It takes the value 0 if the predicted output is the same as the actual output, and it takes the value 1 if the predicted output is different from the actual output.
Regularization
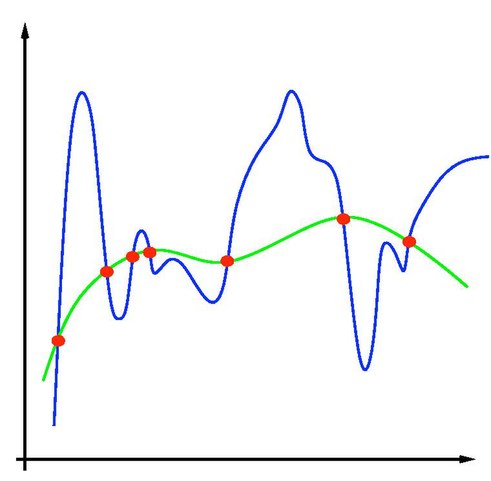
In machine learning problems, a major problem that arises is that of overfitting. Because learning is a prediction problem, the goal is not to find a function that most closely fits the (previously observed) data, but to find one that will most accurately predict output from future input. Empirical risk minimization runs this risk of overfitting: finding a function that matches the data exactly but does not predict future output well.
Overfitting is symptomatic of unstable solutions; a small perturbation in the training set data would cause a large variation in the learned function. It can be shown that if the stability for the solution can be guaranteed, generalization and consistency are guaranteed as well.[6][7] Regularization can solve the overfitting problem and give the problem stability.
Regularization can be accomplished by restricting the hypothesis space [math]\mathcal{H}[/math]. A common example would be restricting [math]\mathcal{H}[/math] to linear functions: this can be seen as a reduction to the standard problem of linear regression. [math]\mathcal{H}[/math] could also be restricted to polynomial of degree [math]p[/math], exponentials, or bounded functions on L1. Restriction of the hypothesis space avoids overfitting because the form of the potential functions are limited, and so does not allow for the choice of a function that gives empirical risk arbitrarily close to zero.
One example of regularization is Tikhonov regularization. This consists of minimizing
where [math]\gamma[/math] is a fixed and positive parameter, the regularization parameter. Tikhonov regularization ensures existence, uniqueness, and stability of the solution.[8]
Supervised Learning
Supervised learning (SL) is the machine learning]task of learning a function that maps an input to an output based on example input-output pairs.[9] It infers a function from labeled training data consisting of a set of training examples.[10] In supervised learning, each example is a pair consisting of an input object (typically a vector) and a desired output value (also called the supervisory signal). A supervised learning algorithm analyzes the training data and produces an inferred function, which can be used for mapping new examples. An optimal scenario will allow for the algorithm to correctly determine the class labels for unseen instances. This requires the learning algorithm to generalize from the training data to unseen situations in a "reasonable" way.
Steps to follow
To solve a given problem of supervised learning, one has to perform the following steps:
- Determine the type of training examples. Before doing anything else, the user should decide what kind of data is to be used as a training set. In the case of handwriting analysis, for example, this might be a single handwritten character, an entire handwritten word, an entire sentence of handwriting or perhaps a full paragraph of handwriting.
- Gather a training set. The training set needs to be representative of the real-world use of the function. Thus, a set of input objects is gathered and corresponding outputs are also gathered, either from human experts or from measurements.
- Determine the input feature representation of the learned function. The accuracy of the learned function depends strongly on how the input object is represented. Typically, the input object is transformed into a feature vector, which contains a number of features that are descriptive of the object. The number of features should not be too large, because of the curse of dimensionality; but should contain enough information to accurately predict the output.
- Determine the structure of the learned function and corresponding learning algorithm. For example, the engineer may choose to use support-vector machines or decision trees.
- Complete the design. Run the learning algorithm on the gathered training set. Some supervised learning algorithms require the user to determine certain control parameters. These parameters may be adjusted by optimizing performance on a subset (called a validation set) of the training set, or via cross-validation.
- Evaluate the accuracy of the learned function. After parameter adjustment and learning, the performance of the resulting function should be measured on a test set that is separate from the training set.
Algorithm choice
A wide range of supervised learning algorithms are available, each with its strengths and weaknesses. There is no single learning algorithm that works best on all supervised learning problems. There are four major issues to consider in supervised learning:
Bias-variance tradeoff
A first issue is the tradeoff between bias and variance.[11] Imagine that we have available several different, but equally good, training data sets. A learning algorithm is biased for a particular input [math]x[/math] if, when trained on each of these data sets, it is systematically incorrect when predicting the correct output for [math]x[/math]. A learning algorithm has high variance for a particular input [math]x[/math] if it predicts different output values when trained on different training sets. The prediction error of a learned classifier is related to the sum of the bias and the variance of the learning algorithm.[12] Generally, there is a tradeoff between bias and variance. A learning algorithm with low bias must be "flexible" so that it can fit the data well. But if the learning algorithm is too flexible, it will fit each training data set differently, and hence have high variance. A key aspect of many supervised learning methods is that they are able to adjust this tradeoff between bias and variance (either automatically or by providing a bias/variance parameter that the user can adjust).
Function complexity and amount of training data
The second issue is the amount of training data available relative to the complexity of the "true" function (classifier or regression function). If the true function is simple, then an "inflexible" learning algorithm with high bias and low variance will be able to learn it from a small amount of data. But if the true function is highly complex (e.g., because it involves complex interactions among many different input features and behaves differently in different parts of the input space), then the function will only be able to learn from a very large amount of training data and using a "flexible" learning algorithm with low bias and high variance. There is a clear demarcation between the input and the desired output.
Dimensionality of the input space
A third issue is the dimensionality of the input space. If the input feature vectors have very high dimension, the learning problem can be difficult even if the true function only depends on a small number of those features. This is because the many "extra" dimensions can confuse the learning algorithm and cause it to have high variance. Hence, high input dimensional typically requires tuning the classifier to have low variance and high bias. In practice, if the engineer can manually remove irrelevant features from the input data, this is likely to improve the accuracy of the learned function. In addition, there are many algorithms for feature selection that seek to identify the relevant features and discard the irrelevant ones. This is an instance of the more general strategy of dimensionality reduction, which seeks to map the input data into a lower-dimensional space prior to running the supervised learning algorithm.
Noise in the output values
A fourth issue is the degree of noise in the desired output values (the supervisory target variables). If the desired output values are often incorrect (because of human error or sensor errors), then the learning algorithm should not attempt to find a function that exactly matches the training examples. Attempting to fit the data too carefully leads to overfitting. You can overfit even when there are no measurement errors (stochastic noise) if the function you are trying to learn is too complex for your learning model. In such a situation, the part of the target function that cannot be modeled "corrupts" your training data - this phenomenon has been called deterministic noise. When either type of noise is present, it is better to go with a higher bias, lower variance estimator.
In practice, there are several approaches to alleviate noise in the output values such as early stopping to prevent overfitting as well as detecting and removing the noisy training examples prior to training the supervised learning algorithm. There are several algorithms that identify noisy training examples and removing the suspected noisy training examples prior to training has decreased generalization error with statistical significance.[13][14]
Other factors to consider
Other factors to consider when choosing and applying a learning algorithm include the following:
Factor | Description |
---|---|
Heterogeneity of the data | If the feature vectors include features of many different kinds (discrete, discrete ordered, counts, continuous values), some algorithms are easier to apply than others. Many algorithms, including support-vector machines, linear regression, logistic regression, neural networks, and nearest neighbor methods, require that the input features be numerical and scaled to similar ranges (e.g., to the [-1,1] interval). Methods that employ a distance function, such as nearest neighbor methods and support-vector machines with Gaussian kernels, are particularly sensitive to this. An advantage of decision trees is that they easily handle heterogeneous data. |
Redundancy in the data | If the input features contain redundant information (e.g., highly correlated features), some learning algorithms (e.g., linear regression, logistic regression, and distance based methods) will perform poorly because of numerical instabilities. These problems can often be solved by imposing some form of regularization. |
Presence of interactions and non-linearities | If each of the features makes an independent contribution to the output, then algorithms based on linear functions (e.g., linear regression, logistic regression, support-vector machines, naive Bayes) and distance functions (e.g., nearest neighbor methods, support-vector machines with Gaussian kernels) generally perform well. However, if there are complex interactions among features, then algorithms such as decision trees and neural networks work better, because they are specifically designed to discover these interactions. Linear methods can also be applied, but the engineer must manually specify the interactions when using them. |
When considering a new application, the engineer can compare multiple learning algorithms and experimentally determine which one works best on the problem at hand (see cross validation). Tuning the performance of a learning algorithm can be very time-consuming. Given fixed resources, it is often better to spend more time collecting additional training data and more informative features than it is to spend extra time tuning the learning algorithms.
How supervised learning algorithms work
Given a set of [math]N[/math] training examples of the form [math]\{(x_1, y_1), ..., (x_N,\; y_N)\}[/math] such that [math]x_i[/math] is the feature vector of the [math]i[/math]-th example and [math]y_i[/math] is its label (i.e., class), a learning algorithm seeks a function [math]g: X \to Y[/math], where [math]X[/math] is the input space and [math]Y[/math] is the output space. The function [math]g[/math] is an element of some space of possible functions [math]G[/math], usually called the hypothesis space. It is sometimes convenient to represent [math]g[/math] using a scoring function [math]f: X \times Y \to \mathbb{R}[/math] such that [math]g[/math] is defined as returning the [math]y[/math] value that gives the highest score: [math]g(x) = \underset{y}{\arg\max} \; f(x,y)[/math]. Let [math]F[/math] denote the space of scoring functions.
Although [math]G[/math] and [math]F[/math] can be any space of functions, many learning algorithms are probabilistic models where [math]g[/math] takes the form of a conditional probability model [math]g(x) = P(y|x)[/math], or [math]f[/math] takes the form of a joint probability model [math]f(x,y) = P(x,y)[/math]. For example, naive Bayes and linear discriminant analysis are joint probability models, whereas logistic regression is a conditional probability model.
There are two basic approaches to choosing [math]f[/math] or [math]g[/math]: empirical risk minimization and structural risk minimization.[15] Empirical risk minimization seeks the function that best fits the training data. Structural risk minimization includes a penalty function that controls the bias/variance tradeoff.
In both cases, it is assumed that the training set consists of a sample of independent and identically distributed pairs, [math](x_i, \;y_i)[/math]. In order to measure how well a function fits the training data, a loss function [math]L: Y \times Y \to \mathbb{R}^{\ge 0}[/math] is defined. For training example [math](x_i,\;y_i)[/math], the loss of predicting the value [math]\hat{y}[/math] is [math]L(y_i,\hat{y})[/math].
The risk [math]R(g)[/math] of function [math]g[/math] is defined as the expected loss of [math]g[/math]. This can be estimated from the training data as
Empirical risk minimization
In empirical risk minimization, the supervised learning algorithm seeks the function [math]g[/math] that minimizes [math]R(g)[/math]. Hence, a supervised learning algorithm can be constructed by applying an optimization algorithm to find [math]g[/math].
When [math]g[/math] is a conditional probability distribution [math]P(y|x)[/math] and the loss function is the negative log likelihood: [math]L(y, \hat{y}) = -\log P(y | x)[/math], then empirical risk minimization is equivalent to maximum likelihood estimation.
When [math]G[/math] contains many candidate functions or the training set is not sufficiently large, empirical risk minimization leads to high variance and poor generalization. The learning algorithm is able to memorize the training examples without generalizing well. This is called overfitting.
Structural risk minimization
Structural risk minimization seeks to prevent overfitting by incorporating a regularization penalty into the optimization. The regularization penalty can be viewed as implementing a form of Occam's razor that prefers simpler functions over more complex ones.
A wide variety of penalties have been employed that correspond to different definitions of complexity. For example, consider the case where the function [math]g[/math] is a linear function of the form
A popular regularization penalty is [math]\sum_j \beta_j^2[/math], which is the squared Euclidean norm of the weights, also known as the [math]L_2[/math] norm. Other norms include the [math]L_1[/math] norm, [math]\sum_j |\beta_j|[/math], and the [math]L_0[/math] "norm", which is the number of non-zero [math]\beta_j[/math]s. The penalty will be denoted by [math]C(g)[/math].
The supervised learning optimization problem is to find the function [math]g[/math] that minimizes
The parameter [math]\lambda[/math] controls the bias-variance tradeoff. When [math]\lambda = 0[/math], this gives empirical risk minimization with low bias and high variance. When [math]\lambda[/math] is large, the learning algorithm will have high bias and low variance. The value of [math]\lambda[/math] can be chosen empirically via cross validation.
Unsupervised Learning
Unsupervised learning is a type of algorithm that learns patterns from untagged data. The hope is that through mimicry, which is an important mode of learning in people, the machine is forced to build a compact internal representation of its world and then generate imaginative content from it. In contrast to supervised learning where data is tagged by an expert, e.g. as a "ball" or "fish", unsupervised methods exhibit self-organization that captures patterns as probability densities [16] or a combination of neural feature preferences.
Two broad methods in unsupervised learning are neural networks and probabilistic methods.
Probabilistic methods
Two of the main methods used in unsupervised learning are principal component and cluster analysis. Cluster analysis is used in unsupervised learning to group, or segment, datasets with shared attributes in order to extrapolate algorithmic relationships.[17] Cluster analysis is a branch of machine learning that groups the data that has not been labelled, classified or categorized. Instead of responding to feedback, cluster analysis identifies commonalities in the data and reacts based on the presence or absence of such commonalities in each new piece of data. This approach helps detect anomalous data points that do not fit into either group.
References
- Vladimir Vapnik (1995) The Nature of Statistical Learning Theory, Springer New York ISBN 978-1-475-72440-0.
- Trevor Hastie, Robert Tibshirani, Jerome Friedman (2009) The Elements of Statistical Learning, Springer-Verlag ISBN 978-0-387-84857-0.
- Mohri, Mehryar; Rostamizadeh, Afshin; Talwalkar, Ameet (2012). Foundations of Machine Learning. USA, Massachusetts: MIT Press. ISBN 9780262018258.
- Tomaso Poggio, Lorenzo Rosasco, et al. Statistical Learning Theory and Applications, 2012, Class 1
- Rosasco, L., Vito, E.D., Caponnetto, A., Fiana, M., and Verri A. 2004. Neural computation Vol 16, pp 1063-1076
- Vapnik, V.N. and Chervonenkis, A.Y. 1971. On the uniform convergence of relative frequencies of events to their probabilities. Theory of Probability and Its Applications Vol 16, pp 264-280.
- Mukherjee, S., Niyogi, P. Poggio, T., and Rifkin, R. 2006. Learning theory: stability is sufficient for generalization and necessary and sufficient for consistency of empirical risk minimization. Advances in Computational Mathematics. Vol 25, pp 161-193.
- Tomaso Poggio, Lorenzo Rosasco, et al. Statistical Learning Theory and Applications, 2012, Class 2
- Stuart J. Russell, Peter Norvig (2010) Artificial Intelligence: A Modern Approach, Third Edition, Prentice Hall ISBN 9780136042594.
- Mehryar Mohri, Afshin Rostamizadeh, Ameet Talwalkar (2012) Foundations of Machine Learning, The MIT Press ISBN 9780262018258.
- S. Geman, E. Bienenstock, and R. Doursat (1992). Neural networks and the bias/variance dilemma. Neural Computation 4, 1–58.
- G. James (2003) Variance and Bias for General Loss Functions, Machine Learning 51, 115-135. (http://www-bcf.usc.edu/~gareth/research/bv.pdf)
- C.E. Brodely and M.A. Friedl (1999). Identifying and Eliminating Mislabeled Training Instances, Journal of Artificial Intelligence Research 11, 131-167. (http://jair.org/media/606/live-606-1803-jair.pdf)
- M.R. Smith and T. Martinez (2011). "Improving Classification Accuracy by Identifying and Removing Instances that Should Be Misclassified". Proceedings of International Joint Conference on Neural Networks (IJCNN 2011). pp. 2690–2697. CiteSeerX 10.1.1.221.1371. doi:10.1109/IJCNN.2011.6033571.
- Vapnik, V. N. The Nature of Statistical Learning Theory (2nd Ed.), Springer Verlag, 2000.
- Hinton, Geoffrey; Sejnowski, Terrence (1999). Unsupervised Learning: Foundations of Neural Computation. MIT Press. ISBN 978-0262581684.
- Roman, Victor (2019-04-21). "Unsupervised Machine Learning: Clustering Analysis". Medium. Retrieved 2019-10-01.
Wikipedia References
- Wikipedia contributors. "Statistical learning theory". Wikipedia. Wikipedia. Retrieved 17 August 2022.
- Wikipedia contributors. "Supervised learning". Wikipedia. Wikipedia. Retrieved 17 August 2022.
- Wikipedia contributors. "Unsupervised learning". Wikipedia. Wikipedia. Retrieved 17 August 2022.