Matrix estimation
[math] \newcommand{\DS}{\displaystyle} \newcommand{\intbeta}{\lfloor \beta \rfloor} \newcommand{\cA}{\mathcal{A}} \newcommand{\cB}{\mathcal{B}} \newcommand{\cC}{\mathcal{C}} \newcommand{\cD}{\mathcal{D}} \newcommand{\cE}{\mathcal{E}} \newcommand{\cF}{\mathcal{F}} \newcommand{\cG}{\mathcal{G}} \newcommand{\cH}{\mathcal{H}} \newcommand{\cI}{\mathcal{I}} \newcommand{\cJ}{\mathcal{J}} \newcommand{\cK}{\mathcal{K}} \newcommand{\cL}{\mathcal{L}} \newcommand{\cM}{\mathcal{M}} \newcommand{\cN}{\mathcal{N}} \newcommand{\cO}{\mathcal{O}} \newcommand{\cP}{\mathcal{P}} \newcommand{\cQ}{\mathcal{Q}} \newcommand{\cS}{\mathcal{S}} \newcommand{\cT}{\mathcal{T}} \newcommand{\cU}{\mathcal{U}} \newcommand{\cV}{\mathcal{V}} \newcommand{\cX}{\mathcal{X}} \newcommand{\cY}{\mathcal{Y}} \newcommand{\cZ}{\mathcal{Z}} \newcommand{\sg}{\mathsf{subG}} \newcommand{\subE}{\mathsf{subE}} \newcommand{\bA}{\mathbf{A}} \newcommand{\bB}{\mathbf{B}} \newcommand{\bC}{\mathbf{C}} \newcommand{\bD}{\mathbf{D}} \newcommand{\bE}{\mathbf{E}} \newcommand{\bF}{\mathbf{F}} \newcommand{\bG}{\mathbf{G}} \newcommand{\bH}{\mathbf{H}} \newcommand{\bI}{\mathbf{I}} \newcommand{\bJ}{\mathbf{J}} \newcommand{\bK}{\mathbf{K}} \newcommand{\bM}{\mathbf{M}} \newcommand{\bN}{\mathbf{N}} \newcommand{\bO}{\mathbf{O}} \newcommand{\bP}{\mathbf{P}} \newcommand{\bp}{\mathbf{p}} \newcommand{\bQ}{\mathbf{Q}} \newcommand{\bS}{\mathbf{S}} \newcommand{\bT}{\mathbf{T}} \newcommand{\bU}{\mathbf{U}} \newcommand{\bV}{\mathbf{V}} \newcommand{\bX}{\mathbf{X}} \newcommand{\bY}{\mathbf{Y}} \newcommand{\bZ}{\mathbf{Z}} \newcommand{\bflambda}{\boldsymbol{\lambda}} \newcommand{\bftheta}{\boldsymbol{\theta}} \newcommand{\bfg}{\boldsymbol{g}} \newcommand{\bfy}{\boldsymbol{y}} \def\thetaphat{\hat{\bftheta}_\bp} \def\bflam{\boldsymbol{\lambda}} \def\Lam{\Lambda} \def\lam{\lambda} \def\bfpi{\boldsymbol{\pi}} \def\bfz{\boldsymbol{z}} \def\bfw{\boldsymbol{w}} \def\bfeta{\boldsymbol{\eta}} \newcommand{\R}{\mathrm{ I}\kern-0.18em\mathrm{ R}} \newcommand{\h}{\mathrm{ I}\kern-0.18em\mathrm{ H}} \newcommand{\K}{\mathrm{ I}\kern-0.18em\mathrm{ K}} \newcommand{\p}{\mathrm{ I}\kern-0.18em\mathrm{ P}} \newcommand{\E}{\mathrm{ I}\kern-0.18em\mathrm{ E}} \newcommand{\1}{\mathrm{ 1}\kern-0.24em\mathrm{ I}} \newcommand{\N}{\mathrm{ I}\kern-0.18em\mathrm{ N}} \newcommand{\field}[1]{\mathbb{#1}} \newcommand{\q}{\field{Q}} \newcommand{\Z}{\field{Z}} \newcommand{\X}{\field{X}} \newcommand{\Y}{\field{Y}} \newcommand{\bbS}{\field{S}} \newcommand{\n}{\mathcal{N}} \newcommand{\x}{\mathcal{X}} \newcommand{\pp}{{\sf p}} \newcommand{\hh}{{\sf h}} \newcommand{\ff}{{\sf f}} \newcommand{\Bern}{\mathsf{Ber}} \newcommand{\Bin}{\mathsf{Bin}} \newcommand{\Lap}{\mathsf{Lap}} \newcommand{\tr}{\mathsf{Tr}} \newcommand{\phin}{\varphi_n} \newcommand{\phinb}{\overline \varphi_n(t)} \newcommand{\pn}{\p_{\kern-0.25em n}} \newcommand{\pnm}{\p_{\kern-0.25em n,m}} \newcommand{\psubm}{\p_{\kern-0.25em m}} \newcommand{\psubp}{\p_{\kern-0.25em p}} \newcommand{\cfi}{\cF_{\kern-0.25em \infty}} \newcommand{\e}{\mathrm{e}} \newcommand{\ic}{\mathrm{i}} \newcommand{\Leb}{\mathrm{Leb}_d} \newcommand{\Var}{\mathrm{Var}} \newcommand{\ddelta}{d_{\symdiffsmall}} \newcommand{\dsubh}{d_H} \newcommand{\indep}{\perp\kern-0.95em{\perp}} \newcommand{\supp}{\mathop{\mathrm{supp}}} \newcommand{\rank}{\mathop{\mathrm{rank}}} \newcommand{\vol}{\mathop{\mathrm{vol}}} \newcommand{\conv}{\mathop{\mathrm{conv}}} \newcommand{\card}{\mathop{\mathrm{card}}} \newcommand{\argmin}{\mathop{\mathrm{argmin}}} \newcommand{\argmax}{\mathop{\mathrm{argmax}}} \newcommand{\ud}{\mathrm{d}} \newcommand{\var}{\mathrm{var}} \newcommand{\re}{\mathrm{Re}} \newcommand{\MSE}{\mathsf{MSE}} \newcommand{\im}{\mathrm{Im}} \newcommand{\epr}{\hfill\hbox{\hskip 4pt\vrule width 5pt height 6pt depth 1.5pt}\vspace{0.5cm}\par} \newcommand{\bi}[1]{^{(#1)}} \newcommand{\eps}{\varepsilon} \newcommand{\Deq}{\stackrel{\mathcal{D}}{=}} \newcommand{\ubar}{\underbar} \newcommand{\Kbeta}{K_{\hspace{-0.3mm} \beta}} \newcommand{\crzero}[1]{\overset{r_0}{\underset{#1}{\longleftrightarrow}}} \newcommand{\hint}[1]{\texttt{[Hint:#1]}} \newcommand{\vp}{\vspace{.25cm}} \newcommand{\vm}{\vspace{.5cm}} \newcommand{\vg}{\vspace{1cm}} \newcommand{\vgneg}{\vspace{-1cm}} \newcommand{\vneg}{\vspace{-.5cm}} \newcommand{\vpneg}{\vspace{-.25cm}} \newcommand{\tp}{\ptsize{10}} \newcommand{\douzp}{\ptsize{12}} \newcommand{\np}{\ptsize{9}} \newcommand{\hp}{\ptsize{8}} \newcommand{\red}{\color{red}} \newcommand{\ndpr}[1]{{\textsf{\red[#1]}}} \newcommandi.i.d{i.i.d\@ifnextchar.{}{.\@\xspace} } \newcommand\MoveEqLeft[1][2]{\kern #1em & \kern -#1em} \newcommand{\leadeq}[2][4]{\MoveEqLeft[#1] #2 \nonumber} \newcommand{\leadeqnum}[2][4]{\MoveEqLeft[#1] #2} \newcommand{\MIT}[1]{{\color{MITred} #1}} \newcommand{\dHyp}{\{-1,1\}^d} \newcommand{\thetahard}{\hat \theta^{hrd}} \newcommand{\thetasoft}{\hat \theta^{sft}} \newcommand{\thetabic}{\hat \theta^{bic}} \newcommand{\thetalasso}{\hat \theta^{\cL}} \newcommand{\thetaslope}{\hat \theta^{\cS}} \newcommand{\thetahard}{\hat \theta^{hrd}} \newcommand{\thetasoft}{\hat \theta^{sft}} \newcommand{\thetabic}{\hat \theta^{bic}} \newcommand{\thetalasso}{\hat \theta^{\cL}} \newcommand{\thetaslope}{\hat \theta^{\cS}} \newcommand{\thetals}{\hat \theta^{ls}} \newcommand{\thetalsm}{\tilde \theta^{ls_X}} \newcommand{\thetaridge}{\hat\theta^{\mathrm{ridge}}_\tau} \newcommand{\thetalsK}{\hat \theta^{ls}_K} \newcommand{\muls}{\hat \mu^{ls}} \newcommand{\Thetasvt}{\hat \Theta^{SVT}} \newcommand{\Thetarank}{\hat \Theta^{RK}} \newcommand{\KL}{\mathsf{KL}} \newcommand{\TV}{\mathsf{TV}} [/math]
Over the past decade or so, matrices have entered the picture of high-dimensional statistics for several reasons. Perhaps the simplest explanation is that they are the most natural extension of vectors. While this is true, and we will see examples where the extension from vectors to matrices is straightforward, matrices have a much richer structure than vectors allowing interaction between their rows and columns. In particular, while we have been describing simple vectors in terms of their sparsity, here we can measure the complexity of a matrix by its rank. This feature was successfully employed in a variety of applications ranging from multi-task learning to collaborative filtering. This last application was made popular by the NETFLIX prize in particular. In this chapter, we study several statistical problems where the parameter of interest [math]\theta[/math] is a matrix rather than a vector. These problems include multivariate regression, covariance matrix estimation, and principal component analysis. Before getting to these topics, we begin with a quick reminder on matrices and linear algebra.
Basic facts about matrices
Matrices are much more complicated objects than vectors. In particular, while vectors can be identified with linear operators from [math]\R^d[/math] to [math]\R[/math], matrices can be identified to linear operators from [math]\R^d[/math] to [math]\R^n[/math] for [math]n \ge 1[/math]. This seemingly simple fact gives rise to a profusion of notions and properties as illustrated by Bernstein's book [1] that contains facts about matrices over more than a thousand pages. Fortunately, we will be needing only a small number of such properties, which can be found in the excellent book [2], which has become a standard reference on matrices and numerical linear algebra.
Singular value decomposition
Let [math]A=\{a_{ij}, 1\le i \le m, 1\le j \le n\}[/math] be a [math]m \times n[/math] real matrix of rank [math]r \le \min(m,n)[/math]. The Singular Value Decomposition (SVD) of [math]A[/math] is given by
where [math]D[/math] is an [math]r\times r[/math] diagonal matrix with positive diagonal entries [math]\{\lambda_1, \ldots, \lambda_r\}[/math], [math]U[/math] is a matrix with columns [math]\{u_1, \ldots, u_r\} \in \R^m[/math] that are orthonormal, and [math]V[/math] is a matrix with columns [math]\{v_1, \ldots, v_r\} \in \R^n[/math] that are also orthonormal. Moreover, it holds that
for [math]j =1, \ldots, r[/math]. The values [math]\lambda_j \gt 0[/math] are called singular values of [math]A[/math] and are uniquely defined. If rank [math]r \lt \min(n,m)[/math] then the singular values of [math]A[/math] are given by [math]\lambda=(\lambda_1, \ldots, \lambda_r, 0, \ldots, 0)^\top \in \R^{\min(n,m)}[/math] where there are [math]\min(n,m)-r[/math] zeros. This way, the vector [math]\lambda[/math] of singular values of a [math]n\times m[/math] matrix is a vector in [math]\R^{\min(n,m)}[/math]. In particular, if [math]A[/math] is an [math]n \times n[/math] symmetric positive semidefinite (PSD), i.e. [math]A^\top=A[/math] and [math]u^\top A u\ge 0[/math] for all [math]u \in \R^n[/math], then the singular values of [math]A[/math] are equal to its eigenvalues. The largest singular value of [math]A[/math] denoted by [math]\lambda_{\max{}}(A)[/math] also satisfies the following variational formulation:
In the case of a [math]n \times n[/math] PSD matrix [math]A[/math], we have
In these notes, we always assume that eigenvectors and singular vectors have unit norm.
Norms and inner product
Let [math]A=\{a_{ij}\}[/math] and [math]B=\{b_{ij}\}[/math] be two real matrices. Their size will be implicit in the following notation.
Vector norms
The simplest way to treat a matrix is to deal with it as if it were a vector. In particular, we can extend [math]\ell_q[/math] norms to matrices:
The cases where [math]q \in \{0,\infty\}[/math] can also be extended matrices:
The case [math]q=2[/math] plays a particular role for matrices and [math]|A|_2[/math] is called the Frobenius norm of [math]A[/math] and is often denoted by [math]\|A\|_F[/math]. It is also the Hilbert-Schmidt norm associated to the inner product:
Spectral norms
Let [math]\lambda=(\lambda_1, \ldots, \lambda_r, 0, \ldots, 0)[/math] be the singular values of a matrix [math]A[/math]. We can define spectral norms on [math]A[/math] as vector norms on the vector [math]\lambda[/math]. In particular, for any [math]q\in [1, \infty][/math],
is called Schatten [math]q[/math]-norm of [math]A[/math]. Here again, special cases have special names:
- [math]q=2[/math]: [math]\|A\|_2=\|A\|_F[/math] is the Frobenius norm defined above.
- [math]q=1[/math]: [math]\|A\|_1=\|A\|_*[/math] is called the Nuclear norm (or trace norm) of [math]A[/math].
- [math]q=\infty[/math]: [math]\|A\|_\infty=\lambda_{\max{}}(A)=\|A\|_{\mathrm{op}}[/math] is called the operator norm (or spectral norm) of [math]A[/math].
We are going to employ these norms to assess the proximity to our matrix of interest. While the interpretation of vector norms is clear by extension from the vector case, the meaning of ‘`[math]\|A-B\|_{\mathrm{op}}[/math] is small" is not as transparent. The following subsection provides some inequalities (without proofs) that allow a better reading.
Useful matrix inequalities
Let [math]A[/math] and [math]B[/math] be two [math]m\times n[/math] matrices with singular values [math] \lambda_1(A) \ge \lambda _2(A) \ldots \ge \lambda_{\min(m,n)}(A)[/math] and [math] \lambda_1(B) \ge \ldots \ge \lambda_{\min(m,n)}(B)[/math] respectively. Then the following inequalities hold:
The singular value decomposition is associated to the following useful lemma, known as the ’'Eckart-Young (or Eckart-Young-Mirsky) Theorem. It states that for any given [math]k[/math] the closest matrix of rank [math]k[/math] to a given matrix [math]A[/math] in Frobenius norm is given by its truncated SVD.
Let [math]A[/math] be a rank-[math]r[/math] matrix with singular value decomposition
Note that the last equality of the lemma is obvious since
Multivariate regression
In the traditional regression setup, the response variable [math]Y[/math] is a scalar. In several applications, the goal is not to predict a variable but rather a vector [math]Y \in \R^T[/math], still from a covariate [math]X \in \R^d[/math]. A standard example arises in genomics data where [math]Y[/math] contains [math]T[/math] physical measurements of a patient and [math]X[/math] contains the expression levels for [math]d[/math] genes. As a result the regression function in this case [math]f(x)=\E[Y|X=x][/math] is a function from [math]\R^d[/math] to [math]\R^T[/math]. Clearly, [math]f[/math] can be estimated independently for each coordinate, using the tools that we have developed in the previous chapter. However, we will see that in several interesting scenarios, some structure is shared across coordinates and this information can be leveraged to yield better prediction bounds.
The model
Throughout this section, we consider the following multivariate linear regression model:
where [math]\Y \in \R^{n\times T}[/math] is the matrix of observed responses, [math]\X[/math] is the [math]n \times d[/math] observed design matrix (as before), [math]\Theta \in \R^{d\times T}[/math] is the matrix of unknown parameters and [math]E\sim \sg_{n\times T}(\sigma^2)[/math] is the noise matrix. In this chapter, we will focus on the prediction task, which consists in estimating [math]\X\Theta^*[/math]. As mentioned in the foreword of this chapter, we can view this problem as [math]T[/math] (univariate) linear regression problems [math]Y^{(j)}=\X\theta^{*,(j)}+ \eps^{(j)}, j=1, \ldots, T[/math], where [math]Y^{(j)}, \theta^{*,(j)}[/math] and [math]\eps^{(j)}[/math] are the [math]j[/math]th column of [math]\Y, \Theta^*[/math] and [math]E[/math] respectively. In particular, an estimator for [math]\X\Theta^*[/math] can be obtained by concatenating the estimators for each of the [math]T[/math] problems. This approach is the subject of Problem.
The columns of [math]\Theta^*[/math] correspond to [math]T[/math] different regression tasks. Consider the following example as a motivation. Assume that the \textsc{Subway} headquarters want to evaluate the effect of [math]d[/math] variables (promotions, day of the week, TV ads,\dots) on their sales. To that end, they ask each of their [math]T=40,000[/math] restaurants to report their sales numbers for the past [math]n=200[/math] days. As a result, franchise [math]j[/math] returns to headquarters a vector [math]\Y^{(j)} \in \R^n[/math]. The [math]d[/math] variables for each of the [math]n[/math] days are already known to headquarters and are stored in a matrix [math]\X \in \R^{n\times d}[/math]. In this case, it may be reasonable to assume that the same subset of variables has an impact of the sales for each of the franchises, though the magnitude of this impact may differ from franchise to franchise. As a result, one may assume that the matrix [math]\Theta^*[/math] has each of its [math]T[/math] columns that is row sparse and that they share the same sparsity pattern, i.e., [math]\Theta^*[/math] is of the form:
where [math]\bullet[/math] indicates a potentially nonzero entry. It follows from the result of Problem that if each task is performed individually, one may find an estimator [math]\hat \Theta[/math] such that
where [math]k[/math] is the number of nonzero coordinates in each column of [math]\Theta^*[/math]. We remember that the term [math]\log(ed)[/math] corresponds to the additional price to pay for not knowing where the nonzero components are. However, in this case, when the number of tasks grows, this should become easier. This fact was proved in[3]. We will see that we can recover a similar phenomenon when the number of tasks becomes large, though larger than in [3]. Indeed, rather than exploiting sparsity, observe that such a matrix [math]\Theta^*[/math] has rank [math]k[/math]. This is the kind of structure that we will be predominantly using in this chapter. Rather than assuming that the columns of [math]\Theta^*[/math] share the same sparsity pattern, it may be more appropriate to assume that the matrix [math]\Theta^*[/math] is low rank or approximately so. As a result, while the matrix may not be sparse at all, the fact that it is low rank still materializes the idea that some structure is shared across different tasks. In this more general setup, it is assumed that the columns of [math]\Theta^*[/math] live in a lower dimensional space. Going back to the \textsc{Subway} example this amounts to assuming that while there are 40,000 franchises, there are only a few canonical profiles for these franchises and that all franchises are linear combinations of these profiles.
Sub-Gaussian matrix model
Recall that under the assumption [math]\textsf{ORT}[/math] for the design matrix, i.e., [math]\X^\top \X=nI_d[/math], then the univariate regression model can be reduced to the sub-Gaussian sequence model. Here we investigate the effect of this assumption on the multivariate regression model\eqref{EQ:MVRmodel}. Observe that under assumption [math]\textsf{ORT}[/math],
Which can be written as an equation in [math]\R^{d\times T}[/math] called the sub-Gaussian matrix model (sGMM):
where [math]y=\frac{1}{n}\X^\top \Y[/math] and [math]F=\frac{1}{n}\X^\top E\sim \sg_{d\times T}(\sigma^2/n)[/math]. Indeed, for any [math]u \in \cS^{d-1}, v \in \cS^{T-1}[/math], it holds
where [math]w=\X u/\sqrt{n}[/math] has unit norm: [math]|w|_2^2=u^\top \frac{\X^\top \X}{n}u=|u|_2^2=1[/math]. Akin to the sub-Gaussian sequence model, we have a direct observation model where we observe the parameter of interest with additive noise. This enables us to use thresholding methods for estimating [math]\Theta^*[/math] when [math]|\Theta^*|_0[/math] is small. However, this also follows from Problem. The reduction to the vector case in the sGMM is just as straightforward. The interesting analysis begins when [math]\Theta^*[/math] is low-rank, which is equivalent to sparsity in its unknown eigenbasis. Consider the SVD of [math]\Theta^*[/math]:
and recall that [math]\|\Theta^*\|_0=|\lambda|_0[/math]. Therefore, if we knew [math]u_j[/math] and [math]v_j[/math], we could simply estimate the [math]\lambda_j[/math]s by hard thresholding. It turns out that estimating these eigenvectors by the eigenvectors of [math]y[/math] is sufficient. Consider the SVD of the observed matrix [math]y[/math]:
The singular value thresholding estimator with threshold [math]2\tau\ge 0[/math] is defined by
Recall that the threshold for the hard thresholding estimator was chosen to be the level of the noise with high probability. The singular value thresholding estimator obeys the same rule, except that the norm in which the magnitude of the noise is measured is adapted to the matrix case. Specifically, the following lemma will allow us to control the operator norm of the matrix [math]F[/math].
Let [math]A[/math] be a [math]d\times T[/math] random matrix such that [math]A \sim \sg_{d\times T}(\sigma^2)[/math]. Then
This proof follows the same steps as Problem. Let [math]\cN_1[/math] be a [math]1/4[/math]-net for [math]\cS^{d-1}[/math] and [math]\cN_2[/math] be a [math]1/4[/math]-net for [math]\cS^{T-1}[/math]. It follows from Lemma that we can always choose [math]|\cN_1|\le 12^d[/math] and [math]|\cN_2|\le 12^T[/math]. Moreover, for any [math]u \in \cS^{d-1}, v \in \cS^{T-1}[/math], it holds
The following theorem holds.
Consider the multivariate linear regression model\eqref{EQ:MVRmodel} under the assumption [math]\textsf{ORT}[/math] or, equivalently, the sub-Gaussian matrix model\eqref{EQ:sGMM}. Then, the singular value thresholding estimator [math]\Thetasvt[/math] with threshold
Assume without loss of generality that the singular values of [math]\Theta^*[/math] and [math]y[/math] are arranged in a non increasing order: [math]\lambda_1 \ge \lambda_2 \ge \dots[/math] and [math]\hat \lambda_1 \ge \hat \lambda_2\ge \dots[/math]. Define the set [math]S=\{j\,:\, |\hat \lambda_j| \gt 2 \tau\}[/math]. Observe first that it follows from Lemma that [math]\|F\|_{\mathrm{op}} \le \tau[/math] for [math]\tau[/math] chosen as in\eqref{EQ:threshSVT} on an event [math]\cA[/math] such that [math]\p(\cA)\ge 1-\delta[/math]. The rest of the proof assumes that the event [math]\cA[/math] occurred. Note that it follows from Weyl's inequality that [math]|\hat \lambda_j - \lambda_j|\le \|F\|_{\mathrm{op}}\le \tau[/math]. It implies that [math]S \subset \{j\,:\, |\lambda_j| \gt \tau\}[/math] and [math]S^c \subset \{j\,:\, |\lambda_j| \le 3 \tau\}[/math]. Next define the oracle [math]\bar \Theta=\sum_{j \in S} \lambda_j u_jv_j^\top[/math] and note that
In the next subsection, we extend our analysis to the case where [math]\X[/math] does not necessarily satisfy the assumption [math]\textsf{ORT}[/math].
Penalization by rank
The estimator from this section is the counterpart of the BIC estimator in the spectral domain. However, we will see that unlike BIC, it can be computed efficiently.
Let [math]\Thetarank[/math] be any solution to the following minimization problem:
This estimator is called estimator by rank penalization with regularization parameter [math]\tau^2[/math]. It enjoys the following property.
Consider the multivariate linear regression model\eqref{EQ:MVRmodel}. Then, the estimator by rank penalization [math]\Thetarank[/math] with regularization parameter [math]\tau^2[/math], where [math]\tau[/math] is defined in\eqref{EQ:threshSVT} satisfies
{{{4}}}
It follows from Theorem that the estimator by rank penalization enjoys the same properties as the singular value thresholding estimator even when [math]\X[/math] does not satisfy the [math]\textsf{ORT}[/math] condition. This is reminiscent of the BIC estimator which enjoys the same properties as the hard thresholding estimator. However this analogy does not extend to computational questions. Indeed, while the rank penalty, just like the sparsity penalty, is not convex, it turns out that [math]\X \Thetarank[/math] can be computed efficiently. Note first that
Therefore, it remains to show that
can be solved efficiently. To that end, let [math]\bar \Y=\X (\X^\top \X)^\dagger\X^\top\Y[/math] denote the orthogonal projection of [math]\Y[/math] onto the image space of [math]\X[/math]: this is a linear operator from [math]\R^{d \times T}[/math] into [math]\R^{n \times T}[/math]. By the Pythagorean theorem, we get for any [math]\Theta \in \R^{d\times T}[/math],
Next consider the SVD of [math]\bar \Y[/math]:
where [math]\lambda_1 \ge \lambda_2 \ge \ldots[/math] and define [math]\tilde \Y[/math] by
By Lemma, it holds
Therefore, any minimizer of [math]\X\Theta\mapsto \|\Y -\X \Theta\|_F^2[/math] over matrices of rank at most [math]k[/math] can be obtained by truncating the SVD of [math]\bar \Y[/math] at order [math]k[/math]. Once [math]\X\Thetarank[/math] has been found, one may obtain a corresponding [math]\Thetarank[/math] by least squares but this is not necessary for our results.
While the rank penalized estimator can be computed efficiently, it is worth pointing out that a convex relaxation for the rank penalty can also be used. The estimator by nuclear norm penalization [math]\hat \Theta[/math] is defined to be any solution to the minimization problem
Covariance matrix estimation
Empirical covariance matrix
Let [math]X_1, \ldots, X_n[/math] be [math]n[/math] i.i.d copies of a random vector [math]X \in \R^d[/math] such that [math]\E\big[X X^\top\big] =\Sigma[/math] for some unknown matrix [math]\Sigma \succ 0[/math] called covariance matrix. This matrix contains information about the moments of order 2 of the random vector [math]X[/math]. A natural candidate to estimate [math]\Sigma[/math] is the empirical covariance matrix [math]\hat \Sigma[/math] defined by
Using the tools of Chapter Sub-Gaussian Random Variables, we can prove the following result.
Let [math]Y \in \R^d[/math] be a random vector such that [math]\E[Y]=0, \E[YY^\top]=I_d[/math] and [math]Y \sim \sg_d(1)[/math]. Let [math]X_1, \ldots, X_n[/math] be [math]n[/math] independent copies of sub-Gaussian random vector [math]X =\Sigma^{1/2}Y[/math]. Then [math]\E[X]=0, \E[XX^\top]=\Sigma[/math] and [math]X \sim \sg_d(\|\Sigma\|_{\mathrm{op}})[/math]. Moreover,
{{{4}}}
Theorem indicates that for fixed [math]d[/math], the empirical covariance matrix is a consistent estimator of [math]\Sigma[/math] (in any norm as they are all equivalent in finite dimension). However, the bound that we got is not satisfactory in high-dimensions when [math]d \gg n[/math]. To overcome this limitation, we can introduce sparsity as we have done in the case of regression. The most obvious way to do so is to assume that few of the entries of [math]\Sigma[/math] are non zero and it turns out that in this case thresholding is optimal. There is a long line of work on this subject (see for example [5] and [6]). Once we have a good estimator of [math]\Sigma[/math], what can we do with it? The key insight is that [math]\Sigma[/math] contains information about the projection of the vector [math]X[/math] onto any direction [math]u \in \cS^{d-1}[/math]. Indeed, we have that [math]\var(X^\top u)=u^\top \Sigma u[/math], which can be readily estimated by [math]\widehat \Var(X^\top u)=u^\top \hat \Sigma u[/math]. Observe that it follows from Theorem that
with probability [math]1-\delta[/math]. The above fact is useful in the Markowitz theory of portfolio section for example [7], where a portfolio of assets is a vector [math]u \in \R^d[/math] such that [math]|u|_1=1[/math] and the risk of a portfolio is given by the variance [math] \Var(X^\top u)[/math]. The goal is then to maximize reward subject to risk constraints. In most instances, the empirical covariance matrix is plugged into the formula in place of [math]\Sigma[/math]. (See Problem).
Principal component analysis
Spiked covariance model
Estimating the variance in all directions is also useful for dimension reduction. In Principal Component Analysis (PCA), the goal is to find one (or more) directions onto which the data [math]X_1, \ldots, X_n[/math] can be projected without loosing much of its properties. There are several goals for doing this but perhaps the most prominent ones are data visualization (in few dimensions, one can plot and visualize the cloud of [math]n[/math] points) and clustering (clustering is a hard computational problem and it is therefore preferable to carry it out in lower dimensions). An example of the output of a principal component analysis is given in Figure. In this figure, the data has been projected onto two orthogonal directions [math]\textsf{PC1}[/math] and [math]\textsf{PC2}[/math], that were estimated to have the most variance (among all such orthogonal pairs). The idea is that when projected onto such directions, points will remain far apart and a clustering pattern will still emerge. This is the case in Figure where the original data is given by [math]d=500,000[/math] gene expression levels measured on [math]n \simeq 1,387[/math] people. Depicted are the projections of these [math]1,387[/math] points in two dimension. This image has become quite popular as it shows that gene expression levels can recover the structure induced by geographic clustering.
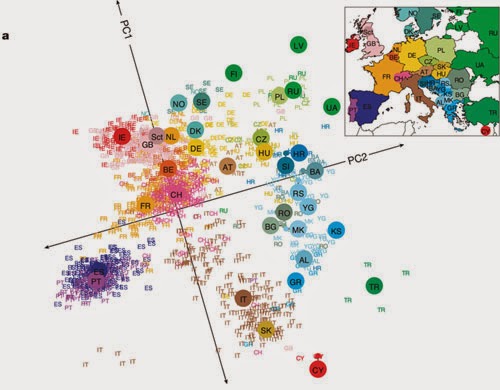
How is it possible to ‘`compress" half a million dimensions into only two? The answer is that the data is intrinsically low dimensional. In this case, a plausible assumption is that all the [math]1,387[/math] points live close to a two-dimensional linear subspace. To see how this assumption (in one dimension instead of two for simplicity) translates into the structure of the covariance matrix [math]\Sigma[/math], assume that [math]X_1, \ldots, X_n[/math] are Gaussian random variables generated as follows. Fix a direction [math]v \in \cS^{d-1}[/math] and let [math]Y_1, \ldots, Y_n \sim \cN_d(0,I_d)[/math] so that [math]v^\top Y_i[/math] are i.i.d. [math]\cN(0,1)[/math]. In particular, the vectors [math](v^\top Y_1)v, \ldots, (v^\top Y_n)v[/math] live in the one-dimensional space spanned by [math]v[/math]. If one would observe such data the problem would be easy as only two observations would suffice to recover [math]v[/math]. Instead, we observe [math]X_1, \ldots, X_n \in \R^d[/math] where [math]X_i=(v^\top Y_i)v+Z_i[/math], and [math]Z_i \sim \cN_d(0, \sigma^2I_d)[/math] are i.i.d and independent of the [math]Y_i[/math]s, that is we add isotropic noise to every point. If the [math]\sigma[/math] is small enough, we can hope to recover the direction [math]v[/math] (See Figure). The covariance matrix of [math]X_i[/math] generated as such is given by
This model is often called the ’'spiked covariance model. By a simple rescaling, it is equivalent to the following definition.
A covariance matrix [math]\Sigma \in \R^{d\times d}[/math] is said to satisfy the spiked covariance model if it is of the form
This model can be extended to more than one spike but this extension is beyond the scope of these notes.
Clearly, under the spiked covariance model, [math]v[/math] is the eigenvector of the matrix [math]\Sigma[/math] that is associated to its largest eigenvalue [math]1+\theta[/math]. We will refer to this vector simply as largest eigenvector. To estimate it, a natural candidate is the largest eigenvector [math]\hat v[/math] of [math]\tilde \Sigma[/math], where [math]\tilde \Sigma[/math] is any estimator of [math]\Sigma[/math]. There is a caveat: by symmetry, if [math]u[/math] is an eigenvector, of a symmetric matrix, then [math]-u[/math] is also an eigenvector associated to the same eigenvalue. Therefore, we may only estimate [math]v[/math] up to a sign flip. To overcome this limitation, it is often useful to describe proximity between two vectors [math]u[/math] and [math]v[/math] in terms of the principal angle between their linear span. Let us recall that for two unit vectors the principal angle between their linear spans is denoted by [math]\angle(u,v)[/math] and defined as
The following result from perturbation theory is known as the Davis-Kahan [math]\sin(\theta)[/math] theorem as it bounds the sin of the principal angle between eigenspaces. This theorem exists in much more general versions that extend beyond one-dimensional eigenspaces.
Let [math]A, B[/math] be two [math]d \times d[/math] PSD matrices. Let [math](\lambda_1, u_1), \ldots, (\lambda_d, u_d)[/math] (resp. [math](\mu_1, v_1), \ldots, (\mu_d, v_d)[/math]) denote the pairs of eigenvalues--eigenvectors of [math]A[/math] (resp. B) ordered such that [math]\lambda_1 \ge \lambda_2 \ge \dots[/math] (resp. [math]\mu_1 \ge \mu_2 \ge \dots[/math]). Then
Note that [math]u_1^\top A u_1=\lambda_1[/math] and for any [math]x \in \cS^{d-1}[/math], it holds
Combined with Theorem, we immediately get the following corollary.
Let [math]Y \in \R^d[/math] be a random vector such that [math]\E[Y]=0, \E[YY^\top]=I_d[/math] and [math]Y \sim \sg_d(1)[/math]. Let [math]X_1, \ldots, X_n[/math] be [math]n[/math] independent copies of sub-Gaussian random vector [math]X =\Sigma^{1/2}Y[/math] so that [math]\E[X]=0, \E[XX^\top]=\Sigma[/math] and [math]X \sim \sg_d(\|\Sigma\|_{\mathrm{op}})[/math]. Assume further that [math]\Sigma=\theta vv^\top + I_d[/math] satisfies the spiked covariance model. Then, the largest eigenvector [math]\hat v[/math] of the empirical covariance matrix [math]\hat \Sigma[/math] satisfies,
This result justifies the use of the empirical covariance matrix [math]\hat \Sigma[/math] as a replacement for the true covariance matrix [math]\Sigma[/math] when performing PCA in low dimensions, that is when [math]d \ll n[/math]. In the high-dimensional case, where [math]d \gg n[/math], the above result is uninformative. As before, we resort to sparsity to overcome this limitation.
Sparse PCA
In the example of Figure, it may be desirable to interpret the meaning of the two directions denoted by [math]\textsf{PC1}[/math] and [math]\textsf{PC2}[/math]. We know that they are linear combinations of the original 500,000 gene expression levels. A natural question to ask is whether only a subset of these genes could suffice to obtain similar results. Such a discovery could have potential interesting scientific applications as it would point to a few genes responsible for disparities between European populations. In the case of the spiked covariance model this amounts to having a sparse [math]v[/math]. Beyond interpretability as we just discussed, sparsity should also lead to statistical stability as in the case of sparse linear regression for example. To enforce sparsity, we will assume that [math]v[/math] in the spiked covariance model is [math]k[/math]-sparse: [math]|v|_0=k[/math]. Therefore, a natural candidate to estimate [math]v[/math] is given by [math]\hat v[/math] defined by
It is easy to check that [math]\lambda^k_{\max}(\hat \Sigma)=\hat v^\top \hat \Sigma \hat v[/math] is the largest of all leading eigenvalues among all [math]k\times k[/math] sub-matrices of [math]\hat \Sigma[/math] so that the maximum is indeed attained, though there my be several maximizers. We call [math]\lambda^k_{\max}(\hat \Sigma)[/math] the [math]k[/math]-sparse leading eigenvalue of [math]\hat \Sigma[/math] and [math]\hat v[/math] a [math]k[/math]-sparse leading eigenvector.
Let [math]Y \in \R^d[/math] be a random vector such that [math]\E[Y]=0, \E[YY^\top]=I_d[/math] and [math]Y \sim \sg_d(1)[/math]. Let [math]X_1, \ldots, X_n[/math] be [math]n[/math] independent copies of sub-Gaussian random vector [math]X =\Sigma^{1/2}Y[/math] so that [math]\E[X]=0, \E[XX^\top]=\Sigma[/math] and [math]X \sim \sg_d(\|\Sigma\|_{\mathrm{op}})[/math]. Assume further that [math]\Sigma=\theta vv^\top + I_d[/math] satisfies the spiked covariance model for [math]v[/math] such that [math]|v|_0=k \le d/2[/math]. Then, the [math]k[/math]-sparse largest eigenvector [math]\hat v[/math] of the empirical covariance matrix satisfies,
We begin by obtaining an intermediate result of the Davis-Kahan [math]\sin(\theta)[/math] theorem. Using the same steps as in the proof of Theorem, we get
Minimax lower bound
The last section has established that the spike [math]v[/math] in the spiked covariance model [math]\Sigma =\theta v v^\top[/math] may be estimated at the rate [math]\theta^{-1} \sqrt{k\log (d/k)/n}[/math], assuming that [math]\theta \le 1[/math] and [math]k\log (d/k)\le n[/math], which corresponds to the interesting regime. Using the tools from Chapter Minimax Lower Bounds, we can show that this rate is in fact optimal already in the Gaussian case.
Let [math]X_1, \ldots, X_n[/math] be [math]n[/math] independent copies of the Gaussian random vector [math]X \sim\cN_d(0, \Sigma)[/math] where [math]\Sigma=\theta vv^\top + I_d[/math] satisfies the spiked covariance model for [math]v[/math] such that [math]|v|_0=k[/math] and write [math]\p_v[/math] the distribution of [math]X[/math] under this assumption and by [math]\E_v[/math] the associated expectation. Then, there exists constants [math]C,c \gt 0[/math] such that for any [math]n\ge 2[/math], [math]d \ge 9[/math], [math]2 \le k \le (d+7)/8[/math], it holds
{{{4}}}
Together with the upper bound of Theorem, we get the following corollary.
Assume that [math]\theta \le 1[/math] and [math]k\log (d/k)\le n[/math]. Then [math]\theta^{-1} \sqrt{k\log (d/k)/n}[/math] is the minimax rate of estimation over [math]\cB_0(k)[/math] in the spiked covariance model.
Graphical models
Gaussian graphical models
In Section Covariance matrix estimation, we noted that thresholding is an appropriate way to gain an advantage from sparsity in the covariance matrix when trying to estimate it. However, in some cases, it is more natural to assume sparsity in the inverse of the covariance matrix, [math] \Theta = \Sigma^{-1} [/math], which is sometimes called concentration or precision matrix. In this chapter, we will develop an approach that allows us to get guarantees similar to the lasso for the error between the estimate and the ground truth matrix in Frobenius norm. We can understand why sparsity in [math] \Theta [/math] can be appropriate in the context of undirected graphical models [8]. These are models that serve to simplify dependence relations between high-dimensional distributions according to a graph.
Let [math] G = (V, E) [/math] be a graph on [math] d [/math] nodes and to each node [math] v [/math], associate a random variable [math] X_v [/math]. An undirected graphical model or Markov random field is a collection of probability distributions over [math] X_v [/math] that factorize according to
This definition implies certain conditional independence relations, such as the following.
A graphical model as in \eqref{eq:factor-graph} fulfills the global Markov property. That is, for any triple [math] (A, B, S) [/math] of disjoint subsets such that [math] S [/math] separates [math] A [/math] and [math] B [/math],
Let [math] (A, B, S) [/math] a triple as in the proposition statement and define [math] \tilde{A} [/math] to be the connected component in the induced subgraph [math] G[V \setminus S] [/math], as well as [math] \tilde{B} = G[V \setminus (\tilde{A} \cup S)] [/math]. By assumption, [math] A [/math] and [math] B [/math] have to be in different connected components of [math] G[V \setminus S] [/math], hence any clique in [math] G [/math] has to be a subset of [math] \tilde{A} \cup S [/math] or [math] \tilde{B} \cup S [/math]. Denoting the former cliques by [math] \mathcal{C}_A [/math], we get
A weaker form of the Markov property is the so-called pairwise Markov property, which says that the above holds only for singleton sets of the form [math] A = \{a\} [/math], [math] B = \{ b \} [/math] and [math] S = V \setminus \{a, b\} [/math] if [math] (a, b) \notin E [/math]. In the following, we will focus on Gaussian graphical models for [math] n [/math] i.i.d samples [math] X_i \sim \cN(0, (\Theta^\ast)^{-1}) [/math]. By contrast, there is a large body of work on discrete graphical models such as the Ising model (see for example [9]), which we are not going to discuss here, although links to the following can be established as well [10]. For a Gaussian model with mean zero, by considering the density in terms of the concentration matrix [math] \Theta [/math],
it is immediate that the pairs [math] a, b [/math] for which the pairwise Markov property holds are exactly those for which [math] \Theta_{i, j} = 0 [/math]. Conversely, it can be shown that for a family of distributions with non-negative density, this actually implies the factorization \eqref{eq:factor-graph} according to the graph given by the edges [math] \{ (i, j) : \Theta_{i, j} \neq 0 \} [/math]. This is the content of the Hammersley-Clifford theorem.
Let [math] P [/math] be a probability distribution with positive density [math] f [/math] with respect to a product measure over [math] V [/math]. Then [math] P [/math] factorizes over [math] G [/math] as in \eqref{eq:factor-graph} if and only if it fulfills the pairwise Markov property.
Show ProofDefine
It remains to show that [math] \Phi_A = 0 [/math] if [math] A [/math] is not a clique.
Assume [math] A \subseteq V [/math] with [math] v, w \in A [/math], [math] (v, w) \notin E [/math], and set [math] C = V \setminus \{v, w\} [/math].
By adding all possible subsets of [math] \{v, w\} [/math] to every subset of [math] C [/math], we get every subset of [math] A [/math], and hence
We show the if direction. The requirement that [math] f [/math] be positive allows us to take logarithms. Pick a fixed element [math] x^\ast [/math]. The idea of the proof is to rewrite the density in a way that allows us to make use of the pairwise Markov property. The following combinatorial lemma will be used for this purpose.
Let [math] \Psi, \Phi : \cP(V) \to \R [/math] be functions defined for all subsets of a set [math] V [/math]. Then, the following are equivalent:
- For all [math] A \subseteq V [/math]: [math] \Psi(A) = \sum_{B \subseteq A} \Phi(B) [/math];
- For all [math] A \subseteq V [/math]: [math] \Phi(A) = \sum_{B \subseteq A} (-1)^{|A \setminus B}\Psi(B) [/math].
[math] 1. \implies 2. [/math]:
In the following, we will show how to exploit this kind of sparsity when trying to estimate [math] \Theta [/math]. The key insight is that we can set up an optimization problem that has a similar error sensitivity as the lasso, but with respect to [math] \Theta [/math]. In fact, it is the penalized version of the maximum likelihood estimator for the Gaussian distribution. We set
where we wrote [math] \Theta_{D^c} [/math] for the off-diagonal restriction of [math] \Theta [/math], [math] \| A \|_1 = \sum_{i, j} | A_{i, j} | [/math] for the element-wise [math] \ell_1 [/math] norm, and [math] \hat{\Sigma} = \frac{1}{n} \sum_{i} X_i X_i^\top [/math] for the empirical covariance matrix of [math] n [/math] i.i.d observations. We will also make use of the notation
for the element-wise [math] \infty [/math]-norm of a matrix [math] A \in \R^{d \times d} [/math] and
for the [math] \infty [/math] operator norm. We note that the function [math] \Theta \mapsto - \log \operatorname{det}(\Theta) [/math] has first derivative [math] - \Theta^{-1} [/math] and second derivative [math] \Theta^{-1} \otimes \Theta^{-1} [/math], which means it is convex. Derivations for this can be found in [11](Appendix A.4). This means that in the population case and for [math] \lambda = 0 [/math], the minimizer in \eqref{eq:af} would coincide with [math] \Theta^\ast [/math]. First, let us derive a bound on the error in [math] \hat{\Sigma} [/math] that is similar to Theorem, with the difference that we are dealing with sub-Exponential distributions.
If [math] X_i \sim \cN(0, \Sigma) [/math] are i.i.d and [math] \hat{\Sigma} = \frac{1}{n} \sum_{i=1}^{n} X_i X_i^\top [/math] denotes the empirical covariance matrix, then
Start as in the proof of Theorem by writing [math] Y = \Sigma^{-1/2} X \sim \cN(0, I_d) [/math] and notice that
Let [math] X_i \sim \cN(0, \Sigma) [/math] be i.i.d and [math] \hat{\Sigma} = \frac{1}{n} \sum_{i=1}^{n} X_i X_i^\top [/math] denote the empirical covariance matrix. Assume that [math] | S | = k [/math], where [math] S = \{(i,j) : i \neq j, \, \Theta_{i, j} \neq 0 \} [/math]. There exists a constant [math] c = c(\| \Sigma \|_{\mathrm{op}}) [/math] such that if
We start by considering the likelihood
If we now write [math] \Delta = \hat{\Theta} - \Theta^\ast [/math] and assume [math] \| \Delta \|_F \geq 1 [/math], then by optimality of [math] \hat{\Theta} [/math],
Write [math] \Sigma^\ast = W^\ast \Gamma^\ast W^\ast [/math], where [math] W^2 = \Sigma^\ast_D [/math] and similarly define [math] \hat{W} [/math] by [math] \hat{W}^2 = \hat{\Sigma}_D [/math]. Define a slightly modified estimator by replacing [math] \hat{\Sigma} [/math] in \eqref{eq:af} by [math] \hat{\Gamma} [/math] to get an estimator [math] \hat{K} [/math] for [math] K = (\Gamma^\ast)^{-1} [/math].
Note that [math] \| A \|_{\infty} \leq \| A \|_{\mathrm{op}} [/math], so that if [math] n \gtrsim \log (d/\delta) [/math], then [math] \| \hat{\Gamma} - \Gamma \|_\infty \lesssim \sqrt{\log (d/\delta)}/\sqrt{n} [/math]. From the above arguments, conclude [math] \| \hat{K} - K^\ast \|_F \lesssim \sqrt{k \log(d/\delta)/n} [/math] and with a calculation similar to \eqref{eq:aa} that
This will not work in Frobenius norm since there, we only have [math] \| \hat{W}^2 - W^2 \|_F^2 \lesssim \sqrt{p \log(d/\delta)/n}[/math].
Lower bounds
Here, we will show that the bounds in Theorem are optimal up to log factors. It will again be based on applying Fano's inequality, Theorem. In order to calculate the KL divergence between two Gaussian distributions with densities [math] f_{\Sigma_1} [/math] and [math] f_{\Sigma_2} [/math], we first observe that
, so that
writing [math] \Delta = \Theta_2 - \Theta_1 [/math], [math] \tilde{\Theta} = \Theta_1 + t \Delta [/math], [math] t \in [0,1] [/math], Taylor expansion then yields
This means we are in good shape in applying our standard tricks if we can make sure that [math] \lambda_\mathrm{min}(\tilde{\Theta}) [/math] stays large enough among our hypotheses.
To this end, assume [math] k \leq p^2/16 [/math] and define the collection of matrices [math] (B_{j})_{j \in [M_1]} [/math] with entries in [math] \{0, 1\} [/math] such that they are symmetric, have zero diagonal and only have non-zero entries in a band of size [math] \sqrt{k} [/math] around the diagonal, and whose entries are given by a flattening of the vectors obtained by the sparse Varshamov-Gilbert Lemma.
Moreover, let [math] (C_j)_{j \in [M_2]} [/math] be diagonal matrices with entries in [math] \{0, 1\} [/math] given by the regular Varshamov-Gilbert Lemma Minimax Lower Bounds.
Then, define
We can ensure that [math] \lambda_\mathrm{min}(\tilde{\Theta}) [/math] is small by the Gershgorin circle theorem. For each row of [math] \tilde{\Theta} - I [/math],
if [math] n \gtrsim k/\log(1 + d/(2k)) [/math]. Hence,
and
which yields the following theorem.
Denote by [math] \mathcal{B}_k [/math] the set of positive definite matrices with at most [math] k [/math] non-zero off-diagonal entries and assume [math] n \gtrsim k \log(d) [/math]. Then, if [math] \p_{\Theta} [/math] denotes a Gaussian distribution with inverse covariance matrix [math] \Theta [/math] and mean [math] 0 [/math], there exists a constant [math] c \gt 0 [/math] such that
Ising model
Like the Gaussian graphical model, the Ising model is also a model of pairwise interactions but for random variables that take values in [math]\{-1,1\}^d[/math]. Before developing our general approach, we describe the main ideas in the simplest Markov random field: an Ising model without[Notes 1] external field[12]. Such models specify the distribution of a random vector [math]Z=(Z^{(1)}, \ldots, Z^{(d)})\in \dHyp[/math] as follows
where [math]W \in \R^{pd \times d}[/math] is an unknown matrix of interest with null diagonal elements and
is a normalization term known as log-partition function. Fix [math]\beta,\lambda \gt 0[/math]. Our goal in this section is to estimate the parameter [math]W[/math] subject to the constraint that the [math]j[/math]th row [math]e_j^\top W[/math] of [math]W[/math] satisfies [math]|e_j^\top W|_1\le \lambda[/math]. To that end, we observe [math]n[/math] independent copies [math]Z_1, \ldots Z_n[/math] of [math]Z\sim \p[/math]. To that end, estimate the matrix [math]W[/math] row by row using constrained likelihood maximization. Recall from [13](Eq.(4)) that for any [math]j \in [d][/math], it holds for any [math]z^{(j)}\in \{-1, 1\}[/math], that
where we used the fact that the diagonal elements [math]W[/math] are equal to zero. Therefore, the [math]j[/math]th row [math]e_j^\top W[/math] of [math]W[/math] may be estimated by performing a logistic regression of [math]Z^{(j)}[/math] onto [math]Z^{(\lnot j)}[/math] subject to the constraint that [math]|e_j^\top W|_1 \le \lambda[/math] and [math]\|e_j^\top W\|_\infty \le \beta[/math]. To simplify notation, fix [math]j[/math] and define [math]Y=Z^{(j)}, X=Z^{(\lnot j)}[/math] and [math]w^*=(e_j^\top W)^{(\lnot j)}[/math]. Let [math](X_1, Y_1), \ldots, (X_n, Y_n)[/math] be [math]n[/math] independent copies of [math](X,Y)[/math]. Then the (rescaled) log-likelihood of a candidate parameter [math]w \in \R^{d-1}[/math] is denoted by [math]\bar \ell_n(w)[/math] and defined by
With this representation, it is easy to see that [math]w \mapsto \bar \ell_n(w)[/math] is a concave function. The (constrained) maximum likelihood estimator (MLE) [math]\hat w \in \R^{d-1}[/math] is defined to be any solution of the following convex optimization problem:
This problem can be solved very efficiently using a variety of methods similar to the Lasso. The following lemma follows from[14].
Fix [math]\delta \in (0,1)[/math]. Conditionally on [math](X_1, \ldots, X_n)[/math], the constrained {MLE} [math]\hat w[/math] defined in\eqref{EQ:MLE-Ising} satisfies with probability at least [math]1-\delta[/math] that
We begin with some standard manipulations of the log-likelihood. Note that
Next, we get from the basic inequality [math]\bar \kappa_n(\hat w) \le \bar \kappa_n(w)[/math] for any [math]w \in \cB_1(\lambda)[/math] that
Note that the Hessian of [math]\kappa[/math] is given by
Next, we bound from below the quantity
To that end, we must exploit the covariance structure of the Ising model. The following lemma is similar to the combination of Lemmas6 and7 in[12].
Fix [math]R \gt 0[/math], [math]\delta\in (0,1)[/math] and let [math]Z \in \R^{d}[/math] be distributed according to\eqref{EQ:defIsing}. The following holds with probability [math]1-\delta/2[/math], uniformly in [math]u \in \cB_1(2\lambda)[/math]:
{{{4}}}
Combining the above two lemmas immediately yields the following theorem.
Let [math]\hat w[/math] be the constrained maximum likelihood estimator\eqref{EQ:MLE-Ising} and let [math]w^*=(e_j^\top W)^{(\lnot j)}[/math] be the [math]j[/math]th row of [math]W[/math] with the [math]j[/math]th entry removed. Then with probability [math]1-\delta[/math] we have
General references
Rigollet, Philippe; Hütter, Jan-Christian (2023). "High-Dimensional Statistics". arXiv:2310.19244 [math.ST].
Notes
References
- Bernstein, Dennis S. (2009). Matrix mathematics. 40. Princeton, NJ: Princeton University Press. pp. xlii+1139. ISBN 978-0-691-14039-1.
- Golub, Gene H.; Van Loan, Charles F. (1996). Matrix computations. 40. Baltimore, MD: Johns Hopkins University Press. pp. xxx+698. ISBN 0-8018-5414-8.
- 3.0 3.1 "Oracle inequalities and optimal inference under group sparsity" (2011). Ann. Statist. 39: 2164--2204. Institute of Mathematical Statistics. doi: .
- "Nuclear-norm penalization and optimal rates for noisy low-rank matrix completion" (2011). Ann. Statist. 39: 2302--2329. Springer. doi: .
- "Optimal rates of convergence for covariance matrix estimation" (2010). Ann. Statist. 38: 2118--2144. Birkh\"auser/Springer, New York. doi: .
- "Minimax estimation of large covariance matrices under $\ell_1$-norm" (2012). Statist. Sinica 22: 1319--1349. Springer-Verlag. doi: .
- Markowitz, Harry (1952). "Portfolio selection". The journal of finance 7: 77--91. Wiley Online Library. doi: .
- Lauritzen, Steffen L. (1996). Graphical models. 17. Boca Raton (Fla.): The Clarendon Press, Oxford University Press, New York. pp. x+298. ISBN 0-19-852219-3.
- Bresler, Guy (2015). "Efficiently learning Ising models on arbitrary graphs". STOC'15---Proceedings of the 2015 ACM Symposium on Theory of Computing 5171: 771--782. Springer, Berlin. doi: .
- Loh, Po-Ling; Wainwright, Martin J.; others (2012). Structure Estimation for Discrete Graphical Models: Generalized Covariance Matrices and Their Inverses. NIPS (Report). 38. Wiley. pp. 2096--2104.
- Boyd, Stephen; Vandenberghe, Lieven (2004). Convex optimization. 36. Cambridge: Cambridge University Press. pp. xiv+716. ISBN 0-521-83378-7.
- 12.0 12.1 "Minimax Rates in Permutation Estimation for Feature Matching" (2016). Journal of Machine Learning Research 17: 1-31. Walter de Gruyter & Co., Berlin. doi: .
- "High-dimensional Ising model selection using $\ell_1$-regularized logistic regression" (2010). Ann. Statist. 38: 1287--1319. Springer. doi: .
- Rigollet, Philippe (2012). "Kullback-Leibler aggregation and misspecified generalized linear models". Ann. Statist. 40: 639--665. Springer-Verlag. doi: .